The Chilling Effect of Governance-by-Data on Data Markets
Big data has become an important resource not only for commerce but also for governance. Governance-by-data seeks to take advantage of the bulk of data collected by private firms to make law enforcement more efficient. It can take many forms, including setting enforcement priorities, affecting methods of proof, and even changing the content of legal norms. For instance, car manufacturers can use real-time data on the driving habits of drivers to learn how their cars respond to different driving patterns. If shared with the government, the same data can be used to enforce speed limits or even to craft personalized speed limits for each driver.
The sharing of data for the purpose of law enforcement raises obvious concerns for civil liberties. Indeed, over the past two decades, scholars have focused on the risks arising from such data sharing for privacy and freedom. So far, however, the literature has generally overlooked the implications of such dual use of data for data markets and data-driven innovation.
In this Essay, we argue that governance-by-data may create chilling effects that could distort data collection and data-driven innovation. We challenge the assumptions that incentives to collect data are a given and that firms will continue to collect data notwithstanding governmental access to such data. We show that, in some instances, an inverse relationship exists between incentives for collecting data and sharing it for the purpose of governance. Moreover, the incentives of data subjects to allow the collection of data by private entities might also change, thereby potentially affecting the efficiency of data-driven markets and, subsequently, data-driven innovation. As a result, data markets might not provide sufficient and adequate data to support digital governance. This, in turn, might significantly affect welfare.
Introduction
Big data has become an important resource not only in the commercial sphere but also in the legal one. Governance-by-data can take many forms, including setting enforcement priorities, affecting methods of proof, and even changing the content of legal norms. Private entities play a central role in collecting and analyzing such data. Indeed, successful implementation of governance-by-data may depend on the potential dual use of data as a commercial asset, generated by the private sector, and as an intrinsic measure of governance.1
The interplay between private and public uses of the same data has important implications for social welfare. Over the past two decades, scholars have identified the growing role private firms play in facilitating governmental access to data,2 analyzed the incentives of private companies to collaborate with governmental surveillance,3 and explored the implications of such data sharing for civil liberties.4
This literature, however, has generally overlooked the implications of the dual use of data for data markets. The current literature largely assumes that incentives to collect data are a given and that firms will continue to collect data while simply erecting higher barriers to governmental access to such data. We challenge this assumption: once governance-by-data is introduced, incentives for data collection might change. But more fundamentally, incentives of data subjects to allow private entities to collect their data might also change, thereby potentially affecting the efficiency of data-driven markets and data-driven innovation. This, in turn, might significantly affect welfare. Furthermore, granting government access to privately collected data might harm the very foundation on which governance-by-data relies. This dynamic effect must be recognized and analyzed before we can make informed choices and ensure that governmental governance-by-data indeed increases welfare.
To elaborate on this claim, this Essay focuses on one form of governance-by-data, personalized law, recognizing that many arguments about it have relevance for other forms of governance-by-data as well. Personalized law seeks to take advantage of technological advances in data collection and data science, which allow data to be transferred, stored, organized, and analyzed in an efficient and timely manner, in order to tailor legal norms to individuals. For instance, rather than setting a single speed limit that applies to all drivers, speed limits might be personally tailored to individual drivers based on their experience, driving history, or real-time road conditions. Or disabled parking permits could be issued to individuals based on relevant temporary or permanent health conditions or family circumstances (for example, driving young children). Such tailored norms could be embedded in the digital infrastructure (such as autonomous cars, smart parking facilities, and roads) and could be individually applied in real time by enabling parking, issuing a ticket, or even remotely disabling a car following a warning.5
Personalized law may increase efficiency by improving law enforcement, reducing under- or overinclusive risk avoidance mechanisms, and reducing institutionalized discrimination.6 At the same time, however, personalized law may undermine important values, raising concerns regarding privacy, equality under the law, and civil liberties.7
To fulfill its promises, personalized law requires governmental access to its main inputs, namely relevant data and algorithms that can turn that data into a governing tool. Indeed, the more personalized the law, the more accurate and personal the data required. This implies a change not only in the volume of data needed for tailoring the legal norm but also in its quality, including its variety, velocity, and veracity.8
While some of this data can be collected from government-controlled data sources (such as speed cameras), the bulk of the data is likely to be collected by private firms (for example, data on driving patterns collected from sensors in cars, operated by the car manufacturers; locational data collected by mobile phone applications; or real-time health data generated by wearables).
Privately produced data is a primary resource, asset, and product of the digital economy. Its high commercial value arises from the fact that it allows for regularized customization of decision-making, thereby reducing risk and improving performance. Among other things, it enables firms to make more profitable investment, pricing, and marketing decisions and to create new or improved products and services in response to individual demand. Accordingly, numerous firms are investing in collecting, organizing, and analyzing data or in creating products, services, and technologies that rely on such data, giving rise to data capitalism.9
Can personalized laws, which heavily rely on data collected by private firms, coexist with a vibrant market for data collection and the resultant data-driven innovation?
This Essay argues that, although data is nonrivalrous and its costs of transfer are usually low, collecting, organizing, storing, and sharing some types of data could be costly.10 As a result, some data intermediaries might enjoy market power. Therefore, it cannot be assumed that data will be shared with the government at low, competitive prices. This consideration affects the feasibility and efficiency of governance-by-data.
More importantly, we argue that governance-by-data may create an inherent tension and sometimes even a clash with data capitalism. Once introduced, it may affect the markets for data on which it relies, thereby changing the current status quo. We identify and analyze four dimensions in which governance-by-data may change the dynamics of data-driven markets: 1) the quantity of data collected; 2) the quality of data collected; 3) the use of efficient technologies that build on ongoing analysis of data; and, resultantly and most importantly, 4) data-driven innovation. These effects, in turn, are likely to affect the efficiency of data-driven markets as well as the feasibility and efficacy of personalized laws that depend on such data.
