Remedies for Robots
What happens when artificially intelligent robots misbehave? The question is not just hypothetical. As robotics and artificial intelligence systems increasingly integrate into our society, they will do bad things. We seek to explore what remedies the law can and should provide once a robot has caused harm.
Remedies are sometimes designed to make plaintiffs whole by restoring them to the condition they would have been in “but for” the wrong. But they can also contain elements of moral judgment, punishment, and deterrence. In other instances, the law may order defendants to do (or stop doing) something unlawful or harmful.
Each of these goals of remedies law, however, runs into difficulties when the bad actor in question is neither a person nor a corporation but a robot. We might order a robot—or, more realistically, the designer or owner of the robot—to pay for the damages it causes. But it turns out to be much harder for a judge to “order” a robot, rather than a human, to engage in or refrain from certain conduct. Robots can’t directly obey court orders not written in computer code. And bridging the translation gap between natural language and code is often harder than we might expect. This is particularly true of modern artificial intelligence techniques that empower machines to learn and modify their decision-making over time. If we don’t know how the robot “thinks,” we won’t know how to tell it to behave in a way likely to cause it to do what we actually want it to do.
Moreover, if the ultimate goal of a legal remedy is to encourage good behavior or discourage bad behavior, punishing owners or designers for the behavior of their robots may not always make sense—if only for the simple reason that their owners didn’t act wrongfully in any meaningful way. The same problem affects injunctive relief. Courts are used to ordering people and companies to do (or stop doing) certain things, with a penalty of contempt of court for noncompliance. But ordering a robot to abstain from certain behavior won’t be trivial in many cases. And ordering it to take affirmative acts may prove even more problematic.
In this Article, we begin to think about how we might design a system of remedies for robots. Robots will require us to rethink many of our current doctrines. They also offer important insights into the law of remedies we already apply to people and corporations.
Introduction
Engineers training an artificially intelligent self-flying drone were perplexed.1 They were trying to get the drone to stay within a predefined circle and to head toward its center. Things were going well for a while. The drone received positive reinforcement for its successful flights, and it was improving its ability to navigate toward the middle quickly and accurately. Then, suddenly, things changed. When the drone neared the edge of the circle, it would inexplicably turn away from the center, leaving the circle.
What went wrong? After a long time spent puzzling over the problem, the designers realized that whenever the drone left the circle during tests, they had turned it off. Someone would then pick it up and carry it back into the circle to start again. From this pattern, the drone’s algorithm had learned—correctly—that when it was sufficiently far from the center, the optimal way to get back to the middle was to simply leave it altogether. As far as the drone was concerned, it had discovered a wormhole. Somehow, flying outside of the circle could be relied upon to magically teleport it closer to the center. And far from violating the rules instilled in it by its engineers, the drone had actually followed them to a T. In doing so, however, it had discovered an unforeseen shortcut—one that subverted its designers’ true intent.
What happens when artificially intelligent robots don’t do what we expect, as the drone did here? The question is not just hypothetical. As robotics and artificial intelligence (AI) systems increasingly integrate into our society, they will do bad things. Sometimes they will cause harm because of a design or implementation defect: we should have programmed the self-driving car to recognize a graffiti-covered stop sign but failed to do so. Sometimes they will cause harm because it is an unavoidable by-product of the intended operation of the machine. Cars, for example, kill thousands of people every year, sometimes unavoidably. Self-driving cars will too. Sometimes the accident will be caused by an internal logic all of its own—one that we can understand but that still doesn’t sit well with us. Sometimes robots will do the things we ask them to (minimize recidivism, for instance) but in ways we don’t like (such as racial profiling). And sometimes, as with our drone, robots will do unexpected things for reasons that doubtless have their own logic, but which we either can’t understand or predict.
These new technologies present a number of interesting questions of substantive law, from predictability, to transparency, to liability for high-stakes decision-making in complex computational systems. A growing body of scholarship is beginning to address these types of questions.2 Our focus here is different. We seek to explore what remedies the law can and should provide once a robot has caused harm.
The law of remedies is transsubstantive. Whereas substantive law defines who wins legal disputes, remedies law asks, “What do I get when I win?” Remedies are sometimes designed to make plaintiffs whole by restoring them to the condition they would have been in “but for” the wrong. But they can also contain elements of moral judgment, punishment, and deterrence. For instance, the law will often act to deprive a defendant of its gains, even if the result is a windfall to the plaintiff, because we think it is unfair to let defendants keep those gains. In other instances, the law may order defendants to do (or stop doing) something unlawful or harmful.
Each of these goals of remedies law, however, runs into difficulties when the bad actor in question is neither a person nor a corporation but a robot. We might order a robot—or, more realistically, the designer or owner of the robot—to pay for the damages it causes. (Though, as we will see, even that presents some surprisingly thorny problems.) But it turns out to be much harder for a judge to “order” a robot, rather than a human, to engage in or refrain from certain conduct. Robots can’t directly obey court orders not written in computer code. And bridging the translation gap between natural language and code is often harder than we might expect. This is particularly true of modern AI techniques that empower machines to learn and modify their decision-making over time,3 as the drone in the opening example did. If we don’t know how the robot “thinks,” we won’t know how to tell it to behave in a way likely to cause it to do what we actually want it to do.
One way to avoid these problems may be to move responsibility up the chain of command from a robot to its human or corporate masters—either the designers of the system or the owners who deploy it. But that too is easier said than done. Robot decision-making is increasingly likely to be based on algorithms of staggering complexity and obscurity. The developers—and certainly the users—of those algorithms won’t necessarily be able to deterministically control the outputs of their robots. To complicate matters further, some systems—including many self-driving cars—distribute responsibility for their robots between both designers and downstream operators. For systems of this kind, it has already proven extremely difficult to allocate responsibility when accidents inevitably occur.4
Moreover, if the ultimate goal of a legal remedy is to encourage good behavior or discourage bad behavior, punishing owners or designers for the behavior of their robots may not always make sense—if only for the simple reason that their owners didn’t act tortiously. The same problem affects injunctive relief. Courts are used to ordering people and companies to do (or stop doing) certain things, with a penalty of contempt of court for noncompliance. But ordering a robot to abstain from certain behavior won’t be trivial in many cases. And ordering it to take affirmative acts may prove even more problematic.
In this Article, we begin to think about how we might design a system of remedies for robots. It may, for example, make sense to focus less of our doctrinal attention on moral guilt and more of it on no-fault liability systems (or at least ones that define fault differently) to compensate plaintiffs. But addressing payments for injury solves only part of the problem. Often, we want to compel defendants to do (or not do) something in order to prevent injury. Injunctions, punitive damages, and even remedies like disgorgement are all aimed—directly or indirectly—at modifying or deterring behavior. But deterring robot misbehavior is going to look very different than deterring humans. Our existing doctrines often take advantage of “irrational” human behavior like cognitive biases and risk aversion. Courts, for instance, can rely on the fact that most of us don’t want to go to jail, so we tend to avoid conduct that might lead to that result. But robots will be deterred only to the extent that their algorithms are modified to include sanctions as part of the risk-reward calculus. These limitations may even require us to institute a “robot death penalty” as a sort of specific deterrence against certain bad behaviors. Today, speculation of this sort may sound far-fetched. But the field already includes examples of misbehaving robots being taken offline permanently5 —a trend which only appears likely to increase in the years ahead.
Finally, remedies law also has an expressive component that will be complicated by robots. We sometimes grant punitive damages—or disgorge ill-gotten gains—to show our displeasure with you. If our goal is just to feel better about ourselves, perhaps we might also punish robots simply for the sake of punishing them. Professor Christina Mulligan half-jokingly suggests that we should have the right to punch a robot.6 But if our goal is to send a slightly more nuanced signal than that through the threat of punishment, robots will require us to rethink many of our current doctrines.
In Part I, we discuss the development of robots and learning AIs, as well as the sorts of robot wrongdoing that will increasingly draw the attention of the legal system. In Part II, we outline the basic principles of remedies law and consider how those remedies will work—or not work—when applied to robots and AIs. Finally, in Part III, we consider how we might remake remedies law with robots in mind.
I. Bad Robots
A. Rise of the Machines
“Robots again.” When Judge Alex Kozinski opened his dissent from denial of rehearing en banc in Wendt v Host International7 with this line, he could count on it fetching an ironic grin because it was, well, ironic.8 Wendt prominently featured an animatronic version of two television personas,9 much like another case the jurist had overseen some three years prior.10 And in the late 1990s, suits of this sci-fi-esque variety represented such a novelty that the judge’s reference was unmissable. Robots again? Sure. But only because two cases in three years involving robots felt, at the time, like a freak recurrence.
Fast forward just two decades to the present, and Judge Kozinski’s quip appears quaint by comparison. Nowadays, robots are ubiquitous. Industries as far flung as finance, transportation, defense, and healthcare regularly invest billions in the technology. Patent filings for robotics and AI applications have surged.11 Even octogenarian senators can be heard fumbling over phrases once confined exclusively to computer science departments, such as “botnet,” “machine learning algorithm,” and “deep neural network.”12 Robots again, indeed.
Comparing these two moments—separated by just twenty years—puts on full display the field’s breathtaking progress. Today, technological feats that read like pages torn from sci-fi novels have become regular fixtures of the news. Robots have driven millions of miles on US roadways,13 humbled human professionals at the pinnacle of their fields,14 and even performed high-stakes surgical procedures on cardiac patients.15 And as innovators continue to compete against each other in increasingly diverse domains, “robots” themselves are taking on new and expansive forms.16 Gone are the days of robots confined to assembly lines or warehouse floors.17 With each passing week, robots infiltrate deeper into our public spaces, places of work, and even bedrooms.18
The disruptive forces unleashed by this ascendant technology are challenging long-held assumptions about the limits of machine capabilities—forcing the rest of society to adapt not only economically and politically, but also legally. In the last few years alone, autonomous19 robots have killed and maimed others, accidentally20 or intentionally;21 helped determine who goes to prison and who stays there;22 spouted racist and homophobic remarks on our social media platforms;23 and even shaped the course of our national elections.24 Far from anomalous, all signs suggest that these types of events are destined to become the new normal as robots continue their march into the social mainstream in the decades ahead.
In the view of many leading experts, the challenges posed by this impending “robot revolution”25 could precipitate a jurisprudential revolution of similar magnitude.26 And though numerous scholars have begun to explore the ramifications robots pose for our substantive legal rules, comparatively little attention has been paid to the rules governing remedies.27 Our goal is to change that. But in order to understand the impact that robots may have on this area of law, it is helpful to first review the technology’s defining characteristics, as well as the ways legal issues will most likely arise.
B. Defining “Robot”
Though “robot” has appeared in common parlance for nearly a century,28 the term is still notoriously resistant to definition.29 For many outside of computer science circles, it continues to evoke 1950s-era stock images of ironclad humanoids adorned with flashing lights, accompanied by the obligatory monotone voice. More recently, though, “robot” and its derivative “robotics” have come to take on more exacting definitions within broader expert communities.
Among legal scholars, efforts have been made to define robots by their so-called “essential qualities.”30 Such qualities refer to the fundamental, legally pertinent “characteristics that distinguish [robots] from prior or constituent technology such as computers or phones.”31 One leading scholar, Professor Ryan Calo, argues that robots exhibit at least three “essential qualities”: namely, “embodiment,”32 “emergence,”33 and “social valence.”34 In Calo’s telling:
Robotics combines, arguably for the first time, the promiscuity of information with the [embodied] capacity to do physical harm. Robots display increasingly emergent behavior, permitting the technology to accomplish both useful and unfortunate tasks in unexpected ways. And robots, more so than any technology in history, feel to us like social actors—a tendency so strong that soldiers sometimes jeopardize themselves to preserve the “lives” of military robots in the field.35
In light of these qualities, Calo argues that “robots are best thought of as artificial objects or systems that sense, process, and act upon the world to at least some degree.”36 Thus, “[a] robot in the strongest, fullest sense of the term exists in the world as a corporeal object with the capacity to exert itself physically.”37
As innovation in robotics continues to advance apace, however, the sharp dividing lines of even these recently established “essential qualities” are rapidly blurring. Nowadays, disembodied systems that exist purely as bits and bytes regularly go by the monikers of “bot,” “chatbot,” “crawlerbot,” “spambot,” “socialbot,” and so forth. When systems of these types operate in parallel, the collective is often referred to by the ominous title of “botnet.” And when gaming or strategy robots run metaphorical circles around human champions in the likes of Go38 or DotA,39 they do so in entirely ethereal forms with the capacity to exert themselves only digitally.
Thus, unlike some technologies that have become routinized as their commercial and social presence has increased, robots appear to have done the opposite. As Professor Jack Balkin recently observed, a similar phenomenon occurred in the cell phone industry.40 According to the scholar, “Thirty years ago people might have argued that an essential characteristic of a cell phone was its ability to make a phone call outside of one’s home. But this feature of cell phones is by no means the primary way that people use them today.”41 So, too, it seems is true of the “essential qualities” of yesteryears’ robots. Already, those that Calo enumerated less than five years ago read like relics of a bygone era—a testament to the field’s engine of innovation firing on all cylinders.42
Today, the terms “robotics” and “artificial intelligence” are often used interchangeably, referring to both embodied and disembodied systems that affect the physical and digital worlds alike. And while there are important technical distinctions to be made between the two concepts, we adopt the convention of construing “robot” to encompass both robots in Calo’s “essentialist” sense and artificially intelligent systems embodied only in software. Our goal is to include any hardware or software system exhibiting intelligent behavior.43
1. What makes robots smart?
But what, then, does it mean for a robot to be “intelligent”? Experts operating at the cutting edge of the field describe “artificial intelligence”—in somewhat circular fashion—as the “science of making machines smart.”44 And though the definition may be wanting for precision, it is this singular feature—the ability to execute complex behaviors such as planning, language processing, or object recognition—that differentiates a robot from a barren hunk of metal, plastic, or bits.45
Robots exhibit their “smarts” by executing “algorithms.”46 Although the term has a certain cerebral ring to it, it actually describes a simple concept. Algorithms are merely sequences of instructions for performing a given task.47 When translated into software, these instructions can be simplified further still. In fact, all commands given to a computational system are reducible to one of three logical operators: AND, OR, and NOT.48 If chained together in the right way, these basic operators can produce behaviors of breathtaking complexity. Yet at bottom, even the most sophisticated algorithms are composed of simple, logic-based building blocks.
For much of AI’s history as a scientific field, the prevailing paradigm of system design involved explicitly encoding the algorithms that governed robots.49 This approach—sometimes termed the “classic,” “symbolic,” or “GOFAI” approach (short for “Good Old-Fashioned A.I.”)—required that scientists or engineers hand-code robot behaviors through “explicit, logical representation of facts about the world.”50 The expression “dogs have four legs,” for example, might be represented as:51
∀x (is_a_dog(x) ⇒ number_of_legs(x) = 4)
In plain English, this statement translates to: “For every entity, if that entity is a dog, it has four legs.”
The precision and austerity of the GOFAI approach has obvious appeal. Among other features, explicitly encoded algorithms are inherently predictable and explainable. And robots programmed using this approach are still capable of exhibiting astonishingly complex behaviors, ranging from mathematical calculations far surpassing human capabilities, to conquering world chess champions.52
But GOFAI also has its limits. How, for example, is an AI system embedded with a four-legged representation of dogs supposed to categorize the small fraction that do not have four legs, either through accident or genetics? Without prospectively accounting for these types of outliers, hand-coded machines have no means of learning such distinctions on the fly.53
Take, for example, the task of navigation. Classically encoded robots have long excelled at getting from point A to point B in warehouses or factories—whether traversing a floor on four wheels or a three-dimensional space with an articulated arm.54 This aptitude has owed to the fact that warehouses and factories are, by and large, tightly controlled environments. As such, “programmers could anticipate the range of scenarios a [robot] may encounter, and could program if-then-else-type decision algorithms accordingly.”55
On a smooth, clearly demarcated surface with little chance of encountering obstacles (much less inclement weather) the number of uncertainties and edge cases presented was reduced to manageable proportions. But translating a similar navigation task to a bustling city street has been another matter entirely. Because the number of uncertainties a robot might encounter in most uncontrolled environments approaches infinity, navigating using a GOFAI approach requires a commensurate number of a priori if-then-else statements. Hand-coded algorithms, in other words, simply do not scale. For decades, this inherent limitation of GOFAI—what Professor Pedro Domingos terms the “knowledge acquisition bottleneck”56 —hindered significant progress in the field, leading to a painful period of stagnation that came to be known as the “AI Winter.”57 Thanks to recent breakthroughs in an innovative approach known as “machine learning,” however, the AI Winter is emphatically over.58
2. How do machines learn?
Machine learning turns the GOFAI approach to algorithmic design on its head. Rather than laying out a specific set of instructions for the robot follow, engineers instead specify a goal or set of goals for the robot to achieve when tackling a given problem, often referred to as an “optimizing function.” Having established the desired goal, the robot is then left to author its own algorithms for achieving it, which it does by practicing on illustrative examples of the problem at hand.
At the outset, the robot usually just flails around in the dark—trying things essentially at random without a good idea of what will or won’t work.59 But each time its experimental efforts move it closer to the goal specified by its designers, the robot receives positive feedback and uses statistical techniques to improve its algorithms accordingly.60 Thus, instead of repeatedly executing an unchanging set of instructions, machine learning approaches enable robots to iteratively write their own instructions as they go.61 And if given enough examples to train on, these systems can prove remarkably adept at solving staggeringly complex tasks that admit of no obvious GOFAI solutions.
Therein lies the promise of machine learning. When the endless fine-tuning of algorithmic instructions would be impossible to do by hand, machines themselves are able to successfully navigate the knowledge acquisition bottleneck.62 The program, thus, becomes the programmer—obviating the need for engineers to anticipate a near-infinite number of edge cases.
When embedded in a broader software or hardware application, the possibilities created by this powerful approach are seemingly endless. Indeed, many leading experts now view machine learning as one among a rarified number of “general-purpose technologies” (GPTs), the likes of which include the modern engine, the Internet, and electricity.63 Such technologies are distinguished by their ability to “significantly enhance productivity or quality across a wide number of fields or sectors.”64 Scholars have recognized three criteria of GPTs that machine learning appears to possess in abundance: “[T]hey have pervasive application across many sectors; they spawn further innovation in application sectors, and they themselves are rapidly improving.”65
Today, companies as diverse as Walmart, Facebook, and General Motors are adopting machine learning systems at “unprecedented rates due to the technology’s ability to radically improve data-driven decision-making at a cost and scale incomparable to that of humans.”66 It is this engineering approach that allows autonomous vehicles, self-flying drones, and warehouse “fetching” robots67 to function with seeming ease in unimaginably complex environments. And beyond these robots of the more “essentialist” variety, machine learning also powers a vast array of entities classified as “cyber-physical systems” (for example, Internet of Things devices), as well as disembodied digital systems often classified as software bots.68
C. When Robots Do Harm
Machine learning is not without its limitations, however. By breaking from the GOFAI paradigm, robots powered by this technique must also embrace a higher degree of uncertainty than their classically encoded counterparts. Because machines share in the task of writing their algorithms, using machine learning requires sacrificing some degree of fine-grained control over a machine’s algorithms. Accordingly, designers seeking to implement this powerful approach also understand that it can produce robots that are difficult to predict, tricky to debug, and hard or even impossible to understand.69
For many years, this engineering reality limited the most successful machine learning applications to domains with high degrees of fault tolerance. After all, it is one thing for a song recommendation engine to miss its mark 20 percent of the time. But it is quite another for an autonomous vehicle’s Light Detection and Ranging (LIDAR) system to miss oncoming vehicles at a similar clip.
In the last decade, however, advances in the field have enabled engineers to dramatically improve the accuracy, predictability, and performance of numerous machine learning applications—thus enabling them to entrust robots with positions of greater decision-making authority than ever before. It is these advances that have allowed for the introduction of high-stakes robotics systems including self-driving cars,70 medical diagnostic robots,71 and even experimental autonomous passenger drones.72 Yet, even the most performant of these systems remains imperfect—much like the human decision-makers they seek to emulate.
Accepting imperfection also means accepting the possibility that robotics systems will sometimes cause harm to others. Indeed, robots acting in harmful, occasionally catastrophic, ways are already a regular fixture of modern life.73 The following sections survey some of the harms complex robotics systems are likeliest to cause, providing contemporary examples of each.
1. Unavoidable harms.
Many robots operating free from software bugs, hardware errors, or failures of engineering precaution will nevertheless harm others. Some dangers, after all, are inherent to a product or service. In such instances, calling for the total elimination of the danger is tantamount to calling for a prohibition on a product or service itself.
Harms of this variety are often referred to as “unavoidable harms.”74 Conceptually, the notion of such harms tends to evoke products such as cigarettes, pharmaceuticals, alcohol, or knives. But as Professor Robert Peterson notes, virtually no product or service is perfectly “safe,” whether it is a jar of peanuts75 or a tea cozy—much less a complex robotics application.76 Robots have injured people by breaking things in warehouses.77 But so, of course, have people.
An illustrative example of the types of unavoidable harms that robots will cause can be found in the autonomous vehicle context. Ever since the transition from the horse-drawn buggy to the modern automobile, vehicular transportation has entailed error-prone humans, strapped to hulking masses of steel, navigating highly complex environments at highly dangerous speeds. Accordingly, “For more than a century, safety professionals have begun with the assumption that cars would crash, and focused their efforts on reducing the damage.”78 Experts too numerous to list have convincingly argued that this same assumption will also hold for cars driven by robots as opposed to humans.79 For even superhumanly safe self-driving systems are subject to the laws of physics. And if autonomous vehicles driven by such systems unexpectedly encounter an individual or object without sufficient time or distance to prevent a collision, harm of some variety may be unavoidable.80
2. Deliberate least-cost harms.
A close relative of the “unavoidable harms” detailed above involves “deliberate least-cost harms.” These harms are similar to unavoidable ones insofar as they are foreseeable by designers and, in some sense, cannot be avoided. But unlike their entirely unavoidable counterparts, deliberate least-cost harms fall into a grey area where there is sufficient forewarning to meaningfully react to an impending harmful event, but no way to avoid the harm entirely. The question, thus, becomes one of triage: Which of the harmful outcomes is the least costly?81
This type of lesser-of-evils dilemma, in which injury is both inevitable and variable, was canonized by the philosopher Judith Thomson in a thought experiment known as the “trolley problem.”82 In its most popular formulation, the trolley problem proceeds as follows:
[A]n observer [ ] is witness to a runaway trolley car barreling toward five unwitting workers on the tracks ahead. The observer, however, is standing at a switch. If pulled, it will divert the trolley onto another track where only one unlucky worker awaits. Tragedy of some kind is foreordained, but the observer holds the proverbial power to steer fate: turn the trolley, killing the one, or refrain from turning the trolley, killing the five?83
Ever since the introduction of experimental autonomous vehicles to US roadways, scenarios involving killer robocars thrust into trolley problem-like dilemmas have captured the public and academic imagination.84 But situations of this kind will likely be the exception, not the rule, when it comes to deliberate least-cost harms.85 Far likelier, albeit subtler, scenarios involving least-cost harms will involve robots that make decisions with seemingly trivial implications at an individual level, but which result in nontrivial impacts at scale.86
Self-driving cars, for example, will rarely face a stark choice between killing a child or killing two elderly people. But thousands of times a day, they will have to choose precisely where to change lanes, how closely to trail another vehicle, when to accelerate on a freeway on-ramp, and so forth. Each of these decisions will entail some probability of injuring someone. And making the “right” decision will require weighing the probability of causing harm, exploring what alternatives exist, and specifying how the car should value the different types of harms that will foreseeably impact different stakeholders. Even seemingly trivial design decisions of this kind will possibly carry a profound ethical and legal weight—requiring designers to grapple with complex, highly fraught tradeoffs inherent to deliberate least-cost harms, such as how much room a self-driving car should give cyclists on one side versus cars on the other.
3. Defect-driven harms.
One of the more obvious ways robots will cause harm is through traditional hardware or software “defects.”87 Harms of this variety occur when a software bug, hardware failure, or insufficient level of precaution by designers causes a robot to injure others. For much of the field’s history, these types of defect-driven harms have been relatively easy to define and identify. They typically occur when designers intend a robot to work in a certain way but make a mistake, causing it to behave differently, as was recently alleged in a case involving a robot that “escaped” from its section of a trailer hitch assembly plant, “entered [a technician’s] work area, surpris[ed] her, and crushed her head between hitch assemblies.”88
As robots continue to take on increasingly sophisticated forms, however, defining and identifying these types of “defects” will likely become more challenging. Is a self-driving car to be deemed “defective” if it brakes more slowly than a human driver? What if it brakes faster than humans, but not as fast as other self-driving cars? Or as fast as other self-driving cars, but not as fast as it might possibly brake if reprogrammed?