These effects result from the fact that the introduction of governance-by-data may lead to self-applied limitations on data provision, collection, and sharing; chilling effects in data markets; and knock-on domino effects in markets for products and services that rely on such data. Some data subjects—whether private individuals or legal entities—might limit their use of devices that collect data or provide inaccurate, partial, or “noisy” data, thereby affecting the quantity and quality of data available. This, in turn, may lower the incentives of data collectors to share data with the government or even to generate data that may be used for the purpose of governance in the first place.11 Such impediments to extracting data or erosion in the quality of data may result in downgrading data-driven innovation and may negatively affect dynamic efficiency, which is the main engine of modern economies, thereby possibly reducing social welfare.
The Essay proceeds as follows: Part I sets the stage by briefly introducing personalized law as a form of governance-by-data and the necessary conditions for its efficient functioning. It also briefly explores the characteristics of markets for data. Part II analyzes a potential source of tension between data capitalism and personalized law: the price of the data. Part III focuses on a more significant manifestation of this tension: distortions in the operation of data markets, including in the quantity and quality of data collected, and the implications for the use of data-based devices and for data-driven innovation. The Conclusion briefly explores some regulatory and technological tools to address these inherent tensions.
I. Personalized Law and Data Resources
Personalized law seeks to take advantage of the proliferation of data to make law enforcement more efficient. For instance, modern cars can collect real-time data on driving patterns. Car manufacturers can use this data to learn how their cars respond to different driving patterns. If shared with the government, the same data can be used to craft personalized speed limits for each driver. To take another example, firms collect data on individuals’ risk levels. Such data can be used to price products (for example, insurance) or to customize marketing (for example, fast cars). The same data can be used to complete an incomplete contract signed by the individual, which requires an assessment of her risk level.
Personalized laws therefore seek to utilize fine-grained data on individuals in order to develop and apply personally tailored legal norms. This Part explores the sources and characteristics of such data.
A. Sources of Data
Two distinct sources of data may be needed to employ personalized law: data collected by the government and data collected by private firms. Data from the two sources does not always overlap. For example, the government collects data from speeding cameras, censuses, and tax returns. Some of this data may be unique, and some may be similar to that collected by private firms. The data collected by the government may not always be sufficient to craft personalized laws.12 In these cases, the government might invest resources in collecting such data or opt to acquire data collected by private firms.13 Indeed, digital communication, data collection, and data storage are currently dominated by private firms. In some cases, a combination of data from governmental and private sources might be necessary.
B. Markets for Data
Data is an important driver in today’s economy. The growth in computing and storage power combined with increased and more efficient internet access have spurred the advent of the digital economy and enabled the rise of business models based on the collection and processing of large quantities of data (big data).14
The ability to store, arrange, and analyze data using sophisticated algorithms in order to gain insights is what gives data its enormous financial value and power.15 The analysis of data allows for regularized customization of decision-making, thereby reducing risk and improving performance. Big data also enables the introduction of new products, such as self-driving cars and smart cities, thereby generating large gains for business, consumers, and society as a whole.16
Personal data plays a key role in this economy. It is used to find correlations that enable prediction of overall trends as well as individual preferences and behavior. It can also be used to create a “digital profile” for each individual, which could then be used to improve personalized products and services or for microtargeted advertising.17
Data markets consist of three main links18 along the data value chain, depicted in Figure 1: collection; synthesis and analysis; and use.
Figure 1: The Data Value Chain
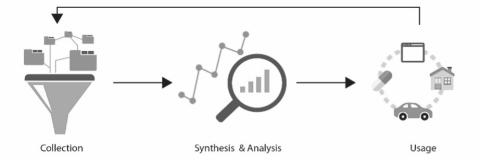
Collection relates to the extraction of the data and its datafication, namely the recording, aggregation, and organization of information into a form that can be used for data mining.19 Synthesis and analysis relate to the integration of different types of data and to the analytical processing of data in order to find correlations. It transforms the raw data into meaningful information. The last link, use, involves utilizing data-based information for prediction and decision-making in relevant markets. The outputs of these activities may include improved or innovative processes, products, or services. This value chain also has a dynamic internal reciprocal dimension, in which data regarding the success of the algorithm’s past predictions is collected and used to “teach” the algorithm to be more accurate so that it can make better predictions in the future.20 The characteristics of data and the markets for its collection, analysis, and use affect how it is provided.
Sources of data vary. One major source, which is characterized by fierce competition, is users’ online attention.21 Several business models for collecting such data have emerged, some of which are based on an implicit or explicit exchange with data subjects: users allow firms to access (some of) their private data in exchange for various benefits (for example, apps, content, services).22 Some firms have developed into “mega” data collectors, with direct and significant access to digital users (for example, Google, Facebook, and Amazon).23 Some data is collected as a by-product of other productive activities, such as sensors embedded in “things” (for example, cars, appliances, wearables, and digital butlers(.
Some types of data are collected by numerous firms at low cost (for example, smartphone users’ location data). Moreover, similar data can be collected from different sources (for example, location data can be collected from wearables or smartphones).24 Yet the costs of data collection are not always low, and the markets for it are not always competitive. As Professors Daniel Rubinfeld and Michal Gal show, markets for certain types of data are characterized by high entry barriers.25 Some of these barriers reflect exclusive access points (for example, patient data collected by doctors) or the point in time that a firm started gathering data (for example, a collection of aerial maps before a natural disaster). Barriers to competition over data may also arise from scale and scope economies in data collection, organization, storage, or analysis;26 from network effects (for example, Facebook and Yelp);27 from legal limitations on data transfer (for example, sharing a person’s medical history without consent);28 from lock-in and switching costs when past data is important;29 and from barriers to data compatibility and interoperability.30 Fierce competition over users’ attention may increase the need to create costly products or services that capture such attention. Finally, when data is a by-product of other activities, the collector must engage in the relevant activities.31
The link in the data value chain consisting of synthesis and analysis is often characterized by economies of scale and scope. The information that can be gleaned from data is positively correlated with the attributes of the data collected (volume, velocity, variety, and veracity),32 at least up to a point.33 Thus, identifying patterns, generating predictions, and promptly adapting to rapidly changing circumstances often require the availability of large quantities of fresh, varied, and accurate data. Interestingly, and relevant to the analysis below, the correlations found in data analysis are not necessarily trivial.
Finally, with regard to use, the nonrivalrous nature of data implies that the same data can have a variety of uses.34 Moreover, if data exists in digital form, the marginal cost of sharing collected data with other entities can be very low. At the same time, data is often not fungible.35 Different types of data may be needed for different markets. For example, when velocity is of high importance, old data cannot serve as a sufficiently effective input.
How might data markets be affected by the introduction of personalized law? This is the focus of the next two Parts.
II. The Price of Data
The feasibility and efficacy of governance-by-data depend, among other things, on the price that the government must pay for access to data. The literature discussing personalized law tends to implicitly assume that markets for data are competitive and that its price would not create a barrier for accessing it. It is generally assumed that data produced by the private sector will be voluntarily shared with the government for governing purposes (hereinafter: “data sharing”) at low, competitive prices and that such dual use will increase overall efficiency and social welfare.36
In the real world, these conditions do not always hold, and the price for data may be high. As noted above, while the cost of sharing data, once collected, may be low, collecting data may involve high costs.37 Collection costs may increase further if personalized law requires data that is not regularly collected by the market due to differing incentives regarding the type of data collected, the frequency of collection, and ways of organizing the data. For example, if the costs of data collection or analysis are high, a firm might decide to sample the data only once a day. To apply personalized law, however, sampling might need to be more frequent. Or take data organization. Governance-by-data may require combining several sources of data and synchronizing data in order to ensure that similar legal principles apply to all. Creating data standards for interoperability and ensuring compliance with such standards may be costly.38 When all competitors incur high collection costs, the market price must cover such costs, at least in the long run.
In addition, some data collectors might enjoy significant comparative advantages, and even exclusivity, in the collection of certain types of data. This, in turn, might create significant market power over such data. Observe that the more specific the personalized law, the more specific the data need to be and, thus, the increased likelihood that it will not be available from many sources. In such cases, the price requested by private firms for sharing data might be high, reflecting their market power. Moreover, the price required might reflect and capture at least some of the positive externalities that the use of the data for personalized law will create on social welfare, thereby further increasing its price. Furthermore, when the application of personalized law requires data from several separate sources, an anticommons problem might arise.39 The price of data may also reflect lost commercial opportunities resulting from the use of data for governance purposes.40 Finally, it might reflect the loss data collectors might suffer from harm to their self-portrayal as “champions of user privacy in the face of government surveillance.”41
For the reasons we explore above, the price of data may be socially suboptimal, and some of the welfare benefits of personalized law could be lost. Observe, however, that the price paid by the government for data might increase incentives for data collection, which in turn could increase competition for the provision of data, at least in some markets. The price of data sharing might then be reduced.
The analysis thus far shows that the provision of personalized law might be suboptimal given the price that the government might need to pay for the data. When the price is too high, the government will simply not buy the data and will refrain from governance-by-data. Sometimes, however, this might not be optimal. This might be the case when the government is locked into a policy of personalized law (for example, it has applied it to some citizens and now needs to apply it to others), and the price for the data rises. This might happen, for example, if the government is technologically locked in to certain supplier(s) of data due to the length and costs of the process for vetting new data suppliers, first-mover advantages in creating interfaces for the use of data, and barriers relating to the inclusion of new types of data in existing systems.
III. Distortions in Data Markets
Personalized law seeks to take advantage of the proliferation of data to make law enforcement more efficient. Because data is nonrivalrous and its use by the government generally does not exhaust or harm its commercial utility, it is presumed that data sharing for law enforcement purposes would increase social welfare.
This Part challenges this presumption. It argues that data sharing may create chilling effects that could distort data and data-driven markets. While the previous Part generally assumes that the extent of data collection by private firms is largely a given, we show that, in some instances, an inverse relationship exists between incentives for collecting data and sharing it for the purpose of governance. As a result, data markets might not provide sufficient and adequate data to support personalized law.
Graphically, the argument that data sharing adds another dimension to market dynamics can be illustrated as follows:
Figure 2: The Effects of Data Sharing on the Data Value Chain
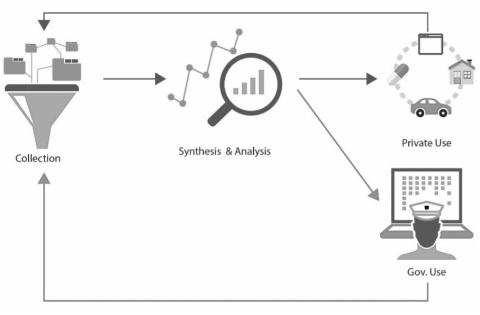
We first explore the potential consequences of personalized law on the conduct of data subjects. We then explore the likely effects of such consequences on data market dynamics, focusing on the quantity and quality of data as well as on the use of data-driven technologies and on data-driven innovation.
A. Effects on Data Subjects’ Conduct
Personalized law requires the sharing of data with the government on a scale and for purposes not previously known. One concern is that such data sharing will affect the incentives of data subjects to make their data available for collection and, resultantly, the ability and incentives of data collectors to collect data.
A fierce debate exists over whether surveillance by private firms affects people’s behavior. Behavioral studies point to a “privacy paradox”—that is, a gap between the high value people claim to place on privacy and their actual online behavior, which demonstrates that they are willing to disclose personal data for relatively small rewards.42 Yet there is little agreement on the conclusions to be drawn from these studies. Some privacy scholars explain this paradox by arguing that consumers lack sufficient information on potential risks involved in disclosing their personal data or hold misconceptions regarding data collection, the risks involved, and the existence of alternative options to data disclosure.43 Others argue, however, that individuals disclose personal data despite pronounced privacy concerns because they prefer immediate benefits over abstract potential risks in the future.44
Furthermore, surveillance has arguably become embedded in the modern ecosystem of an “always on” society, in which data is collected on an ongoing basis (for example, sensors in devices often record data continuously). Therefore, presumably, personalized law would simply add another layer of use of the data collected.
Is there any reason to believe that data subjects might change their behavior if the same data that is used by private firms were also used for governance-by-data?