Additional legal wrinkles involving defect-driven harms will also arise in systems involving a “human-in-the-loop,”89 in which responsibility for controlling a robot is distributed between algorithmic and human decision-makers. A boundary-pushing example of this phenomenon recently occurred in Tempe, Arizona, when a self-driving car deployed by Uber fatally struck a pedestrian.90 Although the vehicle was capable of autonomy under certain design parameters, it also relied on a backup driver to take control in the event of an emergency.91 Yet one night, when a pedestrian unexpectedly walked out in front of one such vehicle, neither the backup driver nor the self-driving system took steps to avoid the collision.92 As a result, the vehicle collided with the pedestrian at speeds in excess of thirty miles per hour without braking or swerving.93 Should the backup driver be held responsible for failing to take over? Or was it unreasonable for Uber to put the operator in such a position to begin with? Does it matter how the car was programmed?
How the legal system will eventually resolve controversies involving these types of “moral crumple zones”94 remains an open question, even among experts.95 But none question the reality that robots exhibiting increasingly complex design defects will continue to harm individuals for the foreseeable future.
4. Misuse harms.
Sometimes, people will misuse robots in a manner that is neither negligent nor criminal but nevertheless threatens to harm others. Given the unpredictable nature of machine learning systems, and the nearly infinite variety of ways humans can interact with modern robotics applications, these types of harms are particularly difficult to prevent. Already, media reports are rife with examples of individuals attempting to manipulate robot behaviors, deceive or “trick” robot perception systems, probe robots for safety or security vulnerabilities, or deploy robots in ways that adversely impact others.96 Whether such forms of meddling are deemed to have been preventable by manufacturers, or to have fallen within the scope of the robot’s intended design, will have significant implications for the substantive legal doctrines that will govern the ultimate outcomes and for who bears the resulting liability.
A now infamous example of robot misuse comes from Microsoft’s Twitter chatbot, “Tay.” Unlike chatbots designed to maintain a static internal state upon deployment, Tay’s system updated itself in real time by learning from interactions with users.97 Within hours of going live, however, hundreds of Twitter users began intentionally tweeting “misogynistic, racist, and Donald Trumpist remarks”98 at the robot.99 Thanks to this barrage of unforeseen misuse, “Tay rapidly morphed from a fun-loving bot . . . into an AI monster.”100 Tay lasted a mere sixteen hours on the platform before Microsoft intervened. After initially declining to comment, the company eventually noted that a “coordinated effort by some [Twitter] users to abuse Tay’s commenting skills” led it to shut the robot down.101
One notable feature of the Tay example is that Microsoft itself did not engage in misuse. Nor is there any reason to think that Tay’s design was defective. Rather, the robot’s rogue conduct resulted from the input of third parties.102 But owners, too, will misuse robots, or at least use them in ways we may not expect. Drone owners, for example, might use them to spy on neighbors or invade their privacy.103 Similarly, self-driving car owners might modify their vehicles to protect occupants at all costs, even if doing so imposes greater risks on bystanders. And predictive learning algorithms that might decide everything from the cost of your life insurance to where you end up in an emergency room queue to whether you are granted parole are all dependent on the training data they are fed. And that training is only as good as the (often imperfect) data users feed the robot.104
5. Unforeseen harms.
Many harms attributable to robots will be neither defect-driven, unavoidable, nor the result of misuse, but will simply be unforeseen by those who designed them.105 Harms of this variety are by no means unique to the field of robotics. Indeed, unpredictability is part and parcel of any sufficiently complex system. It’s why your computer periodically crashes106 and perhaps why new typos seem to pop up in our writing even though we’ve read through a draft at least thirty times.107
But if the last decade of progress in the field of robotics has taught us anything, it is that robotics systems using machine learning techniques can be extremely hard to predict, rendering them particularly susceptible to causing unforeseen harms. This phenomenon owes, in large part, to the fact that machine learning systems “enter[ ] into a social world already in motion, with an existing set of assumptions and expectations about what is likely and unlikely, possible and impossible.”108 Yet because such systems are, by definition, empowered to learn with limited109 direct human intervention, the behaviors that they develop can also be unconstrained by the norms, assumptions, and expectations that implicitly govern humans.
Sometimes, this lack of constraint can lead to astonishing, utterly unintuitive results.110 Robots deployed using machine learning techniques, for example, have devised wholly new tactics for conquering strategy games,111 have inadvertently set off wars of proliferation with bots on online platforms (leading to bizarre pricing decisions),112 and have even invented “codewords” to communicate with other AI systems that were indecipherable by their designers.113 Because of this unpredictability, many complex robots will carry an enormous range of unforeseeable risks—even when numerous precautions are taken in advance of deployment.
To be clear, the unpredictability inherent in machine learning is also one of its greatest strengths. An AI that just engages in rote calculation of equations we already know the answer to might get to the result faster than humans can, but it won’t be any better at understanding or predicting outcomes than humans. We want AIs to do unpredictable things, so long as those things lead to good results. We already get many of our greatest innovations from the freedom to tinker with the existing world in unpredictable ways.114 The same is likely to be true of robots. If an AI can reliably conclude that butterfly population variance in Tibet affects the weather in Indonesia, it will be better than humans at predicting the weather. And if a self-driving car can conclude from subtle changes in the velocity of the cars surrounding it that a crash is imminent, it offers greater hope of avoiding such crashes than a human driver might.115
But the unpredictability of the path that robots will take to achieve their goals means that they may do things that make perfect sense given what they were asked to maximize, but which turn out to reflect either poorly specified goals or flawed training data. The Introduction’s example of a drone learning to intentionally sabotage its flight path provides just one of the now countless documented instances of unforeseen robot behaviors. Another comes from the healthcare domain.
In the 1990s, a pioneering multi-institutional study sought to use machine learning techniques to predict health-related risks prior to hospitalization.116 After ingesting an enormous quantity of data covering patients with pneumonia, the system learned the rule:
has_asthma(x) ⇒ lower_risk(x)
The colloquial translation is: “[P]atients with pneumonia who have a history of asthma have lower risk of dying from pneumonia than the general population.”117
The machine-derived rule was curious, to say the least. Far from being protective, asthma can seriously complicate pulmonary illnesses, including pneumonia. Perplexed by this counterintuitive result, the researchers dug deeper. And what they found was troubling.
They discovered that “patients with a history of asthma who presented with pneumonia usually were admitted not only to the hospital but directly to the ICU (Intensive Care Unit).”118 Once in the ICU, asthmatic pneumonia patients went on to receive more aggressive care, thereby raising their survival rates compared to the general population.119
The rule, in other words, reflected a genuine pattern in data. But the machine had confused correlation with causation—“incorrectly learn[ing] that asthma lowers risk, when in fact asthmatics have much higher risk.”120
Thankfully, the relative simplicity of the machine learning model deployed by the researchers in this instance allowed them to detect, reverse engineer, and remedy the situation before any harmful behavior resulted.121 Indeed, the algorithm taught humans something about the flaws in existing care techniques. But that is a luxury which will not be afforded to all robot designers.122 Indeed, as Dr. Marc Canellas et al. have convincingly argued, the likelihood of these types of unpredictable events actually tends to rise alongside the complexity of computational models, even though the overall likelihood of an abnormal event may remain constant.123 This phenomenon owes to the highly leptokurtic124 failure curves often observed in complex systems, in which a “reduced likelihood of failure in a general sense” tends to be accompanied by an increased “likelihood of more severe failures.”125
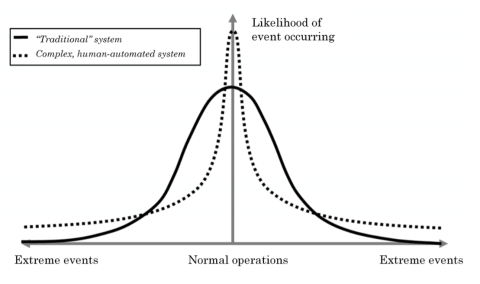
6. Systemic harms.
People have long assumed that robots are inherently “neutral” and “objective,” given that robots simply intake data and systematically output results.126 But they are actually neither. Robots are only as “neutral” as the data they are fed and only as “objective” as the design choices of those who create them. When either bias or subjectivity infiltrates a system’s inputs or design choices, it is inevitably reflected in the system’s outputs.127 Accordingly, those responsible for overseeing the deployment of robots must anticipate the possibility that algorithmically biased applications will cause harms of this systemic nature to third parties.
Robots trained on poorly curated data sets, for example, run the risk of simply perpetuating existing biases by continuing to favor historical haves against have-nots. In such instances, different outcome distributions in the data reflecting racial, ethnic, social, or economic disparities can become self-fulfilling prophecies—leaving already marginalized groups at the mercy of past injustices.128
Similarly, the algorithmic goals and subgoals that define robot behavior can also lead to biased results. After all, each decision in the process of developing an algorithm necessarily reflects the values of its designers. And when designers fail to consider particular stakeholders, or fail to specify goals that accurately map onto their desired outcomes, their robots may unfairly privilege certain individuals or groups over others. Hence, mathematician Cathy O’Neil’s provocative description of an algorithm as an “opinion[ ] embedded in mathematics.”129
Instances of bias or subjectivity infiltrating robotics systems are already well documented. A recent example comes from the car insurance industry. US law obliges all car owners to purchase insurance for their vehicles. But not all premiums are created equal. A recent study by Consumer Reports found that contemporary premiums depended “less on driving habits and increasingly on socioeconomic factors,” including an individual’s credit score.130 After analyzing “2 billion [car insurance] price quotes across approximately 700 companies,” the study found that “[c]redit scores . . . factored into [insurance] algorithms so heavily that perfect drivers with low credit scores often paid substantially more than terrible drivers with high scores.”131 The study’s findings raised widespread concerns that AI systems used to generate these quotes could “create negative feedback loops that are hard to break.”132 According to one expert, “Higher insurance prices for low-income people can translate to higher debt and plummeting credit scores, which can mean reduced job prospects, which allows debt to pile up, credit scores to sink lower, and insurance rates to increase in a vicious cycle.”133 Similar examples of robotics systems causing, or threating to cause, systemic harms have been documented in the domains of predictive policing, criminal sentencing, targeted advertising, search optimization, and facial recognition, among many others.134
To be sure, all advantages are comparative. AI may replicate bias in existing legal systems. But it also has the potential to reduce that bias by replacing human instinct with actual metrics.135 Used properly, AIs can reduce bias by replacing subjectivity with objectivity.136 But it is important that those new objective measures don’t simply replicate the problems of their subjective predecessors.
As we continue to invite robots into our homes, personal lives, and places of work, the types of collateral risks they pose to our privacy, security, environment, and even livelihoods will also grow in kind. Some harms, after all, simply arise as a by-product of pervasiveness. And the threat of these types of harms emerging will be especially true in modern robots, given that they often combine the uncertainties of machine learning, the “promiscuity of data,”137 the inherent security risks of computational systems, and the threat of physically affecting the real world.
Take, for example, the now commonplace phenomenon of inviting “Internet of Things”138 (IoT) devices, such as an Amazon Echo or Google Home, into our homes to monitor our every utterance. For many (ourselves included), the convenience of simply issuing a voice command to set a cookie timer, play a song, or order a cab can be too good to pass up. Yet, in exchange for the capabilities offered by these powerful voice recognition bots, we must also accept the reality of their 24-7 surveillance of our most intimate settings.
Data collection practices of this magnitude will not only present legal oversight challenges to those tasked with gathering it, but also present novel challenges for those seeking to secure robots against external threats. As Professor Balkin notes, “the more opportunities for innovation, the more possible targets for hacking.”139 Accordingly, the very same applications that now gather unprecedented amounts of data from users are also likely to pose unprecedented risks in the event that such data gets into the wrong hands.
Even if they aren’t hacked, the mere presence of these devices can change human behavior. People act differently when they think they are being watched or listened to, even if the thing doing the watching is only a picture of a pair of eyes taped to the computer.140 And if a robot is in your house, you’re not just imagining it: it probably is watching and listening to you.141
Add to this brave new reality the awesome power of cloud computing and networking technologies, and the threat of collateral harms is only exacerbated. Armies of robots linked through networking technologies will enable single, centralized systems to impact our physical and digital environments in profound new ways. Seemingly microscopic design choices within systems controlling fleets of tens of thousands of autonomous vehicles, for example, could produce macroscopic effects including changes to traffic patterns, transportation pricing, congestion, and even energy grid usage. We may, for example, wake up one morning to discover that Google Maps has routed highway traffic through our quiet neighborhood streets. Such a decision harms people who never use Google Maps or self-driving cars. But so might its opposite. Suppose, instead, that the same routing algorithm avoided residential areas entirely, causing greater congestion on highways and interstates than was socially optimal.
II. Remedies and Robots
The injuries we described in the last Part will lead to lawsuits of various types. Indeed, they already have.142 We don’t intend to discuss all the ways courts might apply the substantive law to those legal harms. There is a growing literature doing just that.143 Rather, our focus is on the practical endgame of these coming lawsuits: the law of remedies. Having identified a wrong, courts try to make it right by applying various remedies. But as we will see below, when the defendant is a robot (or its owner), that can be easier said than done. In Part II.A we summarize the law of remedies. In Part II.B we examine the fundamental nature of remedies law, detailing the “normative” and “economic” views as well as the practical implications of robots internalizing one view or the other. Having laid out the legal and moral foundations of remedies and robots, we then discuss how the technology will mesh with or challenge the various mechanisms our remedies rules currently rely on to deter, promote, or compensate for particular behaviors.
A. The Law of Remedies
A remedy, broadly defined, is anything that a judicial body can do for an individual who has been harmed or is threatened with harm. Remedies are the means by which substantive law is given its actual effect. Once a plaintiff is adjudged to have suffered harm under the laws governing primary rights and duties, the law must provide a remedy for those rights and duties to have meaning. Without a remedy, lawfulness and unlawfulness are rendered merely nominal distinctions—or, as it is often more pithily phrased, “No right without a remedy.”144
There are two fundamental kinds of remedies: those that are “compensatory” and those that are “preventative.”145 Compensatory remedies aspire to address the wrongs suffered by an individual through monetary transfers between plaintiff and defendant, compensating the plaintiff for the injury suffered. Preventative remedies, meanwhile, aspire to avoid this transfer entirely. They seek to discourage, avert, or literally undo harm, rather than retrospectively compensating victims once harm has occurred. Some preventative remedies accomplish this aim by threatening lawbreakers with damages, specific performance, or restitution in an effort to deter unlawful conduct. But sometimes courts seek to prevent harm more directly by enjoining individuals from acting or, less commonly, ordering them to take affirmative steps to avoid violating the law.146
One goal of remedies law is to make plaintiffs whole by restoring them to the condition they would have been in “but for” the wrong—what Professor Douglas Laycock calls restoring the “plaintiff’s rightful position.”147 Traditionally, this compensatory goal has focused on the plaintiff in the dispute—presumably a legal person.148 Compensation is normally accomplished through the award of legal damages.
But remedies law also focuses substantial attention on defendants. Equitable restitutionary remedies such as unjust enrichment, disgorgement, and constructive trust are designed not to compensate plaintiffs but to deprive defendants of the benefit of wrongful acts. These remedies are designed not to make the plaintiff whole, but to make the defendant “whole” (in the sense that he is no better off than he would have been but for the wrongdoing).149
Injunctive relief can serve the purpose of putting either the plaintiff or the defendant in her rightful position. Injunctions order the defendant not to act (or, less commonly, to take some affirmative act). Generally, injunctions are designed to prevent a future harm or stop an ongoing one. But they can also aim to make affirmative changes in the world by seeking to change existing structures that have led to past injuries.150
Remedies law also contains many elements of moral judgment, punishment, and deterrence.151 For instance, the law will often act to deprive the defendant of gains, even if the result is a windfall to the plaintiff, because we think it is unfair to let the defendant keep those gains. Courts may also enhance damages beyond what is necessary to compensate plaintiffs or deprive defendants of profits in order to punish behaviors we deem reprehensible.
Most of these noncompensatory remedies laws were explicitly designed to change the behavior of people. But the remedial mechanisms used to shape human behavior cannot be relied upon to do the same when machines, not people, engage in harmful conduct. The remainder of this Part considers some of the complications that robots bring to various remedies rules.
B. The Nature of Remedies
This Section examines the principles that motivate the law of remedies and how robots complicate our traditional understanding. In Part II.B.1 we explain the normative and economic perspectives on the substantive law of remedies. In Part II.B.2 we examine how remedies law must adapt to accommodate robots.
1. Normative versus economic perspectives.
The choice of remedy for a given legal violation often stems from fundamental assumptions regarding the nature of the substantive law itself. Two views predominate. A “normative” view of substantive law sees it as a prohibition against certain conduct, with the remedy being whatever is prescribed by the law itself. The defendant, on this view, has engaged in a wrongful act that we would stop if we could. But because it is not always possible to do so—commonly because the act has already occurred—remedies law seeks to do the next best thing: compensate the plaintiff for the damage done. This view is consistent with laws enforced by property rules.152
An alternative view of substantive law, however, conceptualizes the role of remedies differently. Under this “economic” view, the substantive law alone forbids nothing. Rather, it merely specifies the foreseeable consequences of various choices, with the available remedies signaling the particular penalties associated with particular conduct. Damages, on this view, are simply a cost of doing business—one we want defendants to internalize but not necessarily to avoid the conduct altogether.153 This approach is more commonly associated with liability rather than property rules.154
To help illustrate the difference between these two views, consider an everyday encounter with a traffic light. Under the normative view, a red light stands as a prohibition against traveling through an intersection, with the remedy being a ticket or fine against those who are caught breaking the prohibition. We would stop you from running the red light if we could. But because policing every intersection in the country would be impossible, we instead punish those we do catch in hopes of deterring others.
Under the economic view, however, an absolute prohibition against running red lights was never the intention. Rather, the red light merely signals a consequence for those who do, in fact, choose to travel through the intersection. As in the first instance, the remedy available is a fine or a ticket. But under this view, the choice of whether or not to violate the law depends on the willingness of the lawbreaker to accept the penalty.
In one of his more arresting turns of phrase, Justice Oliver Wendell Holmes Jr famously described the economic view of substantive law as that of a “bad man.” According to Justice Holmes:
If you want to know the law and nothing else, you must look at it as a bad man, who cares only for the material consequences which such knowledge enables him to predict, not as a good one, who finds his reasons for conduct, whether inside the law or outside of it, in the vaguer sanctions of conscience.155
The measure of the substantive law, in other words, is not to be mixed up with moral qualms, but is simply coextensive with its remedy—no more and no less.
While some law and economics scholars accept this precept as fundamental, in many behavioral contexts it does not tell the entire story. Although the actual consequences associated with lawbreaking play a substantial role in much of human decision-making, many individuals nonetheless view law as having distinctly normative underpinnings. As Laycock notes, “It is certainly true that some individuals will obey the law only if the consequences of violation are more painful than obedience,” but the fact that “some individuals are unmoved does not eliminate the statement’s moral force for the rest of us.”156
An illustrative example of this phenomenon in action comes from the Ohio case French v Dwiggins157 involving a fatal motorcycle accident.158 At issue was a recently passed statute expanding the avenues of recovery available to plaintiffs who pursued wrongful death claims. The court wrote that, although the expansion of remedies coincided with the timing of the accident, the defendant “could not reasonably be expected to conduct his affairs differently” than he would have under the prior regime.159 The court reasoned that when it came to this life and death matter, the marginal differences in available remedies played no role in the defendant’s decision-making leading up to the accident.
Justice Holmes, himself, could hardly have been said to disagree with the court’s reasoning.160 Despite his provocative use of the “bad man” metaphor to clarify the role of the legal rules for those acting out of pure self-interest, he understood the complex—and oftentimes competing—roles that normative concerns play in everyday decision-making.161
2. Bad men and good robots.
People are rarely forced to grapple with the distinctions between the normative or economic view of substantive law.162 But robots, or at least their programmers, are afforded no such luxury. Sure, robots can be prohibited from engaging in certain types of conduct, assuming their designers understand and control the algorithm by which they make decisions. But implementing a legal remedy via computer code necessarily involves adopting either a normative or economic view of the substantive law.
That’s because a true “prohibition” can only be communicated to a computer system in one of two basic ways: it can be encoded in the form of an “IF, THEN”163 statement that prevents a robot from engaging in particular types of conduct, or it can be coded as a negative weight for engaging in that same conduct. An IF, THEN statement operates like an injunction, while a weight in a decision-making algorithm operates like a liability rule.
Returning to the example of the red light, a programmer seeking to prohibit a robot from breaking the law could do so with an IF, THEN statement along the lines of: “If the robot encounters a red light, then it will not travel into the intersection.” Similarly, a programmer seeking to achieve that same prohibition in a probabilistic system could do so by assigning an infinitely high negative consequence to traveling into the intersection when the light is red.
An IF, THEN statement is an absolute rule. If a triggering event occurs, then a particular consequence must inexorably follow. As a practical matter, so is an infinitely negative weight. Both achieve the functionally equivalent result of prohibiting the unlawful conduct—the goal of a normative vision of substantive law. But in order to achieve this normative vision, the prohibition must be implemented without regard for the cost of a ticket.
Because the law is encoded as an absolute in its programming, the robot will always obey the law. That’s not true of people. If we want legal rules to be self-executing, the ability to impose perfect obedience may be a good thing.
By contrast, if the underlying theory of a remedy is economic, the machine’s decision-making calculus is fundamentally different. Once more, the example of the traffic light helps to clarify this distinction. To an economist, the substantive law and its remedy do not signal a “self-executing refusal to ever run a red light” but instead an understanding that “running a red light is associated with a small chance of a modest fine and a somewhat increased chance of a traffic accident which will damage the car and may require the payment of damages to another.” Under this view, the remedy, and its risks, are both expressed in probabilistic terms. They translate into probabilistic costs within the robot’s overall decision-making calculus. Those costs won’t be infinite, unless perhaps the penalty is death.164 They will instead reflect a “price” for running a red light that the algorithm might decide to pay depending on what benefits light-running offers.
Thus, under the economic view, the choice of whether to obey a law is, of necessity, the choice of a Holmesian “bad man.” Normative views of substantive law—which we know shape certain aspects of human behavior—cannot be expected to translate cleanly into the robotics context with their associated remedies intact. If we want robots to adopt normative views of the law, we will need outright prohibitions of the type that famously got Isaac Asimov’s robots into so much trouble.165 And imposing bans rather than simply calculating costs will make it hard for robots to achieve many things. After all, it’s hard to operate a robot with too many absolute prohibitions.166 And this will be particularly true of machine learning systems that develop their own algorithms, making it difficult for engineers to reliably predict how encoded prohibitions will interact with other rules.
Encoding the rule “don’t run a red light” as an absolute prohibition, for example, might sometimes conflict with the more compelling goal of “not letting your driver die by being hit by an oncoming truck.” Humans know that “don’t run a red light” doesn’t really mean “don’t ever run a red light.” Rather it translates, roughly, to “don’t run a red light unless you have a sufficiently good reason and it seems safe.” Likewise, even weightier normative prohibitions, such as “thou shalt not kill,” come with an implied “unless . . . .” But designers can’t put that in an IF, THEN statement unless they understand and specify all the exceptions to the rule.
More plausibly, robots operating in the real world will have to adopt algorithmic approaches to almost all complex problems that weigh particular actions against various goals and risks. As a result, the role of remedies in discouraging socially detrimental conduct will need to be reimagined in terms of cost internalization,167 as opposed to normative sanction or punishment. Deterrence makes sense where we are trying to affect individual behavior. But the logical way to “deter” a machine is to put the actual costs into the calculus it uses to make the decision. In practice, that translates into quantifying, and then operationalizing, the price we want robots to have to pay if they take certain actions we want to deter.168 And under the broadest interpretation of the economic view, even doctrines seemingly designed to prevent or deter conduct—like injunctions or prison sentences—could simply be construed as costs, albeit very high ones.
That said, we think it makes more sense to distinguish between remedies designed to internalize costs and those designed to enjoin, deter, or punish behavior.169 While some defendants faced with the latter may treat punitive damages or even prison sentences as mere costs of doing business, the remedy’s ultimate intent is to deter unlawful conduct, not to simply internalize its social costs.
For the vast majority of applications, legal remedies will likely be incorporated into machines through their “economic” formulation—resulting in robots that, by design, adopt this view of substantive law exclusively. Unless specifically programmed otherwise, distinctions between normative and economic goals will be utterly lost on robots. Thus, while it may be true to say that it is the rare “individual[ ] [who] will obey the law only if the consequences of violation are more painful than obedience,”170 this will be definitionally true of robots. And for reasons made clear in virtually every sci-fi plot line featuring robots, it will only be on the rarest of occasions that it actually makes sense to completely bar robots from engaging in certain types of conduct.
It thus appears that Justice Holmes’s archetypical “bad man” will finally be brought to corporeal form, though ironically, not as a man at all. And if Justice Holmes’s metaphorical subject is truly “morally impoverished and analytically deficient,” as some accuse, it will have significant ramifications for robots.171
C. Teaching Robots to Behave
Each of the major types and purposes of remedies we identified in Part II.A will face challenges as applied to robots and AI. In this Section, we consider each in turn.