While the effects on conduct of increased governmental surveillance for the purposes of personalized law have not, as yet, been studied, some rough indicators exist.45 The Edward Snowden revelations offer a classic example of the use of data collected by the private sector for governmental surveillance.46 Several studies found changes in users’ behavior following the Snowden revelations. For instance, Alex Marthews and Professor Catherine Tucker found decreased use of select sensitive terms in search queries following the Snowden revelations.47 Another study, conducted by Professor Jonathon Penney, points to a decline in traffic to privacy-sensitive Wikipedia articles.48
The question arises: How much can we learn from these studies? The observed changes in behavior might simply reflect users’ expectations that data collected by private firms will not be used for government surveillance. As argued by Professor Helen Nissenbaum, context matters, and data that was shared in one context cannot be shared in another without violating the “context-specific substantive norms” that delineate who collects the data, who it can be shared with, and under what circumstances.49 In this sense, it might be argued that the reaction to the Snowden revelations can be partly explained by the breach of trust produced by the disregard for context-specific norms.50 Such breaches are of course not at issue when the sharing of data is transparent and known beforehand.
We argue, however, that, even if data subjects are aware that their data will be accessed for purposes of personalized law, this awareness is still likely to transform their behavior. That is because the use of data for governance adds a new dimension to surveillance, affecting data subjects not only as consumers but also as citizens. Data collected on a data subject may carry concrete consequences related to law enforcement, affecting her legal rights or duties. Even critics who are skeptical that surveillance in and of itself has a chilling effect on behavior agree that a chilling effect may occur when surveillance increases the risk of negative consequences.51 This link between data analysis and sanctions or rewards by the state makes personalized law not simply an enforcement measure (seeking to tailor standards to each individual) but also an instrument that could actually shape the behavior of data subjects.52 Because tailor-made standards (for example, customized speed limits) could be determined based on data collected in other contexts (for example, social media), we may need to rethink assumptions regarding the conduct of data subjects when data is generated and collected.
The use of data for governance-by-data thus raises concerns that go beyond harm to privacy. Several factors may lie at the basis of such a differentiation. These can be classified into potential consequences of personalized laws and other intangible implications.
1. Potential consequences for rights and duties.
For users, the government’s use of data might be (perceived as) more harmful than a private firm’s. The most obvious harm is potentially increased law enforcement or higher legal burdens based on personal profiling. While law-abiding data subjects might benefit from such profiling, others could be harmed by it. For instance, if data collected on a data subject’s use of product reveals the tendency of its user toward negligence, and this fact significantly increases the level of care expected of her, she may be reluctant to enable the collection or the sharing of the relevant data with the government.53
Accordingly, data sharing adds another dimension to risks arising from the collection and use of personal data by private firms. For instance, Google and Amazon collect data on customers’ online reading habits and use it to better predict what users are interested in or what they might want to buy or see next. The same data can be used by governments, not only to investigate crimes or to detect people accessing extremist material who might threaten public safety54 but also to measure the reading performance of students and teachers. Such information could then be used to make decisions about a child’s future or a teacher’s promotion prospects.55 The prospect that one’s online reading habits might be used for governance potentially carries real risks. This risk is compounded by the uncertainty of what one’s data will be used for.
Thus, even for law-abiding data subjects, uncertainty about the correlations that data might reveal or the purposes for which the data might be used in the future could affect their perceptions of the harm that might result from the sharing of that data. Such uncertainty prevents data subjects from assessing the likelihood, size, and type of harm that could result from the use of their data.56 Consider a law-abiding citizen who might benefit from personalized laws (for example, higher speed limits). Should the law change (for example, prohibiting risk assessment scores based on gender), or should different correlations be found in the data, the same database may increase her legal liability in other areas. For instance, the maximal speed limit of a law-abiding data subject who benefited from higher speed limits might be lowered if correlation is found between risky driving and seemingly unrelated personality traits (for example, being disorganized as reflected in social media postings).57 This potential for harm, and therefore the incentive to avoid data collection, may be further strengthened by future technological advances that make personalized law governance processes automatic and quick.
The government’s monopoly over law enforcement is also a source of risk, raising fears of error or abuse. Inaccurate profiling may result in unjustifiably denying rights or increasing the legal duties imposed on a data subject. Such inaccuracies can arise through profiling based on partial data or through inaccurate analysis of the data.58 Inaccuracy can also result from path dependency in the analysis. Even if the data subject changes her conduct—for example, significantly improving her driving habits—it is unclear when this will be registered in the data. Think about a much simpler example that does not require sophisticated analysis—namely, the length of time it can currently take to change one’s credit rating. In such cases, data subjects might attempt to strategically avoid having certain actions recorded.
Another concern is the potential for abuse of data by government agencies for their own purposes—for example, by giving more weight in their algorithms to features that further one or another political goal. Indeed, the assumption that the state is a benevolent actor that strives to fulfill its goals for the welfare of all, free of political influences, is questionable. As analysis becomes more nuanced and data-dependent, it is more easily open to manipulation and abuse. This harm can partly be mitigated by explaining which features of the data actually played a role in determining the outcome.59 Yet a “transparency paradox” then arises: the transparency needed to verify that the government did not tinker with the data or the algorithms could make the data transparent to other actors in the market, thereby harming the commercial interests of the data collectors by reducing their comparative advantage.
The use of data for personalized law may also involve signaling, which may affect the incentives to share personal data. For example, if you are allowed to drive only thirty miles per hour where others are allowed to drive fifty, this could signal that you are reckless and affect the commercial or personal offers you receive. Thus, specific government profiling may create externalities in other spheres of life. Indeed, firms can lower costs by “free riding” on profiling performed by the government, at least those aspects of profiling that are observable to the public.
Finally, data sharing for the purpose of personalized law might increase incentives for identity theft or identity switching. An individual might seek to switch her records with those of another data subject (either with or without the latter’s approval) if it might make her eligible for more beneficial standards under a personalized law regime.60
2. Intangible implications.
The use of data for personalized law could also create intangible harms, which might in turn trigger negative reactions from the public.61 For instance, warrantless government access to personal data may be seen as a violation of civil liberties and inappropriate governmental intervention in the private sphere. What matters is not only what law is enforced but also how.
Data sharing with the government may also increase the perceived loss of control over one’s personal sphere.62 Interestingly, studies have shown that people are more willing to share personal data when they feel in control of that decision, regardless of whether that control is real or illusory.63 This implies that (perceptions of) loss of control over one’s data may reduce voluntary sharing. Indeed, the feeling of loss of control created by data sharing for governmental purposes could be even stronger than that created by the use of data by private companies. In the private sphere, people generally feel they have some level of choice: one can switch suppliers or possibly abstain from or limit the use of most products, even at some cost. It is far more difficult to change governments or to “switch off” their laws. Of course, to some extent, this reasoning is already outdated. When sensors record our every move, regardless of whether we take any particular voluntary action in the digital world (for example, searching), data collection is no longer dependent on our choice of whether to operate devices.64
Another intangible harm associated with the use of data for personalized law involves the experience of freedom. Personal data processing might limit the ability of data subjects to present different aspects of their persona in different circumstances. For example, an individual may be deliberately cautious and prudent in most areas of life while allowing herself to act recklessly in one area in which she cannot cause harm to others. An algorithm that treats data from all spheres of life similarly might give that individual a high risk score, which fails to reflect how she operates in most spheres of life. This negative externality, in turn, could encourage individuals to abandon or downplay traits that they wish others to see in different settings.65 Uncertainty about the weight assigned by the governance algorithm to different aspects of life can produce the same result. The consequence is that people may feel constrained in their ability to present themselves to the world as multifaceted selves.66
Some argue that reluctance to share data with the government might also be based on ideological grounds, protecting libertarian notions of privacy in order to prevent a “dystopian world of pervasive surveillance,” with all the attendant social and political evils.67
Finally, the loss of universality of rules applied may also carry psychological implications. The fact that a data subject may be subject to stricter legal requirements relative to others around her due to her personal traits—especially those that she cannot control—might create a sense of inferiority and also affect her social status.
B. Effects on Market Dynamics
Having canvassed the potential harms to data subjects from data sharing, the next question is: How may such harms affect the conduct of data subjects and data collectors? And how could they affect social welfare arising from the use of data for personalized law? We focus on four main effects: the quantity of data collected, its quality, the use of efficient data-collecting devices, and effects on innovation in data-driven markets.
1. Effects on the quantity of data collected.
A main concern resulting from the foregoing discussion is that less data will be collected. When data subjects have a choice whether to engage in a certain activity that is recorded or whether to use a device that collects data, they might refrain from such action or use. This, in turn, will reduce the quantity of data available. This effect is dependent, however, on whether collection and sharing are known to data subjects.
Data collectors, acknowledging data subjects’ potential reactions, could commit to not sharing the data they collect with the government.68 Microsoft and Apple, for example, used the Snowden revelations as a business opportunity to leverage a market for privacy and to market their products and services as secure from government interception.69 Apple has introduced encryption tools without keys so that it could not technologically share data if asked to do so. Indeed, in 2016, Apple publicly challenged a request by the FBI to enable government access to data on an iPhone despite the fact that the data was required for an investigation implicating national security, which is generally considered a justifiable reason for data sharing and part of the government’s prerogative.70 Other firms may use more subtle ways to limit data sharing, including the erection of technological barriers to data collection or sharing.
Even when firms follow self-imposed limitations on data sharing, data subjects might still be concerned due to incentive asymmetries between users and firms. This might be the case, for example, when a data collector is on its way out of the industry or when data subjects are not aware that the collector’s commitments have changed. Such self-imposed limitations are of less importance if data sharing is mandatory. However, then data subjects may opt for devices that cannot collect data or that collect less data, at least in some situations. When this is the case, manufacturers may offer such devices, at least if the costs from lost consumers are higher than the benefits from data collection.
Observe that individual decisions to limit data collection might not yield the most desirable social outcome. Indeed, individuals may choose to maximize their personal gains by withholding data while not considering what is beneficial to society as a whole. Their decisions could also be affected by collective action and free-riding problems, whereby each individual attempts to free ride on the data collected on others, which might lead to the creation of better products or services from which she could also benefit.
Of course, in some situations nonuse is not an option or could be extremely costly. This might be the case when insurance companies require data as a precondition for offering policies or when data can significantly reduce premiums.71 China’s social credit score presents an extreme example.72 Under this system, a combination of private and governmental data (such as defaults on fines) are used to create a social credit score for individual citizens and businesses. While use of the social score is currently voluntary, costs skyrocket if one chooses not to use it (for example: renting a bike for 15 cents without a social credit score requires a security deposit of $30, putting it out of reach of the poorest).73 Nonuse could also be a limited solution for data subjects when such a choice, by itself, serves as a coarse signal of the characteristics of the data subject. Yet given that different motivations may drive nonuse, it usually cannot signal potential offenders.
Finally, in a globally interconnected world, the use of private data by one government and not another could also lead to changes in data subjects’ decisions with regard to the locality of service providers. The result could be the transfer of some activities to foreign locations, especially if the collection of data cannot be avoided because it is required for better decision-making.74 Indeed, in the wake of the Snowden revelations, some firms stopped using US cables to transfer data and began investing in their own cable infrastructure.75 This created a comparative advantage for foreign firms providing some services, thereby reducing the ability of their US competitors to collect data. Overall, this might reduce the volume of data available for governance in a particular jurisdiction.
At the same time, at least one aspect of data sharing may increase incentives of data collectors to share data beyond the price they receive for it. Sharing data with the government for the purpose of governance may indirectly benefit private collectors by reducing public pressure on them to pay data subjects for the benefits they glean from collecting and using their private data. Prominent thinkers, including Jaron Lanier, Professor Eric Posner, and Glen Weyl, argue that data collectors exploit the “work” of data subjects who supply data without pay, despite the great value of the data for its collectors. They suggest a data labor movement to force digital monopolies to compensate people for their electronic data.76 Data collectors can argue that sharing data in a way that increases overall welfare should be seen as a type of payment to data subjects.