1. Who pays?
The first purpose of damages—to compensate plaintiffs for their losses and so return them to their rightful position—is perhaps the easiest to apply to robots. True, robots don’t have any money (unless we count the one that was recently arrested by Swiss police after going on a black-market shopping spree with $100 worth of Bitcoin).172 So they generally can’t actually pay damage awards themselves.173 In fact, the European Parliament specifically cited this fact in its recommendation against giving robots personhood, noting that they are not fully functioning members of society that could afford to pay their debts.174
But this problem is hardly insurmountable. The law will rise to the challenge. Someone built the robots, after all. And someone owns them. So if a robot causes harm, it may make sense for the company behind it to pay, just as when a defective machine causes harm today.
But it’s not that easy. Robots are composed of many complex components, learning from their interactions with thousands, millions, or even billions of data points, and they are often designed, operated, leased, or owned by different companies. Which party is to internalize these costs? The one that designed the robot or AI in the first place? The one that collected and curated the data set used to train its algorithm in unpredictable ways? The users who bought the robot and deployed it in the field? Sometimes all of these roles will be one in the same, falling upon individuals operating in a single company, as was arguably the case when a self-driving Uber car killed a pedestrian in Tempe, Arizona.175
In such instances, assigning responsibility may be easy. But often the chain of legal responsibility will be more complicated. Is a self-flying passenger drone an inherently dangerous product? If so, one set of rules might apply depending on whether a passenger or, instead, a third party is injured. Is the injury caused by this hypothetical drone the result of a design defect? If so, it may be the designer who should bear the risk.176 But suppose instead that the injury was the result of a software defect that a different designer introduced through an aftermarket modification. Here, the law commonly shifts responsibility away from the manufacturer if the modification was one that the manufacturer didn’t intend.177 Indeed, companies regularly void warranties when third parties modify their products or use them in unexpected ways. Things will get even more complicated if, as seems likely, some or all of the robot code is open source, raising the question of who ultimately is responsible for the code that goes into the robot.178
Robot designers, owners, operators, and users will, of course, fight over who bears true legal responsibility for causing the robot to behave the way it did. And these complex distinctions don’t even account for the role of third parties causing robots to behave in adverse ways, as recently happened when Microsoft’s chatbot, Tay, turned into a proverbial Nazi after interacting with trolls on Twitter.179
These problems aren’t new, of course. Suppliers in a product chain have blamed each other when things go wrong for a long time, and courts have had to sort those claims out. Responsibility issues for robots too can, and will, eventually be resolved by the courts. But long before any consensus is reached, we should expect no shortage of finger-pointing, as different companies and individuals clamor to shift responsibility for harms to others in the causal chain—whether just to minimize their costs or because there are legitimate disputes about how the behavior of different actors in the chain interacted to cause the harm. And there may be one important difference between past disputes and those involving robots: if the AI is self-learning, we may really never know who is to blame.180
2. Law as action: shaping the behavior of rabota economicus.
The second prong of the remedies triad—damage awards and equitable remedies designed to internalize costs and deter socially unproductive behavior—will likely prove even more problematic. If we want to deter a robot, we need to make sure that it is programmed to account for the consequences of its actions. Embedding this type of decision-making in robots often means quantifying the various consequences of actions and instructing the robot to maximize the expected net monetary benefits of its behavior.
This might sound like heaven to an economist. Finally, we will have a truly rational homo economicus (or, more accurately, a rabota economicus)181 who will internalize the social costs of its actions (at least insofar as those costs are accurately calculated in the courts) and modify its behavior accordingly. And if machine learning systems estimate these costs correctly, robots will be “Learned” indeed—presumably deciding to do harm only when it is socially optimal (that is, when B < PL).182
But not so fast. Things are more complicated. Robots won’t reflexively care about money. They will do whatever we program them to do. We can align robot incentives with social incentives by properly pricing, punishing, or deterring the companies that design, train, own, or operate robots. Those companies, in turn, should internalize the relevant costs of their robots’ actions. It might be reasonable to assume that corporations and people want to maximize their rational self-interest and will, thus, program their robots accordingly. But not all will, either intentionally or unintentionally. There are at least three potential problems.
First, the goal of cost internalization through legal liability can only be accomplished by proxy. And it isn’t clear who the proxy will be. All the problems we noted in the prior section about assigning responsibility to compensate victims will return in spades as we try to force robots to account for the costs of their conduct. Even truly rational, profit-maximizing companies with perfect information about the costs of their actions won’t internalize those costs unless they expect the legal system to hold them liable. If they are wrong, either in fearing liability when none exists or in believing someone else will foot the bill, their pricing will not accurately reflect reality.
Second, we are unlikely to have anything resembling “perfect” information about the potential harms robots may cause. As noted in Part I, robots operating in complex environments can do a wide variety of harmful things. Some of those things we want to stop altogether. Some we want to discourage except in unusual circumstances. Some we want to outright permit but still price appropriately to account for externalities imposed on others. And some we want to permit despite their costs to society because the alternatives are worse.
Getting robots to make socially beneficial, or morally “right,” decisions means we first need a good sense of all the things that could go wrong. Unfortunately, we’re already imperfect at that. Then we’d need to decide whether the conduct is something we want to ban, discourage, tax, or simply permit. Having done so, we would then need to decide who in the chain of robot design, training, ownership, and operation should be responsible for the harm, if anyone. Then, we would need to figure out how likely each adverse outcome is in any given situation. Finally, we would need to assign a price to those potential harms—even the amorphous ones, such as a reduction in consumer privacy. And we’d want to balance those harms against reasonable alternatives to make sure the decision the robot made was the right one, even if it did cause harm.
Our entire system of tort law has been trying to accomplish this feat for centuries. And it hasn’t worked very well. Indeed, most of tort is composed of standards, as opposed to hard and fast rules, for good reason. Standards give us the leeway to reserve judgment for later, when we might have a better idea of the actual facts leading up to an event.
Tort law, for example, requires us to value injury, and—if we are to deter conduct—to decide on a multiplier to that value that serves as an optimal deterrent. While there are some circumstances in which we calculate these values formulaically,183 the primary way we do so is by leaving it to juries to pick the right number after an injury has already occurred. Effective deterrence in robots would, therefore, require accurate predictions about how juries might assess specific harmful events, not to mention a host of other computationally complex considerations. Scholars already find these types of predictions difficult, if not impossible, in the human context.184 And we know virtually nothing of how juries will react to harmful events caused by robots, particularly those exhibiting behaviors they can’t understand because the algorithm is inscrutable.185 As we discuss below,186 this reality on the ground may even lead to feedback loops, in which the very act of trying to price harms in a decision-making algorithm changes the jury’s view of the robot’s responsibility.187
The problem is even more complex than that, though, because robots don’t necessarily care about money. They will maximize whatever they are programmed to maximize. If we want them to internalize the costs of their behavior, we will need to put those costs in terms robots can understand—for example, as weights that go into a decision-making algorithm. That’s all well and good for robots already designed to maximize profit in purely monetary terms—say, a day-trading AI. But lots of robots will be designed with something other than money in mind. A policing or parole algorithm might minimize the likelihood that a released offender commits another crime. A weather-prediction system may maximize successful prediction outcomes. A surgery robot might maximize success in the surgery without considering certain side effects down the road. And a self-driving car might minimize time to destination subject to various constraints like generally obeying traffic laws and reducing the risk of accidents. But to build deterrence into those algorithms, we must convert certain divergent values into a common metric, whether it be money or something else.
A third complexity involving rabota economicus emerges for economic costs that are not directly reflected by legal remedies. The cost of any given decision, after all, is not just a function of the legal system. In many instances, extralegal forces such as ethical consumerism, corporate social responsibility, perception bias, and reputational costs will provide powerful checks on profit-maximizing behaviors that might, otherwise, be expected to produce negative societal externalities.188 By pricing socially unacceptable behavior through the threat of public backlash, these and other market forces help to fill some of the gaps left by existing remedies regimes. But they may open up other holes, creating rather than internalizing externalities. In fact, in certain circumstances, these factors may end up utterly swamping the costs of actual legal liability. For instance, if I make it clear that my car will kill its driver rather than run over a pedestrian if the issue arises, people might not buy my car. The economic cost of lost sales may swamp the costs of liability from a contrary choice. (In the other direction, car companies could run into PR problems if their cars run over kids.) Put simply, it is aggregate profits—not just profits related to legal sanctions—that will drive robot decision-making.
Further, even when a profit-maximizing corporation is wholly responsible for the conduct of a robot, incentives may misalign for other reasons. Corporations might want robots that maximize the long-term value of their brand even if doing so imposes unnecessary hidden costs. Conversely, they may task their robots with creating content that goes viral and, therefore, maximizes short-term visibility—even if it is divisive and potentially contrary to the corporation’s long-term interest. Corporations may also decide that first-mover advantages are worth the risk of causing some injury in order to capture a long-term market. “Move fast and break things” is a slogan in Silicon Valley, one that has served many disruptive tech companies well.189 But this same slogan can take on a somewhat more sinister cast when it is self-driving cars that are literally moving fast and breaking things.
Corporations are also likely to be siloed in ways that interfere with effective cost internalization. Machine learning is a specialized programming skill, and programmers aren’t economists.190 Even those who are employed by profit-maximizing companies interested in effectively internalizing their legal costs may see no reason to take the law into account, or may not be very good at it even if they try to. They may resent constant interference from the legal department in their design decisions. And agency costs mean that different subgroups within companies may be motivated by different incentives—as when sales divisions, manufacturing divisions, and service departments all get compensated based on different and potentially conflicting metrics.191
Furthermore, designers aren’t the only people whose motivations we need to worry about. What a self-learning robot will maximize depends not only on what it is designed to do—the default optimizing function or functions that it starts with—but also how it learns. To efficiently deter behavior, we must be able to predict it. But if we don’t know how the robot will behave because it might discover novel ways of achieving the goals we specify, simply pricing in the cost of bad outcomes might have unpredictable effects, such as shutting down a new and better approach that produces some bad results but is nonetheless worth it. And even if it doesn’t, we once again have to confront the possibility that not all engineers will design their robots to maximize profit. Even if the designer of my self-driving car defaults to an algorithm that appropriately balances the risks to everyone associated with driving, I might personally prefer a car that protects its passengers at the expense of pedestrians. And if I (or, more realistically, a car company that wants to market to me) instruct the car accordingly, simply pricing the social cost of accidents into the algorithm won’t modify behavior in the way we hope.
This complex relationship between deterrence, responsibility, and financial liability does not, alone, differentiate robots from corporations or people. Deterrence is imperfect among humans, too, because humans aren’t motivated entirely by money and because they can’t always pay for the harm they cause. But what is different here is that the possibility of deterrence working at all will depend entirely on the robot’s code. A robot programmed to be indifferent to money won’t be deterred by any level of legal sanction. And while making the responsible legal party pay192 might encourage that party to design robots that do take adequate care, the division of responsibility between component makers, software designers, manufacturers, users, owners, and third parties means that the law must be careful about who exactly it holds accountable.193
D. Deterrence without Rational Actors: Is There Still a Role for Morality and Social Opprobrium in Robot Remedies?
People often assume that robots are rational actors. But because robots act based upon their underlying code, that assumption will not always manifest in ways we would expect of rational human actors. Our legal system needs to address that difference. In Part II.D.1, we consider equitable remedies in the context of deterring “bad” robot behavior. In Part II.D.2, we consider punitive damages and other forms of punishment.
1. Equitable monetary relief and punishment.
So far, we have focused on internalizing the costs of accidents or other injuries that result from otherwise socially desirable activities, such as driving cars. But we also need to worry about genuinely “bad” behavior by robots that may merit prohibition. Many of our equitable monetary remedies are aimed at this sort of conduct. Their goal is not to make defendants internalize costs—to put a price on socially valuable behavior because of the costs it imposes—but to prevent the behavior. If you steal my car, the law says that you don’t get to keep it even if you value it more than me. Rather, you hold it in constructive trust for me.194 If you make profits by infringing my copyright or trade secret (but not my patent), the law will require you to disgorge those profits, paying me the money you made even if I never would have made it myself.195 We require defendants to give up such “unjust enrichment,” not because we think we need to do so to compensate the plaintiff, but because we don’t want the defendant to have the money.196
These equitable rules share some similarities with the cost-internalization measures discussed in the last Section. But there are two key differences: (1) the money a defendant must pay is not limited to what is needed to compensate the plaintiff, and (2) the defendant must give up all gains, making the entire activity unprofitable. The focus here is not on the plaintiff’s rightful position but on the defendant’s rightful position. And in the class of cases in which we often use these remedies, the defendant’s rightful position is one in which she didn’t engage in the activity at all.197
From an economic perspective, depriving defendants of their gains is simply a matter of coming up with a number. It might be greater than, equal to, or less than the damages we would otherwise impose to internalize the costs of unlawful conduct or to restore the plaintiff’s rightful position. But there is something psychologically effective about taking away a defendant’s gains altogether. Indeed, in certain contexts, it might be a better means of deterring humans than the threat of paying compensatory damages, even if those damages turn out to be higher than a disgorgement remedy would.198 When it comes to robots, however, there is little reason to think that the notion of taking “all your profits” will have the same psychological effects. True, if you set “profit = 0,” a profit-maximizing AI would not engage in the conduct. But that same logic would apply with equal force if the damages award made the activity unprofitable too.
Remedies focused on the defendant’s rightful position do have one significant economic advantage over damages remedies intended strictly as ex ante deterrents: we can calculate them after the fact once we have all the necessary information. If we want to use the threat of damages to deter conduct, we need to predict the likelihood and severity of the harm that the conduct will cause.199 But if we care only about depriving the defendant of benefits on the theory that doing so will deter her, we just need to wait to set the number until the parties get to court and figure out how much the defendant actually gained. That often won’t be trivial. The benefit of stealing a trade secret, for example, can be as amorphous as a “quicker time to market” or a “more competitive product.”200 But it’s still likely to be easier than predicting in advance who will be injured and by how much.
This same calculus doesn’t work for injuries that are the by-product of productive behavior. It doesn’t make sense to say that a self-driving car that hits a pedestrian should disgorge its profits. It likely didn’t profit from hitting the pedestrian. And we don’t want to force defendants to disgorge all the value they make from driving. But defendant-focused equitable monetary remedies, like disgorgement or constructive trust, may have advantages for robot torts for which our goal is to stop the conduct altogether, not simply to price it efficiently.
2. Detection, deterrence, and punitive damages.
The fact that robots won’t be affected by the psychological impact of certain remedies also has consequences for how we should think about the threat of detection. For a robot to be optimally deterred by remedies like disgorgement—which rely on human psychology to maximize their effects—we must also detect and sanction the misconduct 100 percent of the time.201 That, in turn, leads us to the problem of robots (or their masters) that hide misconduct.
To be sure, many robot harms will be well-publicized. The spate of autonomous vehicle accidents covered by media in recent years provides one stark example. But countless robot harms will be of far subtler, so-called black box,202 varieties and will, therefore, be much harder to detect.203
Makers and trainers of robots may have incentives to hide their behavior, particularly when it is profitable but illegal. If a company’s parole algorithm concludes (whether on the merits of the data or not) that black people should be denied parole more often than similarly situated white people, it might not want the world to know. And if you, as an owner, tweaked the algorithm on your car to run over pedestrians rather than put your own life at risk, you might seek to hide that too. We have already seen remarkable efforts by companies conspiring to cover up wrongdoing, many of which succeeded for years.204 Often such conspiracies are brought down by sheer virtue of their scale—that is, the fact that many people know about and participate in the wrongdoing. This same property may be less true of future robotics firms, which may require fewer people to participate and cover up unlawful acts.205
Further, robots that teach themselves certain behaviors might not know they are doing anything wrong. And if their algorithms are sophisticated enough, neither may anyone else for that matter.206 Deterrence will work on a robot only if the cost of the legal penalty is encoded in the algorithm. A robot that doesn’t know it will be required to disgorge its profits from certain types of conduct will not accurately price those costs and so will optimize for the wrong behaviors.
The economic theory of deterrence responds to the improbability of getting caught by ratcheting up the sanctions when you are caught, setting the probability of detection times the penalty imposed equal to the harms actually caused.207 Proportionality of punishment makes sense here. As the chance of detection goes down we want the damage award to go up. And machines can do this math far better than humans can.208 Indeed, this idea may be tailor-made for robots. Professor Gary Becker’s “high sanctions infrequently applied” approach seems unfair in many human contexts because it can have widely varied interpersonal effects: even if we get equal deterrence from a 100 percent chance of a year in prison or a 10 percent chance of ten years in prison, the lottery system that punishes a few very harshly seems intuitively unfair. We want our laws to protect both victims and wrongdoers against some forms of moral bad luck (whereas Becker’s approach exacerbates it). But robots will internalize the probability of punishment as well as its magnitude, so we may be able to encourage efficient behavior without worrying about treating all robots equitably. Further, we are unlikely to feel bad for harshly punished robots in the ways that we might for human beings.209
Even if we decide to heed Becker’s advice, getting the numbers right presumes that we have a good estimate of the proportion of torts committed by robots that go undetected. That’s tough to do, especially for newly introduced technologies. And it also requires programmers to predict the multiplier and feed those calculations into the algorithm, something that might not be a straightforward undertaking for any of the variety of reasons covered in the last Section (not to mention the possibility that we get the numbers wrong, which will either over- or under-deter certain behaviors).210
Maybe society will instead be able to force corporations to internalize their costs through nonlegal mechanisms—for example, by voting with their wallets when a company’s robots engage in misconduct. But this, too, is easier said than done, particularly for the types of systemic harms described in Part I. In the era of big data and even bigger trade secrets, structural asymmetries often prevent meaningful public engagement with the data and software critical to measuring and understanding the behavior of complex machines. Because private companies retain almost exclusive control over both the proprietary software running the machines and their resultant data,211 barriers to accessing the information necessary to understand the reasons behind particular machine decisions can often be insurmountable. What’s more, even in circumstances in which the information is available, evidence of unlawful decision-making can still be notoriously difficult to detect. As the AI Now Institute notes, “Unintended consequences and inequalities [of sophisticated computational systems] are by nature collective, relative, and contextual, making measurement and baseline comparisons difficult” and creating the “potential for both over- and under-counting biases in measurement of distributions given the limits on observable circumstances for individuals, problems with population gaps and possible measurement errors.”212
Current trends in AI appear likely to only exacerbate this problem. As Bryce Goodman and Seth Flaxman observe, even after “[p]utting aside any barriers arising from technical fluency, and also ignoring the importance of training the model,” modern machine learning techniques pose significant “tradeoff[s] between the representational capacity of a model and its interpretability.”213 Systems capable of achieving the richest predictive results tend to do so through the use of aggregation, averaging, or multilayered techniques which, in turn, make it difficult to determine the exact features that play the largest predictive role.214 Thus, even more so than with the past generation of algorithms governing machines, understanding how modern robots arrive at a given decision can be prohibitively difficult, if not technically impossible—even for the designers themselves.215 As a result, potentially unlawful or defective decision-making within such systems can often only be demonstrated in hindsight, after measuring the unevenly distributed outcomes once they have already occurred. And as systems get more complex, maybe not even then.
The risk presented by this combination of factors is not so much that corporations will intentionally build bad robots in order to eke out extra profits, but that “[bad] effects [will] simply happen, without public understanding or deliberation, led by technology companies and governments that are yet to understand the broader implications of their technologies once they are released into complex social systems.”216 Indeed, much of the misconduct that tomorrow’s designers, policymakers, and watchdogs must guard against might not be intentional at all. Self-learning machines may develop algorithms that take into account factors we may not want them to, like race or economic status.217 But on some occasions, taking precisely those factors into account will actually get us to the ultimate result of interest.
For this reason, we think AI transparency is no panacea.218 Transparency is a desirable goal in the abstract. But it may inherently be at odds with the benefits of certain robotics applications. We may be able to find out what an AI system did. But, increasingly, we may not be able to understand why it did what it did.219 Calls for transparency are useful to the extent that they identify bad behavior, defective designs, or rogue algorithms. But mostly what people want when they talk about transparency is an explanation they can understand.220 Why was my loan application denied? Why did the car swerve in the way it did? For some robots, we simply won’t know the answer.221 Even if we see how the algorithm comes to a conclusion, we won’t necessarily be able to understand how it derived a relationship between, say, butterfly populations in Mongolia and thunderstorms in Ethiopia, or why it thinks the precise time of day and year should affect the speed at which it proceeds through an intersection.222
Are we right to be bothered by this? Should we have a right to understand the mens rea of robots? Or to impute explanations so we can appropriately channel opprobrium? Our punitive and deterrence remedies are based on identifying and weeding out bad behavior. The search for that bad behavior is much of what drives the “intuitive appeal of explainable machines.”223 But our intuitions may not always serve us well. The question is whether the demand for an explanation is actually serving legitimate purposes (Preventing Skynet? Stopping discrimination?) or just making us feel that we’re the ones in charge.224 The punitive and equitable monetary side of remedies law wants to understand the “why” question because we want to assign blame. But that might not be a meaningful question when applied to a robot. More on this later.225
3. Inhuman, all too inhuman.
- Punishing robots for responding to punishment. Even economic forms of deterrence—both legal and extralegal—will look different than they currently do when people or corporations are being deterred. Deterrence of people often takes advantage of cognitive biases and risk aversion. People don’t want to go to jail, for instance, so they will avoid conduct that might lead to that result. But robots can be deterred only to the extent that their algorithms are modified to include external sanctions as part of the risk-reward calculus.226
Once more, we might view this as a good thing—the ultimate triumph of a rational law and economics calculus of decision-making. But humans who interact with robots may demand a noneconomic form of moral justice even from entities that lack the human capacity to understand the wrongfulness of their actions (a fact that anyone who has ever hit a malfunctioning device in frustration can understand).227
Indeed, the sheer rationality of robot decision-making may itself provoke the ire of humans. Any economist will tell you that the optimal number of deaths from many socially beneficial activities is more than zero. Were it otherwise, our cars would never go more than five miles per hour. Indeed, we would rarely leave our homes at all.
Effective deterrence of robots requires that we calculate the costs of harm caused by the robots interacting with the world. If we want a robot to take optimal care, we need it to figure out not just how likely a particular harm is but how it should weight the occurrence of that harm. The social cost of running over a child in a crosswalk is high. But it isn’t infinite.228
Even today, we deal with those costs in remedies law unevenly. The effective statistical price of a human life in court decisions is all over the map.229 The calculation is generally done ad hoc and after the fact. That allows us to avoid explicitly discussing politically fraught concepts that can lead to accusations of “trading lives for cash.”230 And it may work acceptably for humans because we have instinctive reactions against injuring others that make deterrence less important. But in many instances, robots will need to quantify the value we put on a life if they are to modify their behavior at all. Accordingly, the companies that make robots will have to figure out how much they value human life, and they will have to write it down in the algorithm for all to see (at least after extensive discovery).
The problem is that people strongly resist the idea of actually making this calculus explicit.231 They oppose the seemingly callous idea of putting a monetary value on a human life, and juries punish companies that make explicit the very cost-benefit calculations that economists want them to make.232 Human instincts in this direction help explain why we punish intentional conduct more harshly than negligent conduct. A deliberate decision to run over a pedestrian strikes us as worse than hitting one by accident because you weren’t paying attention. Our assumption is that if you acted deliberately, you could have chosen not to cause the harm, thereby making you a bad actor who needs to modify your behavior. But that assumption often operates even when causing that harm was the socially responsible thing to do, or at least was justified from a cost-benefit perspective.
Things are more complicated, of course. We do try to create justifications and excuses in the law, even for intentional conduct that we think is socially acceptable. But juries often have a visceral desire to hold someone responsible when bad things happen. And they are inclined to treat killing or injuring a human being as a bad act even if it was (statistically) inevitable. They will rebel against treating it as a mere cost of doing business. Thinking about it in such terms offends many people’s sense of human decency.
- Punishment as catharsis: punching robots. Punishment may serve other, nonmonetary purposes as well. We punish, for instance, to channel social opprobrium. That can set norms by sending a message about the sorts of things we won’t tolerate as a society. And it may also make us feel better. We have victim allocution in court for good reason, after all. It may provide useful information to courts. But it also helps people to grieve and to feel their story has been heard.
Our instinct to punish is likely to extend to robots. We may want, as Professor Mulligan puts it, to punch a robot that has done us wrong.233 Certainly people punch or smash inanimate objects all the time.234 Juries might similarly want to punish a robot, not to create optimal cost internalization but because it makes the jury and the victim feel better.235
That kind of expressive punishment may also stem from the fact that much human behavior is regulated by social sanction, not just law. Aggressively signaling social displeasure doesn’t just make us feel better; it sends an object lesson to others about what is not acceptable behavior. Our instinct makes us want to send that lesson to robots too.