These effects on and reactions of data collectors are also largely relevant to the analysis that follows. We refer to them only when they differ.
2. Effect on quality of data.
Data sharing can also affect the quality of the data collected for several reasons. For instance, surveillance-conscious data subjects may use various strategies of obfuscation, using tools to hide their activities77 or their identity (for example, incognito searches).78 This, in turn, may reduce the reliability of personalized laws.79
Moreover, data sharing could also create adverse selection that may interfere with the accuracy of governance-by-data. Some distortions could arise when data may be shared disproportionately by individuals who expect to benefit from personalized law or by those who do not have sufficient alternatives (for example, those who lack sufficient access to credit and must therefore rely on social scoring to establish trustworthiness). Distortions in the quality of data may also arise from tinkering and attempts to game the system in order to gain a higher score or a “better” personalized standard.80 Distortions might also arise when exogenous factors related to governance-by-data change the conduct of data subjects. Once people understand the predictors of their characteristics, their conduct might change with regard to such factors. This, in turn, reduces the effectiveness of past predictors and requires constant adjustments and additional cost.81
Clearly, the use of polluted or partial data could lead to inaccurate profiling of individuals, thus distorting the norms set by personalized laws and unjustifiably changing the level of legal duties imposed on the data subject.82 Moreover, high reliance on polluted or partial data would distort any attempt to establish accurate standards and make reliable predictions. Observe, however, that should the negative effects of inaccurate profiling on data subjects be sufficiently large (for example, as a result of the government using other, less accurate data sources), data subjects may avoid the use of data pollution tools, at least in some spheres of life.
Overall, when data collected by firms might be shared for the sake of personalized law, it may become selective, partial, and inaccurate. This may exacerbate the shortcomings for a personalized law regime arising from “data invisibles,” who do not use data-driven services at all and are presumably underrepresented in private and public data sets.
3. Effects on use of data-driven learning devices.
The effects on data collection we describe above may negatively affect both productive and dynamic efficiency. This Section explores the former, while the next focuses on the latter.
The argument here is based on the presumption that data sharing will factor into data subjects’ decisions about which devices to use. Assume that data collected by the manufacturer on the use of its product increases the technological benefits for both data subjects and future users because ongoing data collection creates a positive feedback loop. Further assume that, absent data sharing, any given data subject will prefer this particular product over competing versions. Yet data sharing might change her decisional parameters.
Consider, for instance, a car manufacturer that is collecting and analyzing data regarding one’s driving habits in order to improve the vehicle’s functionality. Should the manufacturer share this data with the government, higher legal burdens might be placed on some users of its cars. Consequently, such users might decide not to buy the “smart car,” especially if competing manufacturers do not collect such data. Note that users will not take into account positive externalities on others and will likely discount long-term positive effects from data-driven learning on themselves. Data sharing may thus have a chilling effect on the spread of otherwise efficient technologies, thereby possibly reducing benefits not only for the specific user but for all other users (given that the algorithm will have less data to learn from and that the data will be less variable due to adverse selection).
Moreover, should a sufficiently large number of consumers refrain from using the more efficient technology, this could chill the development, introduction, and assimilation of more efficient technologies that employ learning algorithms based on ongoing data collection. Indeed, in our example, while the car manufacturer will likely not commit to stop collecting data completely (given that the ongoing analysis of such data is what gives its cars their comparative advantage), it might reduce the quantity or types of data collected even if this slows advances in the functionality or safety of its cars.
4. Effects on data-driven innovation.
Perhaps most importantly, changes in data collection could also negatively affect dynamic efficiency given that data shapes the functionality and reliability of data-driven innovation. Data is the building block needed to develop new products and services based on machine learning. Machine learning enables algorithms to discover clusters and patterns in data, thereby enabling prediction based on the relationship between different parameters. Algorithms that use artificial intelligence (AI) to generate predictions require data for training. Large and diverse data sets are the foundation of any innovation in machine learning.83
Incomplete or biased data may lead to flawed predictions (garbage in, garbage out). Indeed, as concluded by a government report, “AI needs good data. If the data is incomplete or biased, AI can exacerbate problems of bias.”84 In fact, if the overall data provided to such algorithms is partial and distorted, this will reduce firms’ ability to improve predictions, including those needed for governance-by-data.
In today’s digital ecosystem, in which data is an important driver of innovation, the resultant effects on social welfare could be significant, at least in some markets.85 To cite just a few examples, in just a few years, big data has significantly increased the accuracy of biometric facial recognition;86 reduced traffic congestion;87 and increased doctors’ ability to detect malignant skin cancer and to more accurately assess side effects of medical treatments.88 For these innovations to happen, we need data, and lots of it.
Observe that, for the effects just explored to take place, the changes in conduct they bring about need not be common to all data subjects or data intermediaries. Indeed, the introduction of governance-by-data might affect market players differently. It might have no effect on the conduct of many. It might even increase the incentives of some to share data with the government. Yet governance-by-data may create a vicious circle in which changes in data collection affect the overall quality of data analysis, especially when such changes are unpredictable, undetectable, or cannot be counteracted by analytical tools. In some cases, a small change in the data may have strong effects across the whole database.
Conclusion
We live in the golden age of data collection and analysis. The number of devices that record data about our actions, our bodily responses, and the conditions in which we live is growing exponentially, as is the volume of data that is being collected, organized, synthesized, analyzed, stored, and used by private entities. The growth in the quantity and quality of data, along with advances in data-driven innovation, offer new opportunities not only to the private sector but also to the public. Data-driven innovation could strengthen law enforcement and make it more nuanced. Arguably, the nonrivalrous nature of data suggests that data could have a dual use and, therefore, that it would be efficient to extract additional value from data by putting it to use as a measure of governance. Yet as this Essay demonstrates, the use of data collected by private firms for the purpose of governance may undermine the fundamental market mechanisms of the data economy on which it relies.
The current literature implicitly endorses the proposition that a free or liberalized market for data sharing will increase social welfare. Furthermore, it treats private incentives for data collection and analysis as a given. This Essay demonstrated that these assumptions might be flawed. We showed that, once governance-by-data is introduced, the incentive of data subjects, as well as data collectors, might change. Even when the cost of sharing is nil, or close to it, concerns about risks and uncertainty and perceptions regarding governmental surveillance could reduce incentives to allow the collection and sharing of personalized data. Furthermore, when making decisions, data subjects and data collectors are likely to disregard the potential positive externalities that data sharing may create. As shown, both mandatory and voluntary data sharing might therefore lead to suboptimal markets for data collection, affecting its quantity and quality and leading to suboptimal use of data-driven learning devices and data-driven innovation.
Can the negative effects of data sharing on data-driven markets be reduced? Mandatory sharing of data (once collected) is not an efficient solution because it could potentially reduce the quantity and quality of data collected to a socially suboptimal level. Of course, the government might also mandate firms to collect certain data. But this might increase data subjects’ reluctance to share their data. Transparency and accountability in data sharing by both private firms and the government also provide a limited solution, as they address only concerns of abuse or misuse of data by governmental agents. Similarly, governmental precommitments regarding the current and future use of data for governance mandate a high level of trust in the government. Other approaches may involve placing control of data sharing in the hands of data subjects (such as Do Not Track lists), creating incentives to provide personal data through default rules and creating disincentives to provide fake data. Each has its own limitations and costs. A comprehensive analysis of these approaches is beyond the scope of this Essay.
Accordingly, to ensure that governance-by-data indeed increases welfare, the interaction among innovation, economic growth, and increased legal enforcement must be recognized and carefully analyzed. Given that data sharing affects incentive patterns, governance-by-data requires a fundamental reassessment of risk to and reward for social welfare. Put bluntly, we need to ask ourselves whether the benefits of such governance outweigh its potential negative effects on the creation of data-driven innovations, such as personalized medicine, before personalized law becomes a reality rather than legal science fiction. This Essay has taken a first step in this direction.
- 1Some of the arguments raised also apply, to some extent, to governance-by-data performed by private firms. For instance, “interactive life insurance,” which was recently announced by the life insurance company John Hancock, offers policyholders discounted premiums provided that they wear a tracking device that monitors their health. The collection of data intends to affect people’s behavior, incentivizing healthier living and, consequently, reducing the cost for the life insurance company. See Suzanne Barlyn, Strap On the Fitbit: John Hancock to Sell Only Interactive Life Insurance (Reuters, Sept 19, 2018), archived at http://perma.cc/JNJ3-EVFV. We leave this issue for future discussion.
- 2See, for example, Christopher L. Izant, Note, Equal Access to Public Communications Data for Social Media Surveillance Software, 31 Harv J L & Tech 237, 238–40 (2017); Michael D. Birnhack and Niva Elkin-Koren, The Invisible Handshake: The Reemergence of the State in the Digital Environment, 8 Va J L & Tech 6, 18–28 (2003).
- 3See, for example, Alan Z. Rozenshtein, Surveillance Intermediaries, 70 Stan L Rev 99, 112–22 (2018).
- 4See, for example, Developments in the Law: More Data, More Problems, 131 Harv L Rev 1714, 1729–36 (2018); Steven I. Friedland, Drinking from the Fire Hose: How Massive Self-Surveillance from the Internet of Things Is Changing the Face of Privacy, 119 W Va L Rev 891, 906–12 (2017); Niva Elkin-Koren and Eldar Haber, Governance by Proxy: Cyber Challenges to Civil Liberties, 82 Brooklyn L Rev 105, 131–43 (2016); Jon D. Michaels, All the President’s Spies: Private-Public Intelligence Partnerships in the War on Terror, 96 Cal L Rev 901, 929–41 (2008).
- 5For other examples of tailored norms in the driving context, see Anthony J. Casey and Anthony Niblett, The Death of Rules and Standards, 92 Ind L J 1401, 1416–17 (2017).
- 6See Omri Ben-Shahar and Ariel Porat, Personalizing Negligence Law, 91 NYU L Rev 627, 646–67 (2016); Ariel Porat and Lior Jacob Strahilevitz, Personalizing Default Rules and Disclosure with Big Data, 112 Mich L Rev 1417, 1470–76 (2014). See also Jane Bambauer, Other People’s Papers, 94 Tex L Rev 205, 242–57 (2015).
- 7See Julia Angwin, et al, Machine Bias (ProPublica, May 23, 2016), archived at http://perma.cc/MCJ5-HGU3; Big Data: A Tool for Inclusion or Exclusion? *9–11 (Federal Trade Commission, Jan 2016), archived at http://perma.cc/Q39G-NKZD; Daniel J. Solove, Nothing to Hide: The False Tradeoff between Privacy and Security 182–94 (Yale 2011). For the purposes of this Essay, we do not question the desirability of personalized law.
- 8Take, for instance, the data required to tailor speed limits to specific drivers. The data may include information about the specific circumstances (for example, weather conditions or the presence of an emergency), the general traits of the driver (for example, young, new, or repeat offender), and even her specific driving abilities and patterns (for example, her reactions to changing road conditions, eyesight, or a tendency to drive recklessly). As this example indicates, the application of personalized law requires more personal data and different types of data than currently required to enforce a speed limit. Furthermore, it may require a combination of different data sources in order to acquire the relevant information or to verify its accuracy.
- 9For examples of data capitalism and discussion of its social implications, see Shoshana Zuboff, Big Other: Surveillance Capitalism and the Prospects of an Information Civilization, 30 J Info Tech 75, 81–85 (2015).
- 10For earlier formulations of this argument, see, for example, Julie E. Cohen and William M. Martin, Intellectual Property Rights in Data, in Deanna J. Richards, Braden R. Allenby, and W. Dale Compton, eds, Information Systems and the Environment 45, 52–54 (National Academy 2001); J.H. Reichman and Pamela Samuelson, Intellectual Property Rights in Data?, 50 Vand L Rev 51, 70–72 (1997).