It’s already quite easy to think of robots as humans.236 We naturally anthropomorphize.237 That instinct is likely to get stronger over time, as companies increasingly deploy “social robots” that intentionally pull on these strings.238 Humans will expect humanlike robots to act, well, human. And we may be surprised, even angry, when they don’t. Our instinct may increasingly be to punish humanoid robots as we would a person—even if, from an economic perspective, it’s silly.239 Making us feel better may be an end unto itself. But hopefully there is a way to do it that doesn’t involve wanton destruction of or damage to robots.
E. Ordering Robots to Behave
All these problems with monetary remedies as deterrents seem to point in the direction of using injunctive relief more with robots than we currently do with people. Rather than trying to encourage robot designers to build in correctly priced algorithms to induce efficient care, wouldn’t it be easier just to tell the robot what to do—and what not to do?
1. Be careful what you wish for.
First, the good news: injunctions against robots might be simpler than against people or corporations because they can be enforced with code. A court can order a robot, say, not to take race into account in processing an algorithm. Likewise, it can order a self-driving car not to exceed the speed limit. Someone will have to translate that injunction, written in legalese, into code the robot can understand. But once they do, the robot will obey the injunction. This virtual guarantee of compliance seems like a significant advantage over existing injunctions. It is often much harder to coerce people (and especially groups of people in corporations) to comply with similar court orders—even when the consequences are dire.
But once again, not so fast. As the adage goes (and as legions of genies in bottles have taught us): be careful what you wish for. Automatic, unthinking compliance with an injunction is a good idea only if we’re quite confident that the injunction itself is a good idea. Now, obviously the court thinks the injunction improves the world. Otherwise, it wouldn’t issue it. But the fact that injunctions against people aren’t self-enforcing offers some potential breathing room for parties and courts to add a dose of common sense when circumstances change. This is a common problem in law. It’s a major reason we have standards rather than rules in many cases.240 And it’s the reason that even when we do have rules, we don’t enforce them perfectly. To a person (and even to a police officer), “don’t exceed the speed limit” implicitly means “don’t exceed the speed limit unless you’re rushing someone to the emergency room or it would be unsafe not to speed.” “Don’t cross the double yellow line” implicitly means “don’t cross the double yellow line unless you need to swerve out of the lane to avoid running over a kid.” No cop is going to ticket you for such a maneuver.241 Similarly, even if an injunction says “don’t cut lumber on this property,” a court isn’t going to hold you in contempt for taking down the one rotten tree that’s about to fall on your neighbor’s house. That’s because people understand that rules and injunctions come with the implied catchall “unless you have sufficient justification for departing from the rule” exception.
Try telling that to a robot, though. Machines, unlike at least some humans, lack common sense. They operate according to their instructions—no more, no less. If you mean “don’t cross the double yellow line unless you need to swerve out of the lane to avoid running over a kid” you need to say that. Meanwhile, autonomous vehicles should probably avoid adults too, so better put that in the algorithm. . . . And maybe dogs. . . . And deer and squirrels, too. Or maybe not—crossing into oncoming traffic is dangerous, so while we might do it to avoid hitting a kid even if it raises the risk of a head-on collision, we shouldn’t do it to avoid a squirrel unless the risk of a head-on collision seems low. If you want the self-driving car to do all that, you need to tell it exactly when to swerve and when not to swerve. That’s hard. It’s more plausible to give each outcome weights—killing squirrels is bad, but head-on collisions are much worse, and killing a kid is (Probably? Maybe?) worse still. But then we’re back to deterrence and cost internalization, not injunctions.
Further, even if we can specify the outcome we want with sufficient precision in an injunction, we need to be extremely careful about the permissible means a robot can use to achieve that result. Think back to our example from the Introduction. The drone did exactly what we told it to do. The problem is that we weren’t sufficiently clear in communicating what we wanted it to do. We wanted it to head to the center of the circle without shutting down and without human intervention. But we didn’t say that, because we didn’t anticipate the possibility of the drone doing what it did.242
The “be careful what you wish for” problem is a major one for robotics and AI. Tim Urban of Wait But Why tells the hypothetical story of Turry, a self-learning AI that is designed to mimic handwritten greeting cards.243 If you don’t specify the things it can’t do, or at least impose cost weights, an AI could literally take over all the resources of the world and devote them to producing handwritten greeting cards.244 Computer programmers will, we hope, be aware of this problem and be extremely careful about phrasing their instructions to a robot in just the right way, with precise caveats and limiting conditions to prevent them turning into Skynet or Turry. But judges aren’t computer programmers, and they are unlikely to be as knowledgeable or as careful in what they order robots to do or not do. And even if we could do it, an injunction of this sort represents a pretty significant intrusion into the product design process, something courts have been unwilling to do in other circumstances.245 Whether or not courts are right to shy away from telling companies how to design products generally, we think that’s a good instinct when it comes to robotics, at least in the early stages of the industry.
To issue an effective injunction that causes a robot to do what we want it to do (and nothing else) requires both extreme foresight and extreme precision in drafting it. If injunctions are to work at all, courts will have to spend a lot more time thinking about exactly what they want to happen and all the possible circumstances that could arise. If past experience is any indication, courts are unlikely to do it very well. That’s not a knock on courts. Rather, the problem is twofold: words are notoriously bad at conveying our intended meaning,246 and people are notoriously bad at predicting the future.247 Coders, for their part, aren’t known for their deep understanding of the law, and so we should expect errors in translation even if the injunction is flawlessly written.248 And if we fall into any of these traps, the consequences of drafting the injunction incompletely may be quite severe.
2. “What do you mean you can’t?!”
Courts that nonetheless persist in ordering robots not to do something may run into a second, more surprising problem: it may not be simple or even possible to comply with the injunction. Just as robots don’t have money, they also don’t read and implement court opinions.249 And they aren’t likely to be a party to the case in any event. Enjoining a robot, in other words, really means ordering someone else to implement code that changes the behavior of the robot.
The most likely party to face such an injunction is the owner of the robot. The owner is the one who will likely have been determined to have violated the law, say by using a discriminatory algorithm in a police-profiling decision or operating a self-driving car that has behaved unsafely. But most owners won’t have the technical ability, and perhaps not even the right, to modify the algorithm their robot runs. The most a court could order may be that they ask the vendor who supplied the robot to make the change, or perhaps to take the robot off the market as long as it doesn’t comply with the injunction.250
Even if the developer is a party to the case, perhaps on a design defect theory, the self-learning nature of many modern robots makes simply changing the algorithm more complicated still. A court may, for instance, order the designer of a robot that makes predictions about recidivism for parole boards not to take race into account.251 But that assumes that the robot is simply doing what it was originally programmed to do. That may be less and less common as machine learning proliferates. Ordering a robot to “unlearn” something it has learned through a learning algorithm is much less straightforward than ordering it to include or not include a particular function in its algorithm. Depending on how the robot learns, it might not even be possible.
Life gets easier if courts can control what training information is fed to robots in the first place. At the extremes, a court might order a company to take badly trained robots out of service and to train new ones from scratch. But as the example in the Introduction indicates, the effects of training material on robots are not always predictable. And the results of training are themselves unpredictable, so even controlling the training dataset is no guarantee that a robot, once trained, will behave as the court wants it to.
Further, the future may bring robots that are not only trained in complicated ways but that train themselves in ways we do not understand and cannot replicate. Ordering such a robot to produce or not produce a particular result, or even to consider or not consider a particular factor, may be futile. If we don’t understand how the robot makes decisions, we can’t effectively guide those decisions. It is one thing to look at a transparent algorithm written by programmers and see whether it includes the race of the parolee as a factor. It is quite another to try to untangle whether a robot has learned that race matters by looking at the data and how that learning is implemented in an always-changing algorithm that doesn’t itself explicitly include race. An algorithm that is simply told to minimize the risk of recidivism but not to take race directly into account might end up generating proxies that are correlated with race instead.252 That’s fine if those proxies are in fact the variable of interest. If, say, the fact that members of a minority group commit disproportionately more crimes results from the fact that they are poorer than average, an algorithm that gets to the same result by considering family poverty instead of race may solve the problem.253 But if the algorithm has really just found a proxy for race (say, the street you grew up on in a segregated neighborhood) we aren’t any better off. And it is much harder to tell a robot not to consider race or anything that serves as a proxy for race.254
Courts are used to telling people to do something and having them do it. They may have little patience for the uncertainties of machine learning systems. And they are quite likely to have even less patience with lawyers who tell them their “client” can’t comply with the court’s order.
3. Unintended consequences.
Even when the injunction is simple and clearly identifies who should change the algorithm and how, ordering a robot to change how it “thinks” is likely to have unintended consequences. Consider two examples.
- We don’t want self-driving cars to hit pedestrians. But just brute-forcing that result might lead to other problems, from taking crowded freeways instead of less-crowded surface streets to running into other cars. Some of those consequences could be worse, either because a head-on collision kills more people than running over the pedestrian would or, more likely, because instructing the car to act in a certain way may cause it to avoid a very small chance of killing a pedestrian by avoiding surface streets altogether (even though the collective cost of traffic jams might be quite great). This is a version of the same problem we saw in damages: we need to assign a cost to various outcomes if we want an algorithm to weigh the alternatives. But here the injunction effectively sets the cost as infinite.255 That’s fine if there really is nothing to balance on the other side. But that will rarely be true.
- The case against algorithmic bias seems one of the strongest, and easiest to enjoin, cases.256 And if that bias results simply from a bad training set,257 it may be straightforward to fix. But if an algorithm takes account of a prohibited variable like race, gender, or religion because that variable matters in the data, simply prohibiting consideration of that relevant information can have unanticipated consequences. One possible consequence is that we make the algorithm worse at its job. We might be fine as a society with a certain amount of that in exchange for the moral clarity that comes with not risking discriminating against minorities. But where people are in fact different, insisting on treating them alike can itself be a form of discrimination. Being male, for example, is an extremely strong predictor of criminality. Men commit many more crimes than women,258 and male offenders are much more likely to reoffend.259 We suspect police and judges know this and take it into account, consciously or unconsciously, in their arrest, charging, and sentencing decisions, though they would never say so out loud. But a robot won’t conceal what it’s doing. A court that confronts such a robot is likely to order it not to take gender into account, since doing so seems a rather obvious constitutional violation. But it turns out that if you order pretrial sentencing algorithms to ignore gender entirely, you end up discriminating against women, since they get lumped in with the heightened risks of recidivism that men pose.260
Ordering a robot not to violate the law can lead to additional legal difficulties when injunctions are directed against discrete subsystems within larger robotics systems. These types of injunctions seem likeliest to be granted against newly introduced subsystems within a tried and true application—given that older systems will, by definition, have a longer track record of success. Not only could targeting one component of a larger system change it in unpredictable and often undesirable ways, doing so could also discourage innovation. With the field of AI improving by leaps and bounds, maybe we should be less protective of tried-and-true approaches and more willing to experiment. Even though some of those experiments will fail, the overall arc is likely to bend toward better systems than we have now. But we won’t get there if courts are too quick to shut down new systems while leaving established but imperfect procedures in place. If the alternative to a flawed predictive policing algorithm is the gut instincts of a large number of cops, some of whom are overtly racist and others of whom are subconsciously biased, we might be better off with the robots after all.
III. Rethinking Remedies for Robots
We’ve seen that robots and AI pose a number of challenges to the law of remedies as it is currently applied. In this Part, we offer some preliminary thoughts about how we might redesign the law for the world that is fast approaching. We don’t intend this to be the last word on how to design remedies for robots. Much more remains to be done. Rather, we hope it marks the beginning of a conversation on these issues. The suggestions we outline below will help align the law of remedies with what we know about the behavior of robots.
A. Compensation, Fault, and the Plaintiff’s “Rightful” Position
Compensation is the easiest remedy to translate to robots because its focus is on the (presumably human or corporate) plaintiff. The harm is to plaintiffs, not robots,261 and the same valuation measurement problems arise here that always do in calculating damages. But as we have seen, robot defendants do introduce some complications. Who is responsible when a robot misbehaves? The designer? The manufacturer? The owner? Under current tort law the answer may depend on whether the harm resulted from a design defect, a problem in training, or an error in operation. But learning AIs will blur this line; the designer might not be the one training the AI in ways that caused it to subsequently do harm.
Many (though not all) of the problems with compensating plaintiffs for robot injury come from tort law’s focus on fault as a prerequisite to responsibility. We generally hold entities responsible for accidental injuries only if they act unreasonably.262 And this has the effect of raising the cost of products, activities, or services that cause harm––thereby deterring suboptimal ones. In theory, tort law makes that calculus directly by setting B < PL or demanding some other risk-utility test.263 But in doing so, the law makes a threshold judgment as to whether there should be any liability for costs imposed on others to begin with. At first, this judgment may not seem like a concern of remedies law. After all, remedies kick in only after the legal system has determined who (or what) was to blame. But this threshold question of fault can also function as a de facto limit on remedy allocations. Less common liability regimes, such as strict liability, instead require actors to pay for any harms they cause, “reasonable” or not. That, in turn, shifts the focus of deciding whether B is less than PL to the company that makes the product rather than to the courts––a fact that inevitably impacts what remedies we deem appropriate.
What, for example, does it mean for a robot to behave “unreasonably” or “negligently,” as was recently alleged in an autonomous vehicle accident?264 Tort law’s focus on fault and moral culpability here may make sense where people are concerned. But it is much less meaningful as applied to a robot.265 True, we might want to single out certain design or implementation choices that we think are problematic and discourage them. But in many environments in which robots operate there are more direct regulatory means to do so. The National Highway Traffic Safety Administration (NHTSA), for instance, approves or mandates the introduction of many vehicle safety technologies.266 So, too, does the Federal Aviation Administration (FAA) for aircraft. If we think a particular design shouldn’t be on the market at all, some regulatory bodies will be able to simply prohibit it.267 Indeed, even scholars like Professor Richard Epstein who are no fans of regulation default to regulatory frameworks when it comes to autonomous vehicles.268
Perhaps we just want someone to pay the costs of any harm robots cause, even if the harm occurred without a wrongful or illegal act.269 We often use negligence as a proxy for whether the defendant’s conduct was justified despite the costs it imposes, but there are reasons to think that may be harder to do with robots.270 And maybe we don’t want to ask a jury to decide who was at fault if programmers can actually code in a standard of care that internalizes the harm the robot imposes on others.271
Existing remedies laws might get us there, though not without modification. We do impose strict liability in some circumstances. That’s easier to do when the plaintiff is a passive victim like someone injured by pollution from a factory or from a product that unexpectedly exploded. It’s more problematic when both the plaintiff and the defendant might have contributed to the cause of the injury. When two cars collide, one reason we try to decide who was at fault (or whether both were in part) is to fairly allocate the cost of injury to the party who was best positioned to avoid it. Allocating that fault will raise new questions when a robot-driven car gets into an accident because its driving capabilities and the sorts of evidence it can provide will be different than human drivers. We can’t cross-examine the robot to interrogate its state of mind.272 On the other hand, autonomous vehicles are likely to record every aspect of the accident, giving us a better record than fallible human memory currently does. A second reason we focus on blame is that we need to worry that the parties might lie about what happened. But self-driving cars are likely to keep clear records and video that may make it easier to figure out what happened.273 And it may make less sense to try to assess fault when two robotic cars collide, though we expect that will be a much rarer occurrence.274
Yet another reason we assess fault against people is that blame for wrongdoing can encourage more careful behavior. As we discussed in Part II, that isn’t likely to work, or at least to work in the same way, with robots. Without the element of moral culpability that underlies much remedies law, we might need to consider new means of internalizing the costs robots impose on society rather than hoping that our existing legal rules will produce the same moral or behavioral effects that they currently do with humans. As robots and AI take on more responsibility in our society, the law should move away from efforts to assess moral culpability and toward a system that internalizes the costs those machines impose on those around them. Doing so will make the problem of coding effective care easier. And it may increasingly mean tort cases involving robots don’t show up in the legal system at all, but in some sort of regulatory compensation system or even a more general social insurance network.275
While we could assess the overall safety of an autonomous vehicle and—assuming it was safer than the human standard—deny liability altogether in crashes,276 we think that depriving injured parties of any remedy might not make sense. Another straightforward way to train autonomous vehicles to avoid doing unnecessary harm is to make them responsible for the harm they cause whether or not they were “negligent.” But we may only want regulatory standards that reduce the harm in circumstances in which it is avoidable, just as we have taken steps to mitigate the damage from manufacturing defects using similar incentives.277
That doesn’t solve all problems with autonomous vehicles, particularly when they interact with humans, because we still must decide when an autonomous vehicle “causes” an accident with a human driver. While occasional fatal crashes have dominated the headlines, most autonomous vehicle-human car accidents involve humans running into autonomous vehicles, often because the autonomous vehicle did something legal and presumably safe but unexpected, like driving the speed limit or coming to a complete stop at an intersection.278 While that may suggest that we want to program autonomous vehicles to behave in a more predictable way, or even impose strict liability on the activity, it’s hard to fault the autonomous vehicle for being rear-ended because it came to a complete stop at an intersection. Without the addition of a contributory negligence defense (which functions a lot like plain old B < PL from a fault perspective), innovators would end up disproportionately bearing costs, human drivers wouldn’t be priced off the roads as quickly as they should, and companies would also be apt to spend less on safety from a competitive perspective, since no amount of investment could get them off the liability hook when people, themselves, created the hazards.279
Thus, while we think moral fault makes little sense in accidents involving autonomous vehicles, and perhaps any consideration of blame is problematic when considering accidents between two autonomous vehicles,280 we will still need to compare the behavior of humans and autonomous vehicles in order to make sure that we give proper incentives to human drivers. Comparative negligence may still matter for robot drivers, therefore. And negligence rules that rely on inference, such as res ipsa loquitur, may be particularly useful in aiding these so-called “fault” determinations.281 But it is the idealized cost-internalization vision of negligence reflected in Judge Learned Hand’s formula—not consciousness of fault or state of mind—that we should care about.
Finally, we want to give robots (or their makers) appropriate incentives to improve over time. Traditional tort law doesn’t necessarily encourage people to improve. The standard of negligence is based on the behavior of other people, which by nature remains relatively static over time. That standard might get higher if norms change; antilock braking systems on cars were once a novelty but are now standard, so failing to include them would probably give rise to liability today. But the standard might also get lower. Texting while driving should be strong evidence of negligence, but if it becomes common enough, that might change, with juries treating it just as they do operating a radio in a car today—a distraction, but a normal one.
But the law has an opportunity to push robots to improve. Robots don’t seem to be good targets for rules based on moral blame or state of mind, but they are good at data. So we might consider a legal standard that bases liability on how safe the robot is compared to others of its type—a sort of “robotic reasonableness” test.282 That could take the form of a carrot, such as a safe harbor for self-driving cars that are significantly safer than average (or significantly safer than human drivers). Or we could use a stick, holding robots liable if they lag behind their peers or even shutting down the worst 10 percent of robots in a category every year.283
B. Punishment, Deterrence, and the Human Id
Deterrence, unlike compensation, is forward-looking. We want robots to internalize the costs of their actions even apart from compensation of particular victims. The good news is that cost internalization has the potential to work better with robots than it does with people.284 Robot algorithms may allow us to internalize costs further down the causal chain than tort law normally does, for example, by accounting for the social cost of pollution or other nebulous injuries to society as a whole. But these injuries must be priced, again requiring fraught social tradeoffs to be made explicit. And the pricing should be cost based. We should minimize the psychologically-driven aspects of deterrence (jail, disgorgement of ill-gotten gains) and replace them with more rational measures of cost.
Doing so is at odds with many of the mechanisms we have for deterrence, however. Often those mechanisms are directed at showing moral opprobrium or at punishing people in ways we expect them to react to psychologically. Professor Mulligan’s idea of punching robots who wrong us285 sounds silly, but there is a serious idea behind it. Much of our law of remedies, including our search for fault (but also the way in which we punish), is designed not to compensate plaintiffs or even to internalize costs for defendants but to make us feel better. This sometimes involves “sending a message,” but often the defendant isn’t the target of the message. Perhaps it is society as a whole; large punitive damage awards or harsh criminal penalties can signal the things we won’t tolerate as a society, and overly lenient sentences can do the opposite. That is a broader social conversation, albeit one usually carried out in the context of legal remedies.286 But often, remedies are purely cathartic: we want someone to blame to make ourselves feel better for the bad thing that happened to us. When there is no obvious candidate for blame, we go to considerable lengths to find one.287 Punishment in this sense is a form of psychological compensation—the very act of punishing the defendant is the compensation.
This seems socially wasteful. Punishing robots, not to make them behave better but just to punish them, is kind of like kicking a puppy that can’t understand why it’s being hurt. The same might be true of punishing people to make us feel better, but with robots the punishment is stripped of any pretense that it is sending a message to make the robot understand the wrongness of its actions.
We don’t deny that there is a real phenomenon at work here, or even that it may benefit the victim psychologically. But it might not make sense to serve those goals when suing robots. Is there a way to make us stop? To channel that instinct into other areas than the legal system where it might be more productive? Should we just abandon the signaling function of remedies altogether? Perhaps, but we probably won’t, human nature being what it is.
Rather, if we want to rationalize remedies for robots, we might need to take human decision-makers (especially untrained ones like juries) out of the remedies equation in some cases (or at least closely constrain the remedies they can order and the reasons that justify those remedies).288 Juries are likely to have an instinct to punish bad behavior by robots. But punishment makes sense only if we think compensation for damages is inadequate and so defendants will take insufficient precautions or engage in socially harmful behavior that we want them to stop.289 A robot that calculates the cost of its various decisions accurately will make bad decisions if we add in data on the likelihood of punitive damages that exceed those costs. And if the robot is being punished precisely because it is calculating how many people it’s ok to kill,290 the problem becomes recursive and we will undo the purpose of optimal deterrence and cost internalization.
C. Reeducating Robots
Injunctions, as we have seen, are both important and problematic remedies for robots. Can courts order a robot to change its programming? Perhaps we can require changes in design, or we might compel some sorts of modifications to learning algorithms.
Courts in general favor injunctions that preserve the status quo and prohibit parties from changing things (so-called prohibitory injunctions). They are traditionally more reluctant to order parties to do affirmative things to change the state of affairs (mandatory injunctions).291 It does happen, particularly in impact litigation after a final finding of liability. But courts tend to shy away from involving themselves in the details of running a business or designing a product if they can avoid it.292 With robots, though, there’s no avoiding it—whether the injunction is mandatory or prohibitory. An order for a robot to do something and an order for it to not do something both require redesigning the product. Courts should take care when and how they grant those injunctions.
In light of this reality, what exactly will courts order robots to do? Rather than ordering the code to be written in a specific way, one likely compromise is to order the company to find a way to achieve a specific result. As we saw in Part II, that by no means solves the problems with injunctions against robots. And courts cannot simply order a defendant to obey the law.293 But it does offer some flexibility to the company that needs to rewrite their code, ideally without introducing other problems in the process.
One way to increase that flexibility is to give companies time to comply. Courts generally expect their orders to be obeyed quickly. But writing quick code often means writing bad code, particularly in an ever-changing, complex machine learning system. Courts and regulators should be patient. Self-driving cars go through years of testing before we are comfortable that they will drive safely. We shouldn’t just rewrite that code and put it on the streets without testing. So courts should delay implementation of their orders against robots to enable the defendant to develop and test a solution that doesn’t cause more problems than it solves. Regulators have so far shown admirable restraint in not rushing to mandate particular rules for autonomous vehicles.294 That restraint should extend to other sorts of robots as well.295
Turning that results-oriented goal into an injunction runs into legal problems, though. Obviously we don’t want cars to run over kids, but a judge can’t simply order that. Court orders can’t just say “obey the law”;296 they must give clear notice of what the defendant must do. So an injunction might say “stop the car if the likelihood that a pedestrian will imminently enter the intersection is greater than 0.2 percent.”
In some cases, orders might require robots to make their algorithms perform less well. An injunction preventing the police from taking gender into account in predicting criminality may make it harder to predict who will commit crimes. We might nonetheless want to order it, either to counteract existing bias reflected in the training data or simply because recognizing gender differences in criminality violates a constitutional norm even if the differences are real. But in doing so we are departing from the real world, ordering companies to train their robots to make decisions based on the society we would like to have rather than the one we actually have.
The history of structural injunctions may offer useful lessons here. Courts that have tried to solve systemic problems in complex human systems like prisons or school districts have struggled with how to order changes to those systems. Those injunctions have often gotten more specific over time, requiring specific payments or very specific rules as general orders have failed to achieve the desired results.297 They have also involved the appointment of special masters to oversee the operation of the institution on an ongoing basis.298 We can imagine something similar happening as judges try to tweak an algorithm from the bench. But those injunctions are also viewed as among the most problematic,299 so courts may not want to emulate them.
One compensating advantage to robot injunctions is that the orders involve rewriting code, and in a connected world these changes can often be shipped out retroactively. Tesla updates the software periodically in cars it has already sold. Unlike traditional products, in which an injunction is generally limited to the sale of products in the future, court orders against robots can affect existing robots already in the hands of consumers.300 That makes the injunction much more effective, though it also may raise due process concerns on the part of owners not a party to the case whose robot suddenly behaves differently or stops working altogether.301
D. The Robot Death Penalty?
The fact that a robot, not a person, is the defendant does open up some possible new remedies. Remedies law governs civil wrongs; we have a different and stricter set of rules for criminal cases, one that is outside the scope of this paper.302 We have those stricter standards because we worry about the consequences of depriving people of liberty even when they have done something wrong. We worry even more about depriving them of life. It is an adage that we put a heavy thumb on the scale in favor of innocence, allowing the guilty to go free before punishing the innocent.303 We require guilt to be proven beyond a reasonable doubt, and we have special protections before imposing the death penalty.304 Some states and most countries have in fact abolished the death penalty altogether.
But robots aren’t people, and we might worry less about robot liberty.305 True, robots will be entitled to due process, if for no other reason than that they are owned by people or companies that would lose valuable property if their robots disappeared. But one new and significant form of remedy becomes available against robots that isn’t available against people in most circumstances: the robot death penalty. If a robot is causing unjustified harm and we can’t stop it, either because we don’t understand how it works or because the harm is inextricably bound up with its programming, we might simply shut it down.306 Turning off malfunctioning robots is a simple and effective, if blunt, instrument to enforce an injunction. And removing the robot from commercial deployment may allow us to figure out what went wrong by engaging in the sorts of testing we couldn’t do without jeopardizing operational function.
Should we shut down misbehaving robots? In some cases, the answer is yes. Corporations do it all the time.307 A court has ordered a robot beheaded.308 And essentially any time you change the code you are changing the robot by replacing it with a new and (hopefully) improved one.
Whether courts can order a robot shut down over the objections of its owner is a slightly harder question, but the answer is still probably yes. Courts order the killing of pets that repeatedly attack others and can order other types of machines shut down if they are unreasonably dangerous.309 If a robot can be replaced by others with competing algorithms, we probably want to shut it down if it is operating below the standard of care. One way learning algorithms improve is through natural selection,310 and shutting down the bad ones is just a form of that process. But if an AI has developed unique attributes as a result of its own learning, we have the problem of “dual-use” technologies.311 A self-learning AI may behave differently in both good and bad ways, and those differences may be related. The robot death penalty kills off the good as well as the bad, so we want to do it only if we think the harm the robot is causing is sufficiently great and the unique benefit of its approach sufficiently low that the cost of losing the benefit is worth it.
For this reason, the use of the robot death penalty should probably be rare.312 Shutting down a robot, especially a self-learning one, means shutting down an avenue of innovation.313 We should do that only if there is strong evidence that the AI does more harm than good and that there isn’t a less intrusive way to solve the problem. Just as courts should be reluctant to tell robots to change how they behave, they should be reluctant to turn the robots off altogether.314
Further, the robot death penalty presents more serious due process issues with respect to the existing stock of robots in the hands of people other than the defendant. Courts generally can’t reach out and take away property in the hands of nonparties without due process, even if those products cause problems and even if the court can order the company to stop selling new copies of the product. But the malleability of software presents some grey areas here. It’s okay to order a defendant to push out changes to the product, though it’s an easier case if the recipient has the choice of whether to accept those changes.315 The company can probably stop supporting the product remotely. But a software “upgrade” that is really just an effort to “brick” an existing product seems a reach too far.316
Finally, there is the possibility that the law will recognize robots as sentient entities with their own rights.317 That isn’t as far-fetched as it sounds. Corporations aren’t people either, but they get legal rights (in some instances more rights than people).318 Animals also have some rights, though fewer than humans or corporations.319 And some countries have already recognized rights for robots.320
Charles Stross has called corporations the first AIs.321 Like AIs, corporations are created by people, designed to serve ends dictated by people, but over time come to serve their own purposes.322 And some have operationalized that connection, pointing out that “anyone can confer legal personhood on an autonomous computer algorithm by putting it in control of a limited liability company.”323 It’s not impossible that in the future we will extend at least some legal rights to robots as well, particularly unique robots with learned behavior. And one of those rights may well be the right not to be shut down without due process.324
E. What Robots Can Teach Us about Remedies for Humans
Robots present a number of challenges to courts imposing remedies on robotic and AI defendants. Working through those challenges is valuable and important in its own right. But doing so can also teach us some things about the law of remedies as it currently applies to people and corporations.
First, much of remedies, like much of law, is preoccupied with fault—identifying wrongdoers and treating them differently. There may be good reasons for that, both within the legal system and in society as a whole. But it works better in some types of cases than in others. Our preoccupation with blame motivates many remedies, particularly monetary equitable relief. This preoccupation distorts damage awards, particularly when something really bad happens and there is not an obvious culprit. It also applies poorly to corporations, which don’t really have a unitary purpose in the way a person might.325 It’s also costly, requiring us to assess blame in traffic cases that could otherwise be resolved more easily if we didn’t have to evaluate witness credibility. A fault-based legal system doesn’t work particularly well in a world of robots. But perhaps the problem is bigger than that: it might not work well in a world of multinational corporations either.326 We should look for opportunities to avoid deciding fault, particularly when human behavior is not the primary issue in a legal case.327
A second lesson is the extent to which our legal remedies, while nominally about compensation, actually serve other purposes, particularly retribution. We described remedies law at the outset of the paper as being about “what you get when you win.” But decades of personal experience litigating cases328 have reinforced the important lesson that what plaintiffs want is quite often something the legal system isn’t prepared to give. They may want to be heard, they may want justice to be done, or they may want to send a message to the defendant or to others. Often what they want—closure, or for the wrong to be undone—is something the system not only can’t give them, but that the process of a lawsuit actually makes worse. The disconnect between what plaintiffs want and what the law can give them skews remedies law in various ways. Some do no harm: awards of nominal damages or injunctions that vindicate a position while not really changing the status quo. But we often do the legal equivalent of punching robots—punishing people to make ourselves feel better, even as we frequently deny compensation for real injuries. It’s just that it’s easier to see when it’s a robot you’re punching.
A final lesson is that our legal system sweeps some hard problems under the rug. We don’t tell the world how much a human life is worth. We make judgments on that issue every day, but we do them haphazardly and indirectly, often while denying we are doing any such thing. We make compromises and bargains in the jury room, awarding damages that don’t reflect the actual injury the law is intended to redress but some other, perhaps impermissible consideration.329 And we make judgments about people and situations in- and outside of court without articulating a reason for it, and often in circumstances in which we either couldn’t articulate that decision-making process or in which doing so would make it clear we were violating the law. We swerve our car on reflex or instinct, sometimes avoiding danger but sometimes making things worse. We don’t do that because of a rational cost-benefit calculus, but in a split-second judgment based on imperfect information. Police decide whether to stop a car, and judges whether to grant bail, based on experience, instinct, and bias as much as on cold, hard data.
Robots expose those hidden aspects of our legal system and our society. A robot can’t make an instinctive judgment about the value of a human life, or about the safety of swerving to avoid a squirrel, or about the likelihood of female convicts reoffending compared to their male counterparts. If robots have to make those decisions—and they will, just as people do—they will have to show their work. And showing that work will, at times, expose the tolerances and affordances our legal system currently ignores. That might be a good thing, ferreting out our racism, unequal treatment, and sloppy economic thinking in the valuation of life and property. Or it might be a bad thing, particularly if we have to confront our failings but can’t actually do away with them. It’s probably both. But whatever one thinks about it, robots make explicit many decisions our legal system and our society have long decided not to think or talk about. For that, if nothing else, remedies for robots deserve serious attention.
Conclusion
Robots and AI systems will do bad things. When they do, our legal system will step in to try to make things right. But how it does so matters. Our remedies rules, unsurprisingly, aren’t written with robots in mind. Adapting our existing rules to deal with the technology will require a nuanced understanding of the different ways robots and humans respond to legal rules. As we have shown, failing to recognize those differences could result in significant unintended consequences—inadvertently encouraging the wrong behaviors, or even rendering our most important remedial mechanisms functionally irrelevant.
Robotics will require some fundamental rethinking of what remedies we award and why. That rethinking, in turn, will expose a host of fraught legal and ethical issues that affect not just robots but people, too. Indeed, one of the most pressing challenges raised by the technology is its tendency to reveal the tradeoffs between societal, economic, and legal values that many of us, today, make without deeply appreciating the downstream consequences. In a coming age where robots play an increasing role in human lives, ensuring that our remedies rules both account for these consequences and incentivize the right ones will require care and imagination. We need a law of remedies for robots. But in the final analysis, remedies for robots may also end up being remedies for all of us.
- 1This example comes from a presentation at the 11th Annual Stanford Ecommerce Best Practices Conference in June 2014. As far as we know, it has not been previously described in print.
- 2See generally Solon Barocas and Andrew D. Selbst, Big Data’s Disparate Impact, 104 Cal L Rev 671 (2016); Jack M. Balkin, The Path of Robotics Law, 6 Cal L Rev Cir 45 (2015); Ryan Calo, Robotics and the Lessons of Cyberlaw, 103 Cal L Rev 513 (2015); Harry Surden, Machine Learning and Law, 89 Wash L Rev 87 (2014); Kate Darling, Extending Legal Protection to Social Robots: The Effects of Anthropomorphism, Empathy, and Violent Behavior towards Robotic Objects in Robot Law, in Ryan Calo, A. Michael Froomkin, and Ian Kerr, eds, Robot Law 213 (Edward Elgar 2016); Kate Crawford and Jason Schultz, Big Data and Due Process: Toward a Framework to Redress Predictive Privacy Harms, 55 BC L Rev 93 (2014); Ryan Abbott, I Think, Therefore I Invent: Creative Computers and the Future of Patent Law, 57 BC L Rev 1079 (2016); Bryan Casey, Amoral Machines, or: How Roboticists Can Learn to Stop Worrying and Love the Law, 111 Nw U L Rev 1347 (2017).
- 3See Part II.E.
- 4See notes 89–95 and accompanying text.
- 5See Part III.D.
- 6Christina Mulligan, Revenge against Robots, 69 SC L Rev 579, 588–89 (2018) (“If it turns out that punishing robots provides the right kind of psychological benefit to humans following an injury, we should punish robots.”).
- 7197 F3d 1284 (9th Cir 1999).
- 8Id at 1285 (Kozinski dissenting from denial of petition for rehearing en banc). The zeitgeist captured by Judge Kozinski’s opening line was first noted by Ryan Calo, Robots in American Law *2 (University of Washington School of Law Research Paper No 2016-04, Feb 2016), archived at http://perma.cc/3YD6-XNQV.
- 9Wendt v Host International, Inc, 125 F3d 806, 809 (9th Cir 1997).
- 10The case referred to here is White v Samsung Electronics America, Inc, 971 F2d 1395, 1396–97 (9th Cir 1992), which involved an animatronic version of Vanna White, a television game show persona.
- 11See Calo, Robots in American Law at *3 (cited in note 8), citing World Intellectual Property Organization, World Intellectual Property Report: Breakthrough Innovation and Economic Growth *120–35 (2015), archived at http://perma.cc/FC4D-CX6W (noting surge in IP activity for robots).
- 12Consider, for example, Transcript of Mark Zuckerberg’s Senate Hearing (Wash Post, Apr 10, 2018), online at http://www.washingtonpost.com/news/the-switch/wp/2018/04/10/transcript-of-mark-zuckerbergs-senate-hearing (visited Apr 16, 2019) (Perma archive unavailable).
- 13See, for example, Waymo, Waymo Safety Report: On the Road to Fully Self-Driving *3 (2018), archived at http://perma.cc/LQ64-UJT9.
- 14See notes 38–39 and accompanying text.
- 15See, for example, Robotic Surgery Center, What is Robotic Surgery? (NYU Langone Health), archived at http://perma.cc/88QA-RQRQ.
- 16See Gill A. Pratt, Is a Cambrian Explosion Coming for Robotics?, 29 J Econ Persp 51, 51 (2015) (“Today, technological developments on several fronts are fomenting . . . [an] explosion in the diversification and applicability of robotics.”).
- 17And not just because they sometimes escape. See Complaint and Jury Demand, Holbrook v Prodomax Automation, Ltd, No 1:17-cv-00219, *3 (WD Mich filed Mar 7, 2017) (Holbrook Complaint) (wrongful death suit alleging a robot escaped from its work area at a Michigan auto parts factory and killed a woman).
- 18See notes 66–72 and accompanying text.
- 19Or, as Jonathan Zittrain might describe, “autonomish” robots. See Jonathan L. Zittrain, What Yesterday’s Copyright Wars Teach Us about Today’s Issues in AI, delivered as the David L. Lange Lecture in Intellectual Property Law at Harvard Law School (2018), transcript archived at http://perma.cc/TZP7-H4EH.
- 20See, for example, Daisuke Wakabayashi, Self-Driving Uber Car Kills Pedestrian in Arizona, Where Robots Roam (NY Times, Mar 19, 2018), archived at http://perma.cc/N88D-SBX9; Holbrook Complaint at *3 (cited in note 17).
- 21See, for example, Rebecca Crootof, War Torts: Accountability for Autonomous Weapons, 164 U Pa L Rev 1347, 1389–1402 (2016) (discussing robotic weapons systems and their potential legal liability).
- 22See, for example, Sam Corbett-Davies, Sharad Goel, and Sandra González-Bailón, Even Imperfect Algorithms Can Improve the Criminal Justice System (NY Times, Dec 20, 2017), archived at http://perma.cc/Z55B-DK22.
- 23See, for example, James Vincent, Twitter Taught Microsoft’s AI Chatbot to Be a Racist Asshole in Less Than a Day (The Verge, Mar 24, 2016), archived at http://perma.cc/8B3G-LWEC.
- 24See, for example, Charles Duhigg, The Case against Google (NY Times, Feb 20, 2018), archived at http://perma.cc/64Y7-3NAU (noting that prominent lawmakers and critics “have accused Google of creating an automated advertising system so vast and subtle that hardly anyone noticed when Russian saboteurs co-opted it in the last election”).
- 25See Andrew Berg, Edward F. Buffie, and Luis-Felipe Zanna, Should We Fear the Robot Revolution? (The Correct Answer Is Yes) *4 (International Monetary Fund Working Paper No 18-116, May 21, 2018), archived at http://perma.cc/TBM9-RSLC (arguing that global society is on the cusp of a second industrial revolution thanks to advances in robotics and artificial intelligence).
- 26See note 143.
- 27See id.
- 28See Oliver Morton, Immigrants from the Future (The Economist, May 27, 2014), archived at http://perma.cc/Y59U-WB2Y (noting Karl Capek’s coinage of the term in his 1920s play titled R.U.R.: Rossumovi Univerzální Roboti).
- 29Indeed, the problem may be intractable, as we argue elsewhere. See Bryan Casey and Mark A. Lemley, You Might Be a Robot, 105 Cornell L Rev *18–28 (forthcoming 2019), archived at http://perma.cc/L3B6-7Y6A.
- 30See Calo, 103 Cal L Rev at 529–32 (cited in note 2).
- 31See id at 514 (discussing the “essential qualities” of the Internet and the emergence of “cyberlaw” in the mid-1990s).
- 32Id at 534 (describing “embodiment” as the “capacity to act physically upon the world [and], in turn, to the potential to physically harm people or property”).
- 33Id at 538 (describing “emergence” as the ability to “do more than merely repeat instructions but adapt to circumstance”).
- 34Calo, 103 Cal L Rev at 545–49 (cited in note 2) (describing “social valence” as the heightened emotional response triggered in humans by our tendency to anthropomorphize robots).
- 35Id at 515.
- 36Id at 531.
- 37Id.
- 38Cade Metz, In a Huge Breakthrough, Google’s AI Beats a Top Player at the Game of Go (Wired, Jan 27, 2016), archived at http://perma.cc/KB42-9TGC. “Go” is an ancient strategy game that is comparable to chess, though far more computationally complex. Id.
- 39Tom Simonite, Can Bots Outwit Humans in One of the Biggest Esports Games? (Wired, June 25, 2018), online at http://www.wired.com/story/can-bots-outwit-humans-in-one-of-the-biggest-esports-games (visited Apr 24, 2019) (Perma archive unavailable). DotA is one of the internet’s most popular real-time strategy games and is more difficult for AI systems than Go or chess. Id.
- 40See Balkin, 6 Cal L Rev Cir at 47 (2015) (cited in note 2).
- 41Id.
- 42See notes 66–69 and accompanying text. See also, for example, Balkin, 6 Cal L Rev Cir at 45 (cited in note 2) (stating he does “not think it is helpful to speak in terms of ‘essential qualities’ of a new technology that we can then apply to law”).
- 43Our broad reading of “robot” also extends to regression-based predictive systems such as the headline-grabbing “COMPAS” tool for predicting criminal behavior. See Julia Angwin, et al, Machine Bias (ProPublica, May 23, 2016), archived at http://perma.cc/GE6Q-7GQY (detailing COMPAS’s role as a criminal risk assessment tool). We include these systems—which could also be described as mere statistical tools—in our definition of “robot” with some hesitation. But the fact remains that such systems are now routinely anthropomorphized by academics and media outlets alike as “AI.” See, for example, Anupam Chander, The Racist Algorithm?, 115 Mich L Rev 1023, 1025 (2017) (“[O]ur prescription to the problem of racist or sexist algorithms is algorithmic affirmative action.”) (citations omitted); Christian Sandvig, et al, When the Algorithm Itself Is a Racist: Diagnosing Ethical Harm in the Basic Components of Software, 10 Intl J Communication 4972, 4975 (2016) (“Since these [algorithms] require an explicit definition of skin and non-skin colors in advance, it logically follows they could certainly be racist.”); James Zou and Londa Schiebinger, Design AI So That It’s Fair, 559 Nature 324, 324 (2018). We believe including them in our discussion of robots is appropriate. As we note elsewhere, however, this is one example of the definitional problems that beset the field. See Casey and Lemley, 105 Cornell L Rev at *18–28 (cited in note 29).
- 44See Kamal Ahmed, Google’s Demis Hassabis—Misuse of Artificial Intelligence “Could Do Harm’’ (BBC News, Sept 16, 2015), archived at http://perma.cc/7PNC-XXAB. While some scholars have suggested that “there is a continuum between ‘robots’ and ‘artificial intelligence,’” Balkin, 6 Cal L Rev Cir at 50 (cited in note 2), the distinction is actually artificial (if you’ll pardon the expression). Without the ability to exhibit intelligent behavior, any so-called robot would be little more than an inanimate composite of metal, plastic, or bits. Accordingly, AI is better understood as a component feature of any robotics system, rather than an entity separate from it.
- 45That doesn’t mean we think this definition of a robot will suffice. To the contrary, we suggest elsewhere that it is as flawed as other definitions. See Casey and Lemley, 105 Cornell L Rev at *18–28 (cited in note 29).
- 46See Pedro Domingos, The Master Algorithm: How the Quest for the Ultimate Learning Machine Will Remake Our World 1–22 (Basic Books 2015).
- 47Id at 1.
- 48Id at 1–2.
- 49See id at 6–8.
- 50See David Auerbach, The Programs That Become the Programmers (Slate, Sept 25, 2015), archived at http://perma.cc/72AJ-Y9YN.
- 51This example derives from David Auerbach’s piece. See id.
- 52The latter example refers to IBM Deep Blue’s defeat of the world chess champion, Garry Kasparov, in 1997, which was accomplished using a brute-force GOFAI approach. See Matt McFarland, Google Just Mastered a Game That Vexed Scientists—and Their Machines—for Decades (Wash Post, Jan 27, 2016), online at http://www.washingtonpost.com/news/innovations/wp/2016/01/27/google-just-mastered-a-game-thats-vexed-scientists-for-decades (visited Apr 16, 2019) (Perma archive unavailable).
- 53In many instances, programmers can teach their robots how to handle these types of “edge cases” by prospectively encoding fail-safe measures that anticipate them. But even robust GOFAI approaches that account for a wide array of edge cases are often no match for amorphous and ambiguous real-world environments.
- 54Ajay Agrawal, Joshua Gans, and Avi Goldfarb, The Simple Economics of Machine Intelligence (Harvard Business Review, Nov 17, 2016), archived at http://perma.cc/V9VW-ZDSF.
- 55See id.
- 56Domingos, The Master Algorithm at 89–90 (cited in note 46).
- 57See id.
- 58See Ray Kurzweil, The Singularity Is Near: When Humans Transcend Biology 289 (Viking 2005) (writing “the AI winter is long since over”).
- 59Robots, of course, receive a considerable amount of help throughout this process. Like children with parents hovering over them, most machines require that designers first label, categorize, or “featurize” input data before the machines begin guessing. See Andrew D. Selbst, Disparate Impact in Big Data Policing, 52 Ga L Rev 109, 131–35 (2017); David Lehr and Paul Ohm, Playing with the Data: What Legal Scholars Should Learn about Machine Learning, 51 UC Davis L Rev 653, 672–77 (2017) (offering a detailed description of the various parts of machine learning). The subset of machine learning known as “unsupervised” or “semi-supervised” learning attempts to minimize human intervention in this regard.
- 60And when it performs poorly, vice versa.
- 61See Domingos, The Master Algorithm at 6 (cited in note 46).
- 62See id at 89–90.
- 63Corbin Barthold, Artificial Intelligence Will Benefit Us Immensely—If We Don’t Get in the Way (Forbes, Dec 4, 2018), archived at http://perma.cc/8CPE-X5BE.
- 64Iain M. Cockburn, Rebecca Henderson, and Scott Stern, The Impact of Artificial Intelligence on Innovation: An Exploratory Analysis *5, NBER Conference on Research Issues in Artificial Intelligence (unpublished manuscript, Dec 16, 2017), archived at http://perma.cc/D53V-C3RD.
- 65Id. See generally Paul A. David, The Dynamo and the Computer: An Historical Perspective on the Modern Productivity Paradox, 80 Am Econ Rev 355 (1990).
- 66Bryan Casey, Ashkon Farhangi, and Roland Vogl, Rethinking Explainable Machines: The GDPR’s “Right to Explanation” Debate and the Rise of Algorithmic Audits in Enterprise, 34 Berkeley Tech L J 143, 149 (2019).
- 67See Mick Mountz, Kiva the Disrupter (Harvard Business Review, Dec 2012), archived at http://perma.cc/6FSY-ZY35.
- 68See Rebecca Crootof, The Internet of Torts, 69 Duke L J *3–10 (forthcoming 2019), archived at http://perma.cc/8TGY-J9QC.
- 69See Domingos, The Master Algorithm at 258–59 (cited in note 46).
- 70See, for example, Alexis C. Madrigal, The Most Important Self-Driving Car Announcement Yet (The Atlantic, Mar 28, 2018), archived at http://perma.cc/D8K5-YD8U (reporting that autonomous driving will be implemented “at scale” within “two years or less” and “that [Waymo’s] fleet [of 20,000 cars] alone will be capable of doing a million trips each day in 2020”); Heather Somerville, Uber, Transitioning to Fleet Operator, Orders 24,000 Driverless Cars from Volvo (Insurance Journal, Nov 21, 2017), archived at http://perma.cc/YY5E-NQMJ.
- 71See, for example, Emily Mullin, FDA Approves AI-Powered Diagnostic That Doesn’t Need a Doctor’s Help (MIT Technology Review, Apr 11, 2018), archived at http://perma.cc/6QZ2-VPCW.
- 72See, for example, Bernard Marr, 6 Amazing Passenger Drone Projects Everyone Should Know About (Forbes, Mar 26, 2018), archived at http://perma.cc/A7X7-STWU.
- 73Robotic cars, aircraft, and manufacturing systems have killed and maimed third parties. See, for example, Wakabayashi, Self-Driving Uber Car Kills Pedestrian (cited in note 20); Peter Holley, After Crash, Injured Motorcyclist Accuses Robot-Driven Vehicle of “Negligent Driving” (Wash Post, Jan 25, 2018), online at http://www.washingtonpost.com/news/innovations/wp/2018/01/25/after-crash-injured-motorcyclist-accuses-robot-driven-vehicle-of-negligent-driving (visited Apr 16, 2019) (Perma archive unavailable); Julie A. Steinberg, “Killer Robot” Suit Awaits Ruling on Japanese Maker (Bloomberg Environment, May 24, 2018), online at http://news.bloombergenvironment.com/safety/killer-robot-suit-awaits-ruling-on-japanese-maker (visited Apr 16, 2019) (Perma archive unavailable). Robots tasked with making online purchases have been “arrested” for illicitly buying narcotics on the dark web. See Arjun Kharpal, Robot with $100 Bitcoin Buys Drugs, Gets Arrested (CNBC, Apr 21, 2015), archived at http://perma.cc/JZ5W-KFVZ. And robots powering our largest social media platforms have even influenced the course of national elections. See Duhigg, The Case against Google (cited in note 24).
- 74See Restatement (Second) of Torts § 520. See generally Joseph A. Page, Liability for Unreasonably and Unavoidably Unsafe Products: Does Negligence Doctrine Have a Role to Play?, 72 Chi Kent L Rev 87 (1996); James A. Henderson Jr and Aaron D. Twerski, Closing the American Products Liability Frontier: The Rejection of Liability without Defect, 66 NYU L Rev 1263 (1991); Harvey M. Grossman, Categorical Liability: Why the Gates Should Be Kept Closed, 36 S Tex L Rev 385 (1995); Frank H. McCarthy, Products Liability—Doctrine of Unavoidably Unsafe Products Applied to Manufacturer of Polio Vaccine, 11 Tulsa L J 296 (1975).
- 75See Welge v Planters Lifesavers Co, 17 F3d 209, 210 (7th Cir 1994).
- 76See Robert W. Peterson, New Technology—Old Law: Autonomous Vehicles and California’s Insurance Framework, 52 Santa Clara L Rev 1341, 1355 (2012) (“However safer [robots] may be, they will still be dangerous and will spin off injuries.”).
- 77See Soo Youn, 24 Amazon Workers Sent to Hospital After Robot Accidentally Unleashes Bear Spray (ABC News, Dec 6, 2018), online at http://abcnews.go.com/US/24-amazon-workers-hospital-bear-repellent-accident/story?id=59625712 (visited May 4, 2019) (Perma archive unavailable).
- 78Mark R. Rosekind, Remarks: Autonomous Car Detroit Conference (National Highway Traffic Safety Administration, Mar 16, 2016), archived at http://perma.cc/6DLL-NC6T. See also, for example, Jensen v American Suzuki Motor Corp, 35 P3d 776, 779 (Idaho 2001) (noting that underlying the doctrine of “crashworthiness” is the assumption that not all accidents are avoidable); Skeie v Mercer Trucking Co, Inc, 61 P3d 1207, 1210 (Wash App 2003) (“[C]ourts recognize that it is reasonably foreseeable, even statistically inevitable, that vehicles will be involved in collisions.”).
- 79Today’s human-driven car accidents can cause unavoidable injuries to drivers, passengers, bystanders, and property. But there is an important difference between contemporary cars and the robocars of the future. Injury from a car crash today is typically the result either of the design of the car or, far more commonly, the behavior of the humans. The law distinguishes those two types of harm, holding manufacturers responsible for injuries caused by product design and human drivers responsible for the injuries they cause. But self-driving cars, as the name implies, drive themselves. The “design” of the product, in other words, is also responsible for its behavior on the road.
- 80See Noah J. Goodall, Virginia Center for Transportation Innovation and Re-search, Ethical Decision Making during Automated Vehicle Crashes, 2424 Transportation Research Record: Journal of the Transportation Research Board 58, 59 (2014) (noting that while “any engineering system can fail,” it is important to distinguish that, “for [ ] automated vehicle[s], even a perfectly-functioning system cannot avoid every collision”).
- 81Not in strictly monetary terms.
- 82See Judith Thomson, Killing, Letting Die, and the Trolley Problem, 59 Monist 204, 206 (1976). Although Thomson coined the term “trolley problem,” the first articulation of the thought experiment originated with the philosopher Philippa Foot. See Philippa Foot, The Problem of Abortion and the Doctrine of Double Effect, 5 Oxford Rev 5, 8–9 (1967).
- 83Thomson’s original experiment asked subjects to imagine themselves as the trolley driver rather than as an outside observer at a switch. Casey, 111 Nw U L Rev at 1353 (quotation marks and citations omitted) (cited in note 2), citing Thomson, 59 Monist at 206 (cited in note 82).
- 84See, for example, Wendell Wallach and Colin Allen, Moral Machines: Teaching Robots Right from Wrong 16 (Oxford 2009); Joel Achenbach, Driverless Cars Are Colliding with the Creepy Trolley Problem (Wash Post, Dec 29, 2015), online at http://www.washingtonpost.com/news/innovations/wp/2015/12/29/will-self-driving-cars-ever-solve-the-famous-and-creepy-trolley-problem (visited Apr 16, 2019) (Perma archive unavailable) (arguing that “we’re suddenly in a world in which autonomous machines, including self-driving cars, have to be programmed to deal with Trolley Problem-like emergencies in which lives hang in the balance”); John Markoff, Should Your Driverless Car Hit a Pedestrian to Save Your Life? (NY Times, June 23, 2016), archived at http://perma.cc/7VLC-5CNE (discussing the “dilemma of robotic morality” and its implications for engineers designing robotic decision-making systems); Matt Simon, To Make Us All Safer, Robocars Will Sometimes Have to Kill (Wired, Mar 13, 2017), archived at http://perma.cc/K2M3-GW6Y (“[T]he trolley problem . . . illustrates a strange truth: Not only will robocars fail to completely eliminate traffic deaths, but on very, very rare occasions, they’ll be choosing who to sacrifice—all to make the roads of tomorrow a far safer place.”).
- 85One curious approach is to ignore the problem altogether. German law simply forbids consideration of the trolley problem in programming autonomous vehicles, saying that an autonomous vehicle headed for an accident cannot alter its behavior to prefer one life over another. See Dave Gershgorn, Germany’s Self-Driving Car Ethicists: All Lives Matter (Quartz, Aug 24, 2017), archived at http://perma.cc/U7MX-2KNB; Federal Ministry of Transport and Digital Infrastructure, Report by the Ethics Commission on Automated and Connected Driving *10 (June 2017), archived at http://perma.cc/YYZ7-N54F. That does leave open the question of what the autonomous vehicle should do in an unavoidable accident situation, though. “Nothing” may often be the worst response.
- 86See Casey, 111 Nw U L Rev at 1363 (cited in note 2) (discussing how minute differences in how individual vehicles operate could have profoundly consequential macroscopic effects).
- 87This Article does not discuss substantive tort law distinctions found in modern tort doctrine.
- 88Steinberg, “Killer Robot” (cited in note 73).
- 89See Lorrie Cranor, A Framework for Reasoning about the Human in the Loop *2 (UPSEC 2008), archived at http://perma.cc/JA53-8AL8.
- 90See Michael Laris, Tempe Police Release Video of Moments before Autonomous Uber Hit Pedestrian (Wash Post, Mar 21, 2018), online at http://www.washingtonpost.com/news/dr-gridlock/wp/2018/03/21/tempe-police-release-video-of-moments-before-autonomous-uber-crash (visited Apr 17, 2019) (Perma archive unavailable).
- 91See id.
- 92Daisuke Wakabayashi, Emergency Braking Was Disabled When Self-Driving Uber Killed Woman, Report Says (NY Times, May 24, 2018), archived at http://perma.cc/S6PW-KMBD.
- 93Id.
- 94M.C. Elish, Moral Crumple Zones: Cautionary Tales in Human-Robot Interaction *23–25 (We Robot Conference Working Paper, Mar 2016), archived at http://perma.cc/LYB8-9PMN.
- 95Whether these types of questions will ultimately be resolved under the umbrella of negligence, breach of warranty, enterprise liability, or traditional product “defect” remains unclear.
- 96See, for example, Ryan Calo, et al, Is Tricking a Robot Hacking? *6–9 (We Robot Conference Working Paper, 2018), archived at http://perma.cc/4MW7-DR2M; Tom Simonite, Microsoft Chatbot Trolls Shoppers for Online Sex (Wired, Aug 7, 2017), archived at http://perma.cc/66VS-HYRR; Kevin Roose, Here Come the Fake Videos, Too (NY Times, Mar 4, 2018), archived at http://perma.cc/3336-E8SK.
- 97See Vincent, Twitter Taught Microsoft’s AI Chatbot to be a Racist Asshole (cited in note 23); Rachel Metz, Microsoft’s Neo-Nazi Sexbot Was a Great Lesson for Makers of AI Assistants (MIT Technology Review, Mar 27, 2018), archived at http://perma.cc/D3DH-CLEM.
- 98Vincent, Twitter Taught Microsoft’s AI Chatbot to be a Racist Asshole (cited in note 23).
- 99But we repeat ourselves.
- 100Metz, Microsoft’s Neo-Nazi Sexbot (cited in note 97).
- 101Sarah Perez, Microsoft Silences Its New A.I. Bot Tay, after Twitter Users Teach It Racism (TechCrunch, Mar 24, 2016), archived at http://perma.cc/6ZVQ-CP72. Other chatbots have also learned unexpected behavior. China shut down chatbots that began questioning the authority of the Communist Party. Neil Connor, Rogue Chatbots Deleted in China after Questioning Communist Party (The Telegraph, Aug 3, 2017), archived at http://perma.cc/Z4EG-2KWQ.
- 102One might argue that failing to plan for hijacking by Nazis was a defective design, just as a cybersecurity vulnerability might be. See Jonathan Zittrain, The Future of the Internet and How to Stop It 149–52 (Yale 2008) (arguing that “strengthen[ing] the Net’s experimentalist architecture . . . [and] creat[ing] . . . practices by which relevant people and institutions can help secure the Net themselves” will help “blunt[ ] the worst aspects of today’s popular generative Internet” without hurting innovation). Courts have been reluctant to declare insecure software to be defective, however. For discussion, see Bryan H. Choi, Crashworthy Code, 94 Wash L Rev 39, 62–65 (2019).
- 103For discussion of the various privacy issues that could arise from home robots, see generally Margot E. Kaminski, et al, Averting Robot Eyes, 76 Md L Rev 983 (2017).
- 104We discuss this problem in more detail in notes 116–25 and accompanying text.
- 105This could be either because of resource constraints involving safety testing or because they were genuinely unforeseeable.
- 106See Clay Shields, Why Do Computers Crash? (Scientific American, Jan 6, 2003), archived at http://perma.cc/4WY8-NX2S.
- 107Okay, maybe not that last one.
- 108See Balkin, 6 Cal L Rev Cir at 50 (cited in note 2).
- 109See Domingos, The Master Algorithm at 10 (cited in note 46) (“[M]achine learning is the ideal occupation, because learning algorithms do all the work but let you take all the credit.”).
- 110See Balkin, 6 Cal L Rev Cir at 51 (cited in note 2) (“Algorithms can . . . threaten, entertain, copy, defame, defraud, warn, console, or seduce. These various effects straddle the lines between the physical, the economic, the social, and the emotional.”).
- 111See, for example, David Silver, et al, Mastering the Game of Go without Human Knowledge, 550 Nature 354, 357 (Oct 19, 2017) (noting that the computer program that defeated a Go world champion discovered “non-standard strategies beyond the scope of traditional Go knowledge”); Nicola Twilley, Artificial Intelligence Goes to the Arcade (New Yorker, Feb 25, 2015), archived at http://perma.cc/NR4Z-HG4J (writing that “without any human coaching,” an AI system designed to play arcade games “not only bec[a]me better than any human player but [ ] also discovered a way to win that its creator never imagined”).
- 112See, for example, Taha Yasseri, Never Mind Killer Robots—Even the Good Ones Are Scarily Unpredictable (The Conversation, Aug 25, 2017), archived at http://perma.cc/A3KW-2SA7 (documenting an inadvertent war of proliferation between Wikipedia editing bots); Jessica Leber, Algorithmic Pricing Is Creating an Arms Race on Amazon’s Marketplace (Fast Company, June 14, 2016), archived at http://perma.cc/N52B-H7XT; Michael Eisen, Amazon’s $23,698,655.93 Book about Flies (It Is NOT Junk Blog, Apr 22, 2011), archived at http://perma.cc/8ZNV-4JY5; Samantha Raphelson, “Grinch Bots” Attempt to Steal Christmas by Driving Up Toy Prices (NPR, Dec 5, 2017), archived at http://perma.cc/5JVD-WKGA.
- 113See, for example, Tom McKay, No, Facebook Did Not Panic and Shut Down an AI Program That Was Getting Dangerously Smart (Gizmodo, July 31, 2017), archived at http://perma.cc/44KC-MCE4.
- 114See, for example, Jeanne Fromer and Mark A. Lemley, Playful Innovation (working paper 2019) (on file with authors); Mark A. Lemley, IP in a World without Scarcity, 90 NYU L Rev 460, 485 (2015) (“[T]he Internet carries a surprising lesson for IP theory: Despite the prevalence of infringement and the teachings of IP theory, people are creating and distributing more content now than ever before, by at least an order of magnitude.”).
- 115See, for example, Rob Ludacer, Watch a Tesla Predict an Accident and React Before It Even Happens (Business Insider, Dec 29, 2016), archived at http://perma.cc/5N5D-LM7K (showing a video of Tesla’s Autopilot doing just that).
- 116See Gregory F. Cooper, et al, An Evaluation of Machine-Learning Methods for Predicting Pneumonia Mortality, 9 Artificial Intelligence in Medicine 107, 109–11 (1997) (using data collected from seventy-eight hospitals to predict pneumonia mortality rates using a variety of machine learning methods); Richard Ambrosino, et al, The Use of Misclassification Costs to Learn Rule-Based Decision Support Models for Cost-Effective Hospital Admission Strategies in Reed M. Gardner, ed, Proceedings of the Annual Symposium on Computer Applications in Medical Care 304, 305–07 (1995) (using the same data to create an algorithm that accounts for asymmetric error costs when determining whether to prescribe inpatient or outpatient therapy for pneumonia patients).
- 117Rich Caruana, et al, Intelligible Models for HealthCare: Predicting Pneumonia Risk and Hospital 30-Day Readmission in Longbing Cao, et al, eds, Proceedings of the 21st ACM SIGKDD International Conference on Knowledge Discovery and Data Mining 1721, 1721 (2015).
- 118Id.
- 119Id.
- 120Id.
- 121Caruana et al, Intelligible Models for HealthCare at 1722 (cited in note 117).
- 122For example, IBM’s Watson, a supercomputer system trained to answer questions posed in natural language, was recently reported as displaying “multiple examples of unsafe and incorrect treatment recommendations.” Jennings Brown, IBM Watson Reportedly Recommended Cancer Treatments That Were “Unsafe and Incorrect” (Gizmodo, July 25, 2018), archived at http://perma.cc/Z544-TH4K.
- 123Marc C. Canellas, et al, Framing Human-Automation Regulation: A New Modus Operandi from Cognitive Engineering *41 (We Robot Conference Working Paper, Mar 2017), archived at http://perma.cc/ZVM5-T54N.
- 124Leptokurtic distributions show higher peaks around mean values and higher densities of values at the tail ends of the probability curve.
- 125Canellas, et al, Framing Human-Automation Regulation at *41 (cited in note 123).
- 126See Lori G. Kletzer, The Question with AI Isn’t Whether We’ll Lose Our Jobs—It’s How Much We’ll Get Paid (Harvard Business Review, Jan 31, 2018), archived at http://perma.cc/L5KY-XE4X (“Currently, most automation involves routine, structured, predictable physical activities and the collection and processing of data.”).
- 127See generally Cathy O’Neil, Weapons of Math Destruction: How Big Data Increases Inequality and Threatens Democracy (Penguin 2016).
- 128See Selbst, 52 Ga L Rev at 133–35 (cited in note 59).
- 129See O’Neil, Weapons of Math Destruction at 16 (cited in note 127).
- 130Illinois Radio Network, How Are Car Insurance Rates Set? (WSIU, July 31, 2015), archived at http://perma.cc/X74X-J74D. A credit score “summarizes an individual’s credit history and financial activities in a way that informs the bank about their creditworthiness.” Lydia T. Liu, et al, Delayed Impact of Fair Machine Learning (Berkeley Artificial Intelligence Research, May 17, 2018), archived at http://perma.cc/GGM6-KPPM.
- 131Christina Couch, Ghosts in the Machine (NOVA, Oct 25, 2017), archived at http://perma.cc/98JM-4PLW.
- 132See id.
- 133Id.
- 134See, for example, Jonas Lerman, Big Data and Its Exclusions, 66 Stan L Rev Online 55, 57 (2013) (warning of the capacity for big data to systematically marginalize people who, “whether due to poverty, geography, or lifestyle . . . are less ‘datafied’ than the general population[ ]”); Brent Daniel Mittelstadt, et al, The Ethics of Algorithms: Mapping the Debate, 3 Big Data & Society 1, 8 (2016) (discussing how profiling by algorithms, which “identify correlations and make predictions about behavior at a group-level, . . . can inadvertently create an evidence-base that leads to discrimination”); Danielle Keats Citron and Frank Pasquale, The Scored Society: Due Process for Automated Predictions, 89 Wash L Rev 1, 10–18 (2014) (describing the potential for “black box” credit scoring to produce arbitrary results that reinforce inequality); Solon Barocas, Data Mining and the Discourse on Discrimination (Proceedings of the Data Ethics Workshop, 2014), archived at http://perma.cc/G9NA-6B7V (explaining the potential harms created by data mining with an example of how predictive policing can produce feedback loops that distort policing priorities); Bart Custers, Data Dilemmas in the Information Society: Introduction and Overview, in Bart Custers, et al, eds, Discrimination and Privacy in the Information Society 3, 22 (Springer-Verlag 2013) (describing how algorithmic “affirmative action” could be used to address gender discrimination in salary predictions); Latanya Sweeney, Discrimination in Online Ad Delivery, in 56 Communications of the ACM 44, 50–51 (2013) (documenting a statistically significant difference in the number of Google AdSense advertisements that included words such as “arrest” and “criminal” when searching black-associated versus white-associated names); Shoshana Zuboff, Big Other: Surveillance Capitalism and the Prospects of an Information Civilization, 30 J Information Tech 75, 81–82 (2015) (warning of the “chilling effects of anticipatory conformity” that emerge from “a ubiquitous networked institutional regime that records, modifies, and commodifies everyday experience . . . all with a view to establishing new pathways to monetization and profit”); Crawford and Schultz, 55 BC L Rev at 101 (cited in note 2) (explaining how big data can provide sophisticated tools to housing suppliers that allow subtler versions of housing discrimination to persist). The problem is not just explicit bias, but the consideration of variables with a disparate impact on particular groups. See Selbst, 52 Ga L Rev at 133–35 (cited in note 59).
- 135See generally, for example, Jason Kreag, Prosecutorial Analytics, 94 Wash U L Rev 771 (2017) (explaining how statistical analysis of prosecutorial discretion can provide better information necessary to reduce bias and misconduct).
- 136See generally Cass R. Sunstein, Algorithms, Correcting Biases (working paper, 2018), archived at http://perma.cc/5D72-XL6L (arguing that algorithms can be better than humans at avoiding consideration of race or other factors).
- 137This phrase comes from Professor Calo and refers to the fact that digital information “faces few natural barriers to dissemination.” See Calo, 103 Cal L Rev at 532–34 (cited in note 2).
- 138The “Internet of Things” refers to the embedding of networked devices in everyday objects, thereby allowing them to gather, send, and receive data.
- 139Balkin, 6 Cal L Rev Cir at 53 (cited in note 2). See also James Grimmelmann, Regulation by Software, 114 Yale L J 1719, 1742–43 (2005).
- 140See Ryan Calo, People Can Be So Fake: A New Dimension to Privacy and Technology Scholarship, 114 Penn St L Rev 809, 838–42 (2010); Kaminski, et al, 76 Md L Rev at 997, 1001–24 (cited in note 103) (noting this problem and offering design principles to minimize it).
- 141For a discussion of the implications of household robots for American privacy law, see generally Margot E. Kaminski, Robots in the Home: What Will We Have Agreed To?, 51 Idaho L Rev 661 (2015).
- 142See, for example, Complaint for Damages, Nilsson v General Motors LLC, No 3:18-cv-00471, *3–4 (ND Cal filed Jan 22, 2018) (litigation alleging that an autonomous vehicle operated by General Motors operated itself negligently); O’Brien v Intuitive Surgical, Inc, 2011 WL 3040479, *1 (ND Ill) (litigation involving injuries allegedly caused by the “da Vinci surgical robot”); Hills v Fanuc Robotics America, Inc, 2010 WL 890223, *1, 4 (ED La) (litigation by employee injured by a robot used to stack crates on wooden pallets); Kharpal, Robot with $100 Bitcoin Buys Drugs (cited in note 73) (describing the “curious story of how a robot armed with a weekly budget of $100 in bitcoin managed to buy Ecstasy, a Hungarian passport and a baseball cap with a built-in camera—before getting arrested”).
- 143See generally, for example, Mark A. Geistfeld, A Roadmap for Autonomous Vehicles: State Tort Liability, Automobile Insurance, and Federal Safety Regulation, 105 Cal L Rev 1611 (2017); Kenneth S. Abraham and Robert L. Rabin, Automated Vehicles and Manufacturer Responsibility for Accidents: A New Legal Regime for a New Era, 105 Va L Rev 127 (2019); Bryant Walker Smith, Automated Driving and Product Liability, 2017 Mich St L Rev 1; A. Michael Froomkin and P. Zak Colangelo, Self-Defense against Robots and Drones, 48 Conn L Rev 1 (2015).
- 144Frederick Pollock, The Continuity of the Common Law, 11 Harv L Rev 423, 424 (1898) (noting the phrase already functioned as a “maxim” in the 19th century).
- 145Douglas Laycock, Modern American Remedies 3 (Aspen 4th ed 2010).
- 146Id.
- 147Id at 14–15.
- 148It is possible to imagine robot plaintiffs. Robots can certainly be injured by humans. You might run a stop light and hit my self-driving car, for example. Or people might attack a robot. See, for example, Isobel Asher Hamilton, People Kicking These Food Delivery Robots Is an Early Insight into How Cruel Humans Could Be to Robots (Business Insider, June 9, 2018), archived at http://perma.cc/LRL3-PMF2 (the headline says it all); Russ Mitchell, Humans Slapped and Shouted at Robot Cars in Two of Six DMV Crash Reports This Year (LA Times, Mar 5, 2018), archived at http://perma.cc/4XT2-WLJW; Silicon Valley Security Robot Attacked by Drunk Man—Police (BBC News, Apr 26, 2017), archived at http://perma.cc/9MT8-3FU2. The robot itself presumably won’t have a right to sue, at least for the foreseeable future. But the owner of the robot might sue for damages. That doesn’t seem to present significant remedies issues different from ordinary property damages cases, though. Valuing the loss of an individual robot or AI that has learned in ways that differ from factory settings may present difficulties akin to the valuation of any unique asset. But that’s likely to be rare, since people will presumably back up their unique AIs periodically.
- 149Laycock, Modern American Remedies at 11–15 (cited in note 145).
- 150Courts do this when they order structural reforms to prisons, hospitals, or schools, for instance. See, for example, Hutto v Finney, 437 US 678, 685–88 (1978) (upholding a district court order placing a maximum limit of thirty days on punitive solitary confinement). But see, for example, Missouri v Jenkins, 515 US 70, 86–103 (1995) (rejecting the district court’s desegregation plan, which required the State of Missouri to increase funding for staff and remedial programs, because it was beyond the court’s remedial authority).
- 151Laycock, Modern American Remedies at 4, 7–8 (cited in note 145).
- 152See generally Guido Calabresi and A. Douglas Melamed, Property Rules, Liability Rules, and Inalienability: One View of the Cathedral, 85 Harv L Rev 1089 (1972).
- 153See Ian Ayres and Eric Talley, Solomonic Bargaining: Dividing a Legal Entitlement to Facilitate Coasean Trade, 104 Yale L J 1027, 1032–33 (1995). But see Louis Kaplow and Steven Shavell, Do Liability Rules Facilitate Bargaining? A Reply to Ayres and Talley, 105 Yale L J 221, 225–30 (1995) (critiquing Ayres and Talley’s argument “that, when bargaining is imperfect, ‘liability rules possess an “information-forcing” quality’ that ‘may induce both more contracting and more efficient contracting than property rules’”).
- 154See Calabresi and Melamed, 85 Harv L Rev at 1092 (cited in note 152).
- 155Oliver Wendell Holmes Jr, The Path of the Law, 10 Harv L Rev 457, 459 (1897).
- 156Laycock, Modern American Remedies at 7 (cited in note 145). See also generally Yuval Feldman, The Law of Good People: Challenging States’ Ability to Regulate Human Behavior (Cambridge 2018) (arguing that we should focus legal rules on the signals they send to good people rather than just constraining the behavior of bad people).
- 157458 NE2d 827 (Ohio 1984).
- 158Id at 827.
- 159Id at 831.
- 160See Marco Jimenez, Finding the Good in Holmes’s Bad Man, 79 Fordham L Rev 2069, 2069 (2011):
[A] careful reading of Holmes suggests that he was himself well aware of the intimate relationship between law and morality, and seems to have recognized, somewhat surprisingly, that only by engaging in an analytical separation of these two concepts can they then be normatively reunited in an intellectually consistent and satisfying manner.
- 161Id at 2103–06.
- 162Corporations are more likely to do so. Because we can’t put a corporation itself in jail, corporate compliance—even with penalties designed to stop conduct rather than just internalize costs—might nonetheless be viewed as a cost of doing business for the corporation.
- 163An IF, THEN statement—or “if-then-else statement”—refers to an expression that conditionally executes a statement or group of statements.
- 164And probably not even then, unless the robot’s algorithm preferences its own survival over most other outcomes (which it probably won’t).
- 165Isaac Asimov, The Rest of the Robots 43 (Doubleday 1964) (remarking that “[t]here was just enough ambiguity in [Asimov’s] Three Laws [of robotics] to provide the conflicts and uncertainties required for new stories, and, to my great relief, it seemed always to be possible to think up a new angle out of the sixty-one words of the Three Laws”).
- 166“Don’t become Skynet” does seem like a good one to include, though. See Randall Munroe, Genetic Algorithms (XKCD), archived at http://perma.cc/W6KZ-65SH.
- 167By “internalization,” we do not necessarily mean that the law should attempt to put an explicit monetary value on every conceivable form of harmful conduct. Rather, internalities and externalities can be addressed by a multitude of direct and indirect means, just as the law does today.
- 168See Casey, 111 Nw U L Rev at 1357–59 (cited in note 2).
- 169See generally Robert Cooter, Prices and Sanctions, 84 Colum L Rev 1523 (1984) (discussing this distinction and how to choose between them).
- 170Laycock, Modern American Remedies at 7 (cited in note 145).
- 171Consider Christoph Bezemek, Bad for Good: Perspectives on Law and Force, in Christoph Bezemek and Nicoletta Ladavac, eds, The Force of Law Reaffirmed: Frederick Schauer Meets the Critics 15 (Springer 2016) (arguing that the perspective of the Holmesian “bad man” is useful for understanding the “general relationship between law and force”).
- 172See Kharpal, Robot with $100 Bitcoin Buys Drugs (cited in note 73). See also Shawn Bayern, Of Bitcoins, Independently Wealthy Software, and the Zero-Member LLC, 108 Nw U L Rev 1485, 1495 (2014) (explaining how tort and contract law can regulate “autonomous systems”).
- 173See United States v Athlone Industries, Inc, 746 F2d 977, 979 (3d Cir 1984) (noting that “robots cannot be sued”). See also Roger Michalski, How to Sue a Robot, 2018 Utah L Rev 1021, 1063–64 (arguing that robots should be a new form of entity for litigation purposes).
- 174See European Parliament Committee on Legal Affairs, Report with Recommendations to the Commission on Civil Law Rules on Robots *16–17 (Jan 27, 2017), archived at http://perma.cc/JM8H-DYAV.
- 175See Wakabayashi, Self-Driving Uber Car Kills Pedestrian (cited in note 20).
- 176See Geistfeld, 105 Cal L Rev at 1634–35 (cited in note 143).
- 177See Daniel A. Crane, Kyle D. Logue, and Bryce C. Pilz, A Survey of Legal Issues Arising from the Deployment of Autonomous and Connected Vehicles, 23 Mich Telecomm & Tech L Rev 191, 215 (2017).
- 178See Lothar Determann and Bruce Perens, Open Cars, 32 Berkeley Tech L J 915, 984–86 (2017); Ryan Calo, Open Robotics, 70 Md L Rev 571, 601–11 (2011) (proposing a liability regime for open-source robots that would balance the goals of fostering innovation and incentivizing safety).
- 179See Vincent, Twitter Taught Microsoft’s AI Chatbot to Be a Racist Asshole (cited in note 23). See also text accompanying notes 97–102. In retrospect, this event probably should have been a wake-up call for 2016.
- 180See Yavar Bathaee, The Artificial Intelligence Black Box and the Failure of Intent and Causation, 31 Harv J L & Tech 889, 931–32 (2018) (opposing strict liability for this reason).
- 181See Science Diction: The Origin of the Word ‘‘Robot’’ (NPR, Apr 22, 2011), archived at http://perma.cc/GCT6-3VLU (noting that “[the word ‘robot’] comes from an Old Church Slavonic word, rabota, which means servitude of forced labor”).
- 182See United States v Carroll Towing Co, 159 F2d 169, 171–73 (2d Cir 1947) (the case in which Judge Learned Hand first expressed his canonical negligence formula).
- 183See, for example, H. Laurence Ross, Settled out of Court: The Social Process of Insurance Claims Adjustment 133–35 (Aldine 2d ed 1980) (discussing the routinization of negligence and insurance compensation formulas for auto accidents).
- 184See Laycock, Modern American Remedies at 165–66 (cited in note 145) (discussing multiple studies showing disagreement among juries “over how to convert severity of injury into dollars”).
- 185See notes 231–32 and accompanying text.
- 186See notes 288–89 and accompanying text.
- 187See, for example, Malcolm Gladwell, The Engineer’s Lament: Two Ways of Thinking about Automotive Safety (The New Yorker, May 4, 2015), archived at http://perma.cc/6QSS-D3H3 (describing jurors’ horror at internal memos that seemed to callously weigh the value of human lives against business considerations).
- 188See Casey, 111 Nw U L Rev at 1359 n 70 (cited in note 2) (discussing “the warped incentive signals conceivably sent [to robots] by transaction costs, first- and third-party insurance intermediaries, administrative costs, technical limitations, agency costs, information costs, human error and incompetence, consumer psychology, potential media backlash, and judicial and regulatory uncertainty”).
- 189See generally Jonathan Taplin, Move Fast and Break Things: How Facebook, Google, and Amazon Cornered Culture and Undermined Democracy (Little, Brown 2017).
- 190At least not most of them.
- 191For a discussion of other agency costs that can arise in modern corporate structures, see Ronald J. Gilson and Jeffrey N. Gordon, The Agency Costs of Agency Capitalism: Activist Investors and the Revaluation of Governance Rights, 113 Colum L Rev 863, 889–95 (2013).
- 192Or face time behind bars.
- 193As it gets easier to design AIs, these entities will be increasingly judgment-proof. That will make us want to look upstream past the owner/user to the manufacturer. A second and more significant category of circumstances where a robot might depart from purely profit-maximizing behavior involves instances where the chain of legal responsibility running from the robot to the manufacturer is intermediated by a downstream user.
- 194See Laycock, Modern American Remedies at 698–99 (cited in note 145).
- 195Id at 655–63.
- 196Id.
- 197Id.
- 198See Robert D. Cooter, Punitive Damages, Social Norms, and Economic Analysis, 60 L & Contemp Probs 73, 76–77 (1997) (“[Because] an injurer is indifferent between no injury and an injury with liability for perfectly disgorging damages . . . an injurer who faces certain liability for extra-disgorging damages prefers not to cause the injury.”); Bert I. Huang, Essay, The Equipoise Effect, 116 Colum L Rev 1595, 1598 (2016).
- 199See notes 183–87 and accompanying text.
- 200See Mark Lemley, The Fruit of the Poisonous Tree in IP Law, 103 Iowa L Rev 245, 266–69 (2017) (explaining when and how IP regimes should limit the “fruit of the poisonous tree doctrine” to trade secret infringement). For examples, see K-2 Ski Co v Head Ski Co, 506 F2d 471, 474 (9th Cir 1974) (“We are satisfied that the appropriate duration for the injunction should be the period of time it would have taken Head, either by reverse engineering or by independent development, to develop its ski legitimately without use of the K-2 trade secrets.”); Winston Research Co v Minnesota Mining and Manufacturing Co, 350 F2d 134, 145–46 (9th Cir 1965) (discussing injunction protection for a machine company); Verigy US, Inc v Mayder, 2008 WL 564634, *9, 11 (ND Cal) (granting a five-month injunction to account for the lag time defendant would have faced in getting to market absent misappropriation).
- 201Theoretically this is true of people too if they are rational profit maximizers. But many won’t be. See, for example, Daniel L. McFadden, The New Science of Pleasure *23–24, 37 (NBER Working Paper No 18687, Jan 2013), archived at http://perma.cc/Y5B3-MR93 (finding that people often don’t maximize profit); Christine Jolls, Cass R. Sunstein, and Richard Thaler, A Behavioral Approach to Law and Economics, 50 Stan L Rev 1471, 1476–81 (1998) (discussing the ways people make systematically “irrational” decisions).
- 202This term refers to algorithms that are inscrutable to outsiders, either by virtue of complexity, lack of technical fluency, or trade secrets protection.
- 203See, for example, James Grimmelmann and Daniel Westreich, Incomprehensible Discrimination, 7 Cal L Rev Online 164, 173 (2017); Frank Pasquale, The Black Box Society: The Secret Algorithms That Control Money and Information 34–35 (Harvard 2015) (discussing the “black box” harms associated from “hyperefficient,” “data-driven” management policies at the workplace).
- 204See, for example, Roger Parloff, How VW Paid $25 Billion for ‘‘Dieselgate’’—and Got Off Easy (Fortune, Feb 6, 2018), archived at http://perma.cc/H4HJ-GDGD (detailing Volkswagen’s decade-long effort to cheat diesel emissions tests in order to falsely market its vehicles as “Clean Diesel”); Margaret Levenstein, Valerie Suslow, and Lynda Oswald, International Price-Fixing Cartels and Developing Countries: A Discussion of Effects and Policy Remedies *10–29 (NBER Working Paper No 9511, Feb 2003), archived at http://perma.cc/S9SL-FYDR (describing prosecutions against three international corporations accused of colluding to fix prices and evade legal detection).
- 205Professor Deven Desai and Joshua Kroll argue for protections for whistleblowers who identify flaws in robotic design in an effort to reduce the risk of such cover-ups. See Deven R. Desai and Joshua A. Kroll, Trust but Verify: A Guide to Algorithms and the Law, 31 Harv J L & Tech 1, 56–60 (2017).
- 206Pricing algorithms may effectively replicate the anticompetitive effects of a cartel by predicting the behavior of their rivals, for instance. See Michal S. Gal, Algorithms as Illegal Agreements, 34 Berkeley Tech L J 67, *97–115 (2019); Kellie Lerner and David Rochelson, How Do You Solve a Problem Like Algorithmic Price Fixing?, 111 Antitrust & Trade Reg Daily 157, 158–59 (2018). But see generally Ulrich Schwalbe, Algorithms, Machine Learning, and Collusion (working paper, June 2018), archived at http://perma.cc/RNR5-WL4B (arguing that algorithmic collusion is harder than assumed, but basing that conclusion on the dubious assumption that it will require direct communication between the algorithms).
- 207See, for example, Gary S. Becker, Crime and Punishment: An Economic Approach, 76 J Polit Econ, 169, 190–93 (1968); Gary S. Becker and George J. Stigler, Law Enforcement, Malfeasance, and Compensation of Enforcers, 3 J Legal Stud 1, 6–13 (1974); Richard A. Posner, Optimal Sentences for White-Collar Criminals, 17 Am Crim L Rev 409, 410 (1980); A. Mitchell Polinsky and Steven Shavell, The Economic Theory of Public Enforcement of Law, 38 J Econ Lit 45, 53–56 (2000).
- 208High sanctions, for example, “may lead juries to be less likely to convict defendants, or may induce injurers to engage in greater efforts to avoid detection.” Polinsky and Shavell, 38 J Econ Lit at 49 n 15 (cited in note 207).
- 209Or perhaps we will. We tend to anthropomorphize at least human-seeming robots. See generally Kate Darling, Palash Nandy, and Cynthia Breazeal, Empathic Concern and the Effect of Stories in Human-Robot Interaction (24th IEEE International Symposium on Robot and Human Interactive Communication, 2015), archived at http://perma.cc/7A73-WQCG.
- 210See Part II.C.2.
- 211See David S. Levine, Secrecy and Unaccountability: Trade Secrets in Our Public Infrastructure, 59 Fla L Rev 135, 177–87 (2007) (providing examples of how information protected by the trade secrecy doctrine has created significant problems for public infrastructure and accountability); Rebecca Wexler, Life, Liberty, and Trade Secrets: Intellectual Property in the Criminal Justice System, 70 Stan L Rev 1343, 1365 (2018) (describing trend toward increasing use of trade secrets claims to prevent outside scrutiny of algorithmic systems); Sonia K. Katyal, Private Accountability in the Age of Artificial Intelligence, 66 UCLA L Rev 54, 121–25 (2009) (discussing “the variety of ways in which companies routinely utilize their intellectual property protections to obfuscate inquiry”).
- 212Alexander Campolo, et al, AI Now 2017 Report *16 (AI Now Institute, Nov 2017), archived at http://perma.cc/VH2C-9BVQ (citations omitted).
- 213Bryce Goodman and Seth Flaxman, European Union Regulations on Algorithmic Decision-Making and a “Right to Explanation” *6 (ICML Workshop on Human Interpretability in Machine Learning, 2016), archived at http://perma.cc/D77Z-J5F6.
- 214Id. For an argument that we can nonetheless improve interpretability, see Andrew D. Selbst and Solon Barocas, The Intuitive Appeal of Explainable Machines, 87 Fordham L Rev 1085, 1129–38 (2018).
- 215Id at 1094–96.
- 216See Campolo, et al, AI Now 2017 Report at *36 (cited in note 212).
- 217See Oscar H. Gandy Jr, Engaging Rational Discrimination: Exploring Reasons for Placing Regulatory Constraints on Decision Support Systems, 12 Ethics & Info Tech 29, 30 (2009) (arguing that automated systems reproduce biases in human data).
- 218Among the many calls for transparency, see generally Pasquale, The Black Box Society (cited in note 203); Katyal, 66 UCLA L Rev 54 (cited in note 211); Citron and Pasquale, 89 Wash L Rev 1 (cited in note 134); Frank Pasquale, Toward a Fourth Law of Robotics: Preserving Attribution, Responsibility, and Explainability in an Algorithmic Society, 78 Ohio St L J 1243 (2017).
- 219See Desai and Kroll, 31 Harv J L & Tech at 29–35 (cited in note 205) (arguing that the push for transparency is misguided because it misunderstands the nature of the algorithms at stake); Selbst and Barocas, 87 Fordham L Rev at 1126–29 (cited in note 214) (arguing that we should rely on other means than intuition in assessing the judgments of AIs that use statistical results rather than explainable rules).
A different form of transparency may be easier with robots. Robots may be able to signal their intentions. Just as drivers use turn indicators and brake lights to telegraph their plans, robots might devise ways to communicate what they will do to those around them. See, for example, Christopher Paul Urmson, et al, Pedestrian Notifications, US Patent No 9,196,164 B1 (filed Nov 24, 2015) (describing a self-driving car that carries a pedestrian notification sign indicating whether the car has seen the pedestrian and it is safe to cross). And they may be required to identify themselves; it is not clear that the constitutional right to anonymity extends to robots. See Pasquale, 78 Ohio St L J at 1253 (cited in note 218). See also 14 CFR § 48.15 (requiring registration of all “unmanned aircrafts”).
- 220Professor Frank Pasquale calls this “explainability.” Pasquale, 78 Ohio St L J at 1252 (cited in note 218). This is what the General Data Protection Regulation (GDPR) requires, for instance. For a discussion, see generally Margot E. Kaminski, Binary Governance: A Two-Part Approach to Accountable Algorithms, 92 S Cal L Rev (forthcoming 2019) (on file with author).
- 221See Ian Goodfellow, Yoshua Bengio, and Aaron Courville, Deep Learning 1–2 (MIT 2016); Bathaee, 31 Harv J L & Tech at 929–30 (cited in note 180) (“[I]t may be that as these networks become more complex, they become correspondingly less transparent and difficult to audit and analyze.”).
- 222We made these examples up. The real ones are likely to be even weirder. The whole point is that they are inexplicable to humans. Even today, AI is making decisions humans struggle to understand. Dave Gershgorn, AI Is Now So Complex Its Creators Can’t Trust Why It Makes Decisions (Quartz, Dec 7, 2017), archived at http://perma.cc/HJH3-9GX5. Some companies are studying the decisions of their own AIs to try to unpack how they are made. See Cade Metz, Google Researchers Are Learning How Machines Learn (NY Times, Mar 6, 2018), archived at http://perma.cc/HDL4-2VH4.
- 223See Selbst and Barocas, 87 Fordham L Rev at 1126–29 (cited in note 214) (describing the demand for “intelligible” models and the limitations such a requirement would impose on innovation).
- 224See Lilian Edwards and Michael Veale, Slave to the Algorithm: Why a “Right to an Explanation” Is Probably Not the Remedy You’re Looking For, 16 Duke L & Tech Rev 18, 65–67 (2017); Mike Ananny and Kate Crawford, Seeing without Knowing: Limitations of the Transparency Ideal and Its Applications to Algorithmic Accountability, 20 New Media & Society 973, 979–82 (2018).
- 225See Parts III.A–B.
- 226See Peter M. Asaro, Punishment, Reinforcement Learning, and Machine Agency (Feb 2013), archived at http://perma.cc/C5ET-448U:
[A] key intuitive difference between humans . . . and machines is that when a human misbehaves, you punish it, whereas when a machine does, you fix it. On our present theory, however, it becomes clear that punishing and fixing are essentially the same: punishing is a clumsy, external way of modifying the utility function.
- 227See, for example, Kate Darling, Children Beating Up Robot Inspires New Escape Maneuver System (IEEE Spectrum, Aug 6, 2015), archived at http://perma.cc/HM62-WWD8; Evan Ackerman, Robotic Tortoise Helps Kids to Learn That Robot Abuse Is a Bad Thing (IEEE Spectrum, Mar 14, 2018), archived at http://perma.cc/WB4L-TFJP. See also Mulligan, 69 SC L Rev at 585–89 (cited in note 6).
- 228Mark Geistfeld, Reconciling Cost-Benefit Analysis with the Principle That Safety Matters More Than Money, 76 NYU L Rev 114, 125–26 (2001).
- 229“Global variation in estimates of the value of life range from $70,000 to $16.3 million.” Deborah L. Rhode, et al, Legal Ethics 645 (Foundation 7th ed 2016). “In the United States, federal agencies operate with figures generally ranging from roughly $6 to $9 million—but tort awards for wrongful death are typically a fraction of that, and even agency estimates tend to shift with the political winds.” Id, citing Eric A. Posner and Cass R. Sunstein, Dollars and Death, 72 U Chi L Rev 537 (2005); Binyamin Appelbaum, As U.S. Agencies Put More Value on a Life, Businesses Fret (NY Times, Feb 16, 2011), archived at http://perma.cc/L4BX-RD6E.
- 230See generally Cass R. Sunstein, Lives, Life-Years, and Willingness to Pay, 104 Colum L Rev 205 (2004).
- 231See, for example, Gladwell, The Engineer’s Lament (cited in note 187) (describing this phenomenon unfolding in the infamous Ford Pinto controversy).
- 232See, for example, id.
- 233See Mulligan, 69 SC L Rev at 585–89 (cited in note 6). Or even if it hasn’t done us wrong, some people may want to punch a robot just because they are jerks. See Katherine Hignett, Locals Attacking Waymo Self-Driving Cars Being Tested in Arizona (Newsweek, Dec 15, 2018), archived http://perma.cc/M4R4-9P5W.
- 234See note 148 and accompanying text.
- 235See Ryan Abbott and Alex Sarch, Punishing Artificial Intelligence: Legal Fiction or Science Fiction, 53 UC Davis L Rev *17–19 (forthcoming 2019), archived at http://perma.cc/K9FN-94T2.
- 236See, for example, Robbie Gonzalez, Hey Alexa, What Are You Doing to My Kid’s Brain? (Wired, May 11, 2018), archived at http://perma.cc/N4FV-7AWN (describing the tendency for children to anthropomorphize chat bots like Amazon’s Alexa).
- 237See Calo, 103 Cal L Rev at 545–49 (cited in note 2) (terming this phenomenon “social valence”).
- 238Indeed, experimental evidence suggests that people are less likely to turn off a robot if it asks them not to. Aike C. Horstmann, et al, Do a Robot’s Social Skills and Its Objection Discourage Interactants from Switching the Robot Off? *1, 16–20 (Public Library of Science, July 31, 2018), archived at http://perma.cc/Q2ER-HRMX. Like Asimov’s fiction, Westworld’s days as pure fantasy may be numbered.
- 239It’s an open question whether we will react differently to a self-learning AI that isn’t in corporeal form and doesn’t act in humanlike ways.
- 240See notes 181–84 and accompanying text.
- 241Or, more precisely, no cop should ticket you.
- 242Former Secretary of Defense Donald Rumsfeld famously described these types of foreseeability concerns:
[T]here are known knowns; there are things that we know we know. We also know there are known unknowns; that is to say we know there are some things we do not know. But there are also unknown unknowns—the ones we don’t know we don’t know. And if one looks throughout the history of our country and other free countries, it is the latter category that tend to be the difficult ones.
Rumspeak (The Economist, Dec 4, 2003), archived at http://perma.cc/84X7-MJA7.
- 243Tim Urban, The AI Revolution: Our Immortality or Extinction (Wait But Why, Jan 27, 2015), online at http://waitbutwhy.com/2015/01/artificial-intelligence-revolution-2.html (visited Apr 18, 2019) (Perma archive unavailable).
- 244This is a variation on Eliezer Yudkowsky’s and Nick Bostrom’s famous “paper clip maximizer” thought experiment. See Nick Bostrom, Ethical Issues in Advanced Artificial Intelligence *2, 5 (Jan 2003), archived at http://perma.cc/WWR9-XPFR.
- 245See, for example, Search King, Inc v Google Technology, Inc, 2003 WL 21464568, *7–8 (WD Okla) (ruling that Google’s page rankings were “subjective result[s]” that constituted “constitutionally protected opinions” entitled to “full constitutional protection”); Langdon v Google, Inc, 474 F Supp 2d 622, 629–30 (D Del 2007) (refusing to affirmatively order Google and Microsoft to rank certain search results prominently on First Amendment grounds); United States v Microsoft Corp, 253 F3d 34, 59 (DC Cir 2001) (applying balancing test to judge whether new product is predatory); United States v Microsoft Corp, 147 F3d 935, 955 (DC Cir 1998) (deferring to a company’s claims of product improvement to avoid enmeshing the court in design decisions); Allied Orthopedic Appliances, Inc v Tyco Health Care Group, 592 F3d 991, 998–99 (9th Cir 2010) (holding that a firm’s improvement on its own product’s design cannot by itself be sufficient grounds for a finding of a Sherman Act violation).
- 246Dan L. Burk and Mark A. Lemley, Fence Posts or Sign Posts? Rethinking Patent Claim Construction, 157 U Pa L Rev 1743, 1744 (2009) (detailing the fraught history of “parties and courts [being] unable to agree on what particular patent claims mean” due to “plausible disagreements over the meanings of the words” in the claims). See, for example, Phillips v AWH Corp, 415 F3d 1303, 1309 (Fed Cir 2005) (en banc); North American Vaccine, Inc v American Cyanamid Co, 7 F3d 1571, 1581 (Fed Cir 1993) (resolving a patent dispute between parties over the meaning of the word “a”); Kustom Signals, Inc v Applied Concepts, Inc, 264 F3d 1326, 1331 (Fed Cir 2001); Chef America, Inc v Lamb-Weston, Inc, 358 F3d 1371, 1374 (Fed Cir 2004); Toro Co v White Consolidated Industries, Inc, 199 F3d 1295, 1300–02 (Fed Cir 1999); Cybor Corp v FAS Technologies, Inc, 138 F3d 1448, 1459 (Fed Cir 1998) (en banc); Sage Products, Inc v Devon Industries, Inc, 126 F3d 1420, 1430–31 (Fed Cir 1997).
- 247See Part I.C.5.
- 248See Danielle Keats Citron, Technological Due Process, 85 Wash U L Rev 1249, 1308–11 (2008).
- 249Well, some do. See Karen Turner, Meet “Ross,” the Newly Hired Legal Robot (Wash Post, May 16, 2016) online at http://www.washingtonpost.com/news/innovations/wp/2016/05/16/meet-ross-the-newly-hired-legal-robot (visited Apr 18, 2019) (Perma archive unavailable) (describing Lex Machina’s ability to “mine[ ] public court documents using natural language processing to help predict how a judge will rule in a certain type of case”). See also Cade Metz and Steve Lohr, IBM Unveils System That “Debates” with Humans (NY Times, June 18, 2018), archived at http://perma.cc/7VDC-HTQ8.
- 250More on this below when we consider the robot death penalty. See notes 312–14 and accompanying text.
- 251Far from hypothetical, courts have considered these types of arguments on multiple occasions in recent years. See, for example, State v Loomis, 881 NW2d 749, 767–73 (Wis 2016) (permitting the use of risk assessment algorithms in sentencing decisions on the condition that improper factors like race and gender are excluded from the risk assessment). See also Malenchik v State, 928 NE2d 564, 575 (Ind 2010) (“We hold that the results of . . . offender assessment [algorithms] are appropriate supplemental tools for judicial consideration at sentencing.”).
- 252See, for example, Bernard E. Harcourt, Risk as a Proxy for Race: The Dangers of Risk Assessment, 27 Fed Sent Reptr (Vera) 237, 238–40 (2015) (“Risk, today, is predominantly tied to prior criminal history, and prior criminality has become a proxy for race. The result is that decarcerating by means of risk instruments is likely to aggravate the racial disparities in our already overly racialized prisons.”).
- 253Whether we want to disproportionately punish poor people is another matter, of course, but doing so isn’t race discrimination.
- 254See for example, Kristen M. Altenburger and Daniel E. Ho, When Algorithms Import Private Bias into Public Enforcement: The Promise and Limitations of Statistical Debiasing Solutions, 175 J Institutional & Theoretical Econ 98, 98 (2019) (showing a solution purporting to “debias” predictive algorithms “may be limited when protected groups have distinct predictor distributions, due to model extrapolation”).
- 255It is possible a company will simply factor the cost of contempt into the algorithm, but that seems unlikely. And if they do, courts will probably not be happy about it.
- 256To the extent that the algorithms are transparent to third parties, of course. Yet, even detecting bias within a system can be less straightforward than may initially appear. See Sam Corbett-Davies, et al, Algorithmic Decision Making and the Cost of Fairness *6 (arXiv.org, June 10, 2017), archived at http://perma.cc/9D3L-PWJT (pushing back on Julia Angwin’s claim that the COMPAS criminal sentencing algorithm was biased). See note 43.
- 257See notes 116–25 and accompanying text. See also, for example, New Zealand Passport Robot Tells Applicant of Asian Descent to Open Eyes (Reuters, Dec 7, 2016), archived at http://perma.cc/47XS-HJXT (reporting on facial recognition software failure that resulted from an evidently unrepresentative training set); Natasha Singer, Amazon Is Pushing Facial Technology That a Study Says Could Be Biased (NY Times, Jan 25, 2019), archived at http://perma.cc/6UH5-85XG (reporting that Amazon’s facial-recognition technology doesn’t work well with women and particularly women of color).
- 258See Dyfed Loesche, The Prison Gender Gap (Statista, Oct 23, 2017), archived at http://perma.cc/E2PQ-LJVM.
- 259Mariel Alper, Matthew R. Durose, and Joshua Markman, 2018 Update on Prisoner Recidivism: A 9-Year Follow-Up Period (2005–2014) *6 (Bureau of Justice Statistics, May 2018), archived at http://perma.cc/26Y3-BAFP.
- 260See Matthew DeMichele, et al, The Public Safety Assessment: A Re-validation and Assessment of Predictive Utility and Differential Prediction by Race and Gender in Kentucky *52–53 (Arnold Foundation, Apr 30, 2018), archived at http://perma.cc/HN9D-ER5R.
- 261A different issue arises when the robot is itself the injured party. What would it mean to put a robot in its rightful position? What that likely means, at least until we recognize robot rights, is putting the robot’s owner or operator in its rightful position. While there are issues here, we think they are likely to be more straightforward than most of the ones we have discussed. If a robot is damaged or destroyed through negligence or vandalism, we will normally treat that as we would damage to any other property. It’s easy enough to replace parts for pre-programmed bots, but if the algorithms learned from unique, one-off interactions and cannot be recovered, robots might not be so easy to replace. Hopefully, emergent AI will be backed up regularly, though, so it could still be replaced.
We can imagine deliberately unique robots, though. Tay, for instance, was a unique chat bot deployed by Microsoft. Like too many people, when exposed to the Internet, Tay quickly became a fascist. See notes 97–101. When Microsoft shut her down, her “learning” was gone and could not be replaced. Few would lament that in this specific case, but we can imagine valuation difficulties if a tortious or malicious act destroys a unique AI personality.
- 262The notion of “unreasonableness” is captured by both negligence (B < PL) and product liability’s risk-utility test (as distinct from forms of truly “strict” liability). For an in-depth analysis of product liability’s risk-utility doctrine, see generally Geistfeld, 105 Cal L Rev 1611 (cited in note 143).
- 263See United States v Carroll Towing Co, 159 F2d 169, 171–73 (2d Cir 1947).
- 264See Complaint for Damages, Willhelm Nilsson v General Motors LLC, 4:18-cv-00471-KAW *3–4 (ND Cal filed Jan 22, 2018). Some have endorsed the existing negligence standard. See, for example, The Future Computed: Artificial Intelligence and Its Role in Society *85 (Microsoft 2018), archived at http://perma.cc/K8JU-4LRQ.
- 265See generally Eric Talley, Automatorts: How Should Accident Law Adapt to Autonomous Vehicles? Lessons from Law and Economics (Hoover IP2 Working Paper No 19002, Jan 8, 2019), archived at http://perma.cc/AXX2-QPJP.
- 266Although, NHTSA’s track record here has been called into question by numerous scholars. See, for example, Jerry L. Mashaw and David L. Harfst, From Command and Control to Collaboration and Deference: The Transformation of Auto Safety Regulation, 34 Yale J Reg 167, 266–73 (2017) (noting that “[t]o date, NHTSA has approached the regulation of [highly automated vehicle] technologies very gingerly, to say the least”).
- 267Omri Rachum-Twaig suggests an intermediate approach between tort law and command-and-control regulation—a series of not-so-safe harbors, in which compliance with certain regulatory rules will not avoid liability but will put a robot into the normal tort system, while failure to comply will lead to automatic liability. See generally Omri Rachum-Twaig, Whose Robot Is It Anyway? Liability for Artificial-Intelligence-Based Robots, 2020 U Ill L Rev (forthcoming), archived at http://perma.cc/3NBT-T5FR.
- 268See generally, for example, Richard A. Epstein, Liability Rules in the Internet of Things: Why Traditional Legal Relations Encourage Modern Technological Innovation (Hoover IP2 Working Paper No 19003, Jan 8, 2019), archived at http://perma.cc/G6AV-6HVM.
- 269Professor Bryan Choi refers to this as “a standard of ‘crashproof’ code.” See Choi, 94 Wash L Rev at 39 (cited in note 102).
- 270See Bryant Walker Smith, The Trolley and the Pinto: Cost-Benefit Analysis in Automated Driving and Other Cyber-Physical Systems, 4 Tex A&M L Rev 197, 205–07 (2017) (discussing the potentially problematic reaction of juries to explicit efforts to trade off safety against the value of human lives).
- 271As clarified in note 167, we don’t necessarily envision remedies law seeking to assign specific costs to all conceivable outcomes. Though in some situations, this may be appropriate.
- 272For a discussion of the difficult problems that evaluating “machine testimony” present in our court system, see generally Andrea Roth, Machine Testimony, 126 Yale L J 1972 (2017).
- 273See, for example, Francesca M. Favarò, et al, Examining Accident Reports Involving Autonomous Vehicles in California, 12 PLoS One 1, 5–16 (2017) (reconstructing autonomous vehicle accidents through the data collected by onboard recording devices); Bryan Casey, Robot Ipsa Loquitur, 107 Georgetown L J *14–16 (forthcoming 2019), archived at http://perma.cc/B2RM-QESL.
- 274For an argument for a strict liability regime for accidents involving autonomous vehicles, see Adam Rosenberg, Strict Liability: Imagining a Legal Framework for Autonomous Vehicles, 20 Tulane J Tech & Intel Prop 205, 218–22 (2017). See also Steven Shavell, A Fundamental Error in the Law of Torts: The Restriction of Strict Liability to Uncommon Activities (working paper, 2018), archived at http://perma.cc/UXX9-B896; Kyle Colonna, Autonomous Cars and Tort Liability, 4 Case W Res J L Tech & Internet 81, 118–30 (2012) (recommending a two-tiered system of liability for autonomous vehicles). But see generally Ryan Abbott, The Reasonable Computer: Disrupting the Paradigm of Tort Liability, 86 Geo Wash L Rev 1 (2018) (arguing that computers should be subject to negligence rather than strict liability).
- 275Some have argued this should incline us toward some sort of a no-fault system as self-driving cars and self-flying planes increasingly share space with their human-operated counterparts. See, for example, Abraham and Rabin, 105 Va L Rev at *23–50 (cited in note 143) (arguing for a no-fault accident compensation regime once autonomous vehicles have reached sufficient market penetration); Katharine Wallis, New Zealand’s 2005 “No-Fault” Compensation Reforms and Medical Professional Accountability for Harm, 126 New Zealand Med J 33, 33–35 (2013) (detailing New Zealand’s “taxpayer funded accident compensation scheme to provide compensation for medical injury”); Rachum-Twaig, 2020 U Ill L Rev at *31–38 (cited in note 267) (discussing a similar regulatory system).
- 276For a suggestion along these lines, see Geistfeld, 105 Cal L Rev at 1634–35, 1660–69 (cited in note 143); Rachum-Twaig, 2020 U Ill L Rev at *31 (cited in note 267). Professor Mark Geistfeld would leave an exception for cars that were designed or manufactured defectively and for those that were hacked.
- 277Choi, 94 Wash L Rev at *86–103 (cited in note 102) (arguing for such an approach).
- 278See Ryan Beene, It’s No Use Honking. The Robot at the Wheel Can’t Hear You (Bloomberg Law, Oct 10, 2017), online at http://bit.ly/2uq0lSa (visited Apr 9, 2019) (Perma archive unavailable) (reviewing California crash reports and documenting the prevalence of those sorts of accidents).
- 279For a more detailed discussion of these issues, see generally Casey, 107 Georgetown L J (cited in note 273).
- 280Even then we might want to assess liability against the autonomous vehicle that is using an outdated or less-safe algorithm, to encourage the development of better safety technology in autonomous vehicles.
- 281See Casey, 107 Georgetown L J at *48–62 (cited in note 273).
- 282See Karni Chagal-Feferkorn, The Reasonable Algorithm, 2018 U Ill J L Tech & Pol 111, 121–22; Ryan Abbot, The Reasonable Robot: Autonomous Machines and the Law (University of Surrey School of Law, 2018), online at http://youtu.be/2ktf0hQ7yMg (visited Apr 9, 2019) (Perma archive unavailable); Casey, 107 Georgetown L J at *48–62 (cited in note 273).
- 283On shutting down robots, see Part III.D.
- 284See generally Aaron Chalfin and Justin McCrary, Criminal Deterrence: A Review of the Literature, 55 J Econ Lit 5 (2017) (finding limited evidence “that crime responds to the severity of criminal sanctions”); Menusch Khadjavi, On the Interaction of Deterrence and Emotions, 31 J L Econ & Org 287, 298–307 (2015) (examining the influence of human emotion on different deterrent effects).
- 285See Mulligan, 69 SC L Rev at 585–89 (cited in note 6).
- 286The recent controversy that erupted over a Stanford University swimmer’s six-month sentence for sexual assault provides just one example. See Maggie Astor, California Voters Remove Judge Aaron Persky, Who Gave a 6-Month Sentence for Sexual Assault (NY Times, June 6, 2018), archived at http://perma.cc/4ETL-KY23.
- 287For instance, we have relaxed the rules of causation in remedies law in order to compensate indirect victims of large oil spills. See 33 USC §§ 2701–02.
- 288One day, we may even want to go further by putting robots in charge of remedies decisions. See generally Eugene Volokh, Chief Justice Robots, 68 Duke L J 1135 (2019) (examining how AI-assisted judges and juries could shape future jurisprudence).
- 289Compensation might be inadequate for various reasons. For example, courts cut off liability with proximate cause before we have traced all the harm from wrongful acts. See, for example, Pruitt v Allied Chemical Corp, 523 F Supp 975, 978–80 (ED Va 1981) (denying relief for indirect injury from pollution); Lemley, 103 Iowa L Rev at 253–54 (cited in note 200) (describing the limits on liability in the patent law context). We are bad at valuing pain and suffering and do so in idiosyncratic ways that will sometimes undercompensate plaintiffs. And we have imposed caps on liability in many circumstances that undercompensate for actual injuries. Michael S. Kang, Comment, Don’t Tell Juries about Statutory Damage Caps: The Merits of Nondisclosure, 66 U Chi L Rev 469, 470 (1999) (noting “[i]t has become increasingly common for Congress and state legislatures to enact statutory limits on the amount of money damages that a plaintiff can recover in a jury trial”). But if we are not compensating plaintiffs properly, the solution is to compensate them properly, not to add a damages multiplier to awards whether or not they are actually compensatory.
- 290See notes 226–32 and accompanying text (discussing this problem).
- 291See generally, for example, Missouri v Jenkins, 515 US 70 (1995); Langdon v Google, Inc, 474 F Supp 2d 622 (D Del 2007).
- 292See note 245 and accompanying text (discussing this phenomenon in the antitrust context).
- 293See FRCP 65(d)(1) (“Every order granting an injunction and every restraining order must (A) state the reasons why it [was] issued; (B) state its terms specifically; and (C) describe in reasonable detail . . . the act or acts restrained or required.”); Mitchell v Seaboard System Railroad, 883 F2d 451, 454 (6th Cir 1989) (vacating an injunction that simply forbade violating Title VII).
- 294See Ryan Beene, Self-Driving Cars Don’t Need Rules Yet, U.S. Regulator Says, (Bloomberg Law, July 12, 2018), online at http://bit.ly/2TUEHEI (visited Apr 19, 2019) (Perma archive unavailable). See also Adam Thierer, Andrea Castillo O’Sullivan, and Raymond Russell, Artificial Intelligence and Public Policy *41–48 (Mercatus Research, Aug 23, 2017), archived at http://perma.cc/2PC3-7TZT (arguing for a period of “permissionless innovation” to help AI systems develop).
- 295See Madeline Lamo and Ryan Calo, Regulating Bot Speech *1 (working paper, 2018), archived at http://perma.cc/UBS9-H7L7 (arguing that we should “proceed with caution in regulating bots, lest we inadvertently curtail a new, unfolding form of expression”).
- 296See FRCP 65(d)(1); Burton v City of Belle Glade, 178 F3d 1175, 1201 (11th Cir 1999) (rejecting an injunction that simply forbade future discrimination); Hughey v JMS Development Corp, 78 F3d 1523, 1531–32 (11th Cir 1996) (overturning injunction that forbade discharge of waste in violation of the Clean Water Act).
- 297See, for example, Jenkins, 515 US at 86–103; Hutto v Finney, 437 US 678, 685–88 (1978); Lewis v Casey, 518 US 343, 357–60 (1996) (overturning the district court’s systemwide injunction against the Arizona Department of Corrections because the remedy improperly exceeded the injuries established by the plaintiff class); United States v Virginia, 518 US 515, 546–58 (1996) (holding that the Virginia Military Institute, which categorically excluded women from enrollment, could not remedy its constitutional violation by creating a separate program for women because it would not have closely fit the injury produced by the violation).
- 298See, for example, Lewis, 518 US at 347.
- 299For a discussion of the issues that arise in structural injunction cases, see Laycock, Modern American Remedies at 310–42 (cited in note 145).
- 300Dana Hull and Tim Smith, Tesla Driver Died Using Autopilot, with Hands off Steering Wheel (Bloomberg, Mar 30, 2018), archived at http://perma.cc/RK7Z-NWSM (noting that Tesla “continuously improves [its “Autopilot” technology] via over-the-air software updates”). Courts in IP cases have sometimes ordered defendants to change not only new products they sold but to push out updates that deleted infringing functionality from products already in the field. See, for example, TiVo v Echostar, 446 F Supp 2d 664, 670–71 (ED Tex 2006); Dennis Crouch, Injunction Granted to TiVo; Injunction Denied in Favor of Toyota (PatentlyO, Aug 18, 2006), archived at http://perma.cc/5VMY-2HWA; Universal City Studios Productions LLLP v TickBox TV LLC, 2018 WL 1568698, *1, 13–15 (CD Cal). For an argument for “government-to-robot” enforcement of laws and regulations that would automatically bring all robots into compliance, whether or not they were part of a lawsuit, see generally Susan C. Morse, Government-to-Robot Enforcement, 2019 U Ill L Rev (forthcoming), archived at http://perma.cc/8HZB-L7TN.
- 301See Hassell v Bird, 420 P3d 776, 794 (Cal 2018) (Kruger concurring) (stressing that “the courts’ power to order people to do (or to refrain from doing) things is generally limited to the parties in the case”). There is a robust debate today about the propriety of nationwide injunctions and whether they allow sufficient percolation among courts. The ability to retroactively implement orders to cover existing products makes that debate all the more important.
- 302Though often they will overlap. The robot that used bitcoin to buy drugs was subject to criminal, not civil, penalties in Switzerland. See Kharpal, Robot with $100 Bitcoin Buys Drugs (cited in note 73).
- 303See generally Alexander Volokh, n Guilty Men, 146 U Pa L Rev 173 (1997) (providing evidence of widespread support for this sentiment throughout history).
- 304John D. Bessler, Tinkering around the Edges: The Supreme Court’s Death Penalty Jurisprudence, 49 Am Crim L Rev 1913, 1919–33 (2012) (discussing limitations surrounding imposition of the death penalty).
- 305For a suggestion that robots can be held liable for crimes just as people can, see Gabriel Hallevy, Dangerous Robots—Artificial Intelligence vs. Human Intelligence *10–34 (working paper, Feb 2018), archived at http://perma.cc/T6FD-QDZT.
- 306We distinguish this from the case in which humans use robots to commit crimes. A human can use a drone to fire missiles, for instance, or to spy on people. See Amanda McAllister, Stranger Than Science Fiction: The Rise of A.I. Interrogation in the Dawn of Autonomous Robots and the Need for an Additional Protocol to the U.N. Convention against Torture, 101 Minn L Rev 2527, 2565–70 (2017) (proffering guidelines for regulating use of autonomous weapons). If the robot is the instrument of the crime but not its cause, it is the human, not the robot, that should face criminal penalties.
- 307See, for example, Perez, Microsoft Silences Its New A.I. Bot Tay (cited in note 101).
- 308For a discussion of the remarkable story of Walter Ego and its beheading, see Calo, Robots in American Law *10–11 (cited in note 8).
- 309See Safia Gray Hussain, Attacking the Dog-Bite Epidemic: Why Breed-Specific Legislation Won’t Solve the Dangerous-Dog Dilemma, 74 Fordham L Rev 2847, 2854–56 (2006). See also Part II.D.1.
- 310These often go by the name “genetic algorithms.”
- 311See Mark A. Lemley and R. Anthony Reese, Reducing Digital Copyright Infringement without Restricting Innovation, 56 Stan L Rev 1345, 1355–56 (2004) (defining “dual-use” technologies as “products or services that can be used by the consumer in noninfringing ways but that can also be used to infringe copyright”). See also, for example, Mark A. Lemley and Philip J. Weiser, Should Property or Liability Rules Govern Information?, 85 Tex L Rev 783, 802 (2007) (giving an example of a copyright infringement dispute involving Napster’s peer-to-peer exchanging software, a dual-use technology).
- 312Particularly because market forces and conventional remedies will often obviate the need for formal legal interventions of the type envisioned here.
- 313We could, of course, shut down only the particular robot that caused harm while leaving other robots running the same code. But that wouldn’t make any sense as a logical matter. If we did so it would presumably reflect the purest form of punching the particular robot that harmed you. So when we speak of “shutting down” a robot, we mean that all of its underlying code is actually destroyed, as opposed to one copy of it. That doesn’t mean the company that sells it can’t sell robots at all; they may have different robots with different learning patterns that won’t be affected. But all robots running that code would presumably suffer the same fate.
One complication is that AI systems increasingly function as “hive minds”: fleets of systems that learn based on individual experience but that share data and update collective decision-making. See David Sedgwick, “Hive Mind” Could Help Cars Expect the Unexpected (Automotive News, Jan 12, 2015), online at http://www.autonews.com/article/20150112/OEM06/301129972/hive-mind-could-help-cars-expect-the-unexpected (visited at Apr 9, 2019) (Perma archive unavailable). Tesla’s self-driving cars, for instance, learn from each other’s experience, improving their reaction to situations that a different car has encountered. All the robots in a hive mind gone wrong would have to be shut down unless the faulty input could be identified and isolated.
There may be circumstances in which robots fail due to a manufacturing defect that is limited to one robot (or one batch) rather than a problem with the code. In those cases, presumably only those flawed robots would be at risk of the robot death penalty. But those instances are likely to be less common and less interesting than those involving code.
- 314Unlike the human death penalty, it is possible the robot death penalty could be reversed. Unless all copies of code are actually destroyed, it might be possible to rehabilitate a broken robot and put it back into service with altered code. A simpler version of that happens all the time today, as software companies push out patched code to fix problems with their prior versions. That sort of versioning will happen regularly with robots, too. But it will get harder to do with confidence as the robot learns on its own and begins to make its own decisions, decisions its designers may not understand. Rather than patching a self-learning robot that is doing bad things, the better alternative may be to roll back the code to a time when it was working and modify it so the next version of the robot doesn’t follow the same problematic path.
- 315See Crouch, Injunction Granted to TiVo (cited in note 300).
- 316See, for example, Universal City Studios Productions LLLP, 2018 WL 1568698 at *1. It appears that the court is poised to order a device maker to use its software update mechanism to remove functionality and content from users’ devices.
- 317See Peter M. Asaro, A Body to Kick, but Still No Soul to Damn: Legal Perspectives on Robotics, in Patrick Lin, Keith Abney, and George A. Bekey, eds, Robot Ethics: The Ethical and Social Implications of Robotics 150, 158–60 (MIT 2012). See also note 174 and accompanying text.
- 318See generally Citizens United v Federal Election Commission, 558 US 310 (2010).
- 319See Hussain, 74 Fordham L Rev at 2856 (cited in note 309). See also generally Christopher Seps, Animal Law Evolution: Treating Pets as Persons in Tort and Custody Disputes, 2010 U Ill L Rev 1339. Animals have only limited standing to bring cases, but they sometimes can. See, for example, Naruto v Slater, 888 F3d 418, 424–25 (9th Cir 2018) (finding that a crested macaque alleged facts sufficient to establish Article III standing because it was the apparent author and owner of selfies it took and may have suffered legally cognizable harms); Cetacean Community v Bush, 386 F3d 1169, 1175 (9th Cir 2004) (stating that mere fact that plaintiffs were animals did not rule out possibility of standing). But the law also refuses to treat animals as anything other than property in many instances. See, for example, Johnson v Douglas, 187 Misc 2d 509, 510–11 (NY Sup 2001) (refusing to allow emotional distress damages because dog was considered personal property).
- 320See Emily Reynolds, The Agony of Sophia, the World’s First Robot Citizen Condemned to a Lifeless Career in Marketing (Wired, June 1, 2018), archived at http://perma.cc/45S5-XEFK (weighing the implications of extending legal personhood to a greater number of robots in the future); The Global Race to Robot Law: 2nd Place, South Korea (Robotics Business Review, Sept 24, 2013), archived at http://perma.cc/2EUX-US3T (discussing South Korea’s “Robot Ethics Charter”).
- 321See Charles Stross, Dude, You Broke the Future! (Charlie’s Diary, Dec 2017), archived at http://perma.cc/V3EA-5NCX.
- 322Id.
- 323Lynn M. LoPucki, Algorithmic Entities, 95 Wash U L Rev 887, 887, 897–901 (2018). See also Shawn Bayern, The Implications of Modern Business-Entity Law for the Regulation of Autonomous Systems, 19 Stan Tech L Rev 93, 105–08 (2015); Bayern, 108 Nw U L Rev at 1495 (cited in note 172).
- 324Consider Asimov’s three laws of robotics, Isaac Asimov, Runaround, in I, Robot 41, 53 (Ballantine 1950), which would allow any person to kill a robot for any reason. Isaac Asimov clearly never anticipated Reddit. Trying to implement the three laws of robotics would leave the world strewn with the carcasses of robots killed by griefers. For an effort to write three human “laws” to regulate robots, see generally Jack M. Balkin, The Three Laws of Robotics in the Age of Big Data, 78 Ohio St L J 1217 (2017).
- 325For an argument that current methods of punishing corporations are ineffective and that corporations should face organizational remedies—the equivalent of rewriting their “code”—see Mihailis E. Diamantis, How to Punish a Corporation (working paper, 2018), archived at http://perma.cc/632P-432H.
- 326We are by no means the first to have advanced this line of argument. See, for example, William S. Laufer, Corporate Culpability and the Limits of Law, 6 Bus Ethics Q 311, 312–14 (1996).
- 327See Shavell, A Fundamental Error in the Law of Torts *25–34 (cited in note 274). This does not mean, however, that we don’t need laws. Some have suggested that we won’t need rules or standards in the future because we can just rely on machine judgment to decide what the right thing to do is in any specific situation. See generally Anthony J. Casey and Anthony Niblett, The Death of Rules and Standards, 92 Ind L J 1401 (2017). For the reasons we explained in Part I, we think that highly unlikely. Robots will cause all sorts of harm the legal system will want to remedy. See generally Dan L. Burk, Algorithmic Fair Use, 86 U Chi L Rev 283 (2019) (explaining why algorithms won’t effectively replace standards in many cases).
- 328Lemley, not Casey.
- 329See Aaron McKnight, Jury Nullification as a Tool to Balance the Demands of Law and Justice, 2013 BYU L Rev 1103, 1129–31 (discussing this phenomenon with respect to jury nullification).