- 11See Part III.B.1.
- 12Determining the optimal level of data collection is beyond the scope of this Essay. We note only that the logic of data capitalism, which involves customization and prediction based on big data analysis, assumes that more data is better. See Zuboff, 30 J Info Tech at 77–79 (cited in note 9). Yet collecting too much data could lead to inefficiency if firms fail to leverage all this data into products, services, or business innovation. How Big Data and AI Are Driving Business Innovation *17 (NewVantage Partners LLC, 2018), archived at http://perma.cc/7UUQ-XZNG.
- 13Self-collection by the government has its own limitations. It could involve duplicative or costly data collection, or it could be impossible (if, for example, data results from a unique interaction that does not involve the government).
- 14Council of Economic Advisors, Big Data and Differential Pricing *8–13 (Executive Office of the President, Feb 2015), archived at http://perma.cc/FZE4-YDZ9. Data collection is also affected by the willingness of users to provide their personal information in return for digital services.
- 15McKinsey & Co estimated that data mining by firms increases operating margins by 60 percent. James Manyika, et al, Big Data: The Next Frontier for Innovation, Competition, and Productivity *2 (McKinsey Global Institute, May 2011), archived at http://perma.cc/8ACA-TPE4.
- 16Data-Driven Innovation: Big Data for Growth and Well-Being 177–207 (OECD 2015). See also Big Data and Differential Pricing at *8–15 (cited in note 14).
- 17See Zuboff, 30 J Info Tech at 78–79 (cited in note 9).
- 18We disregard here the market for data storage. See Daniel L. Rubinfeld and Michal S. Gal, Access Barriers to Big Data, 59 Ariz L Rev 339, 363–64 (2017) (discussing three types of barriers to data storage).
- 19See Katherine J. Strandburg, Monitoring, Datafication, and Consent: Legal Approaches to Privacy in the Big Data Context, in Julia Lane, et al, eds, Privacy, Big Data, and the Public Good: Frameworks for Engagement 5, 10–12 (Cambridge 2014) (discussing the three basic modes of data acquisition); Helen Nissenbaum, Deregulating Collection: Must Privacy Give Way to Use Regulation?, in Michael X. Delli Carpini, ed, Digital Media and Democratic Futures *8–9 (forthcoming 2019), archived at http://perma.cc/HP4A-T2G2 (discussing the view that data is not simply a raw resource, lying about awaiting collection but rather is “constructed or created from the signals of countless technical devices and systems”).
- 20Harry Surden, Machine Learning and Law, 89 Wash L Rev 87, 89–95 (2014).
- 21See generally Tim Wu, The Attention Merchants: The Epic Scramble to Get inside Our Heads (Knopf 2016).
- 22See Michal S. Gal and Daniel L. Rubinfeld, The Hidden Costs of Free Goods: Implications for Antitrust Enforcement, 80 Antitrust L J 521, 527 (2016) (explaining the reciprocal consumer-supplier relationship in the context of Google).
- 23See Justus Haucap and Ulrich Heimeshoff, Google, Facebook, Amazon, eBay: Is the Internet Driving Competition or Market Monopolization?, 11 J Intl Econ & Econ Pol 49, 54–60 (2014).
- 24See Rubinfeld and Gal, 59 Ariz L Rev at 346–47 (cited in note 18).
- 25Id at 369–70.
- 26Id at 352–55.
- 27Id at 355–56.
- 28Rubinfeld and Gal, 59 Ariz L Rev at 359–62 (cited in note 18).
- 29Id at 364.
- 30Id at 365.
- 31Id at 377.
- 32See Mark Lycett, Datafication: Making Sense of (Big) Data in a Complex World, 22 Eur J Info Sys 381, 381–82 (2013); Supporting Investment in Knowledge Capital, Growth and Innovation 324–25 (OECD 2013); President’s Council of Advisors on Science and Technology, Big Data and Privacy: A Technological Perspective *2 (Executive Office of the President, May 2014), archived at http://perma.cc/K49W-CWZ8.
- 33See Rubinfeld and Gal, 59 Ariz L Rev at 352–55 (cited in note 18).
- 34See Data Brokers: A Call for Transparency and Accountability *14 (Federal Trade Commission, May 2014), archived at http://perma.cc/WJ3L-9XN3 (discussing how multiple data brokers share and sell the same sources of data to consumers).
- 35See id at 23–35. See also Maurice E. Stucke and Allen P. Grunes, Big Data and Competition Policy 79 (Oxford 2016).
- 36See note 6.
- 37See Part I.B.
- 38Such standards can also create a chilling effect on some forms of innovation if, for example, they impose higher costs on smaller data collectors than on large ones. Michal S. Gal and Daniel L. Rubinfeld, Data Standardization, 94 NYU L Rev *4 (forthcoming 2019), archived at http://perma.cc/KK5R-EKVN.
- 39See Michael A. Heller and Rebecca S. Eisenberg, Can Patents Deter Innovation? The Anticommons in Biomedical Research, 280 Science 698, 698 (1998) (defining the “tragedy of the anticommons” as when “multiple owners each have a right to exclude others from a scarce resource and no one has an effective privilege of use”).
- 40At the same time, governance-by-data may create new business opportunities for some firms. ChoicePoint, Inc, for example, also offered its data services to the government, “enabl[ing] police to download comprehensive dossiers on almost any adult.” Chris Jay Hoofnagle, Big Brother’s Little Helpers: How ChoicePoint and Other Commercial Data Brokers Collect and Package Your Data for Law Enforcement, 29 NC J Intl L & Comm Reg 595, 595 (2004).
- 41Rozenshtein, 70 Stan L Rev at 145 (cited in note 3).
- 42See, for example, Monika Taddicken, The “Privacy Paradox” in the Social Web: The Impact of Privacy Concerns, Individual Characteristics, and the Perceived Social Relevance on Different Forms of Self-Disclosure, 19 J Computer-Mediated Commun 248, 265–68 (2014).
- 43See, for example, Spyros Kokolakis, Privacy Attitudes and Privacy Behaviour: A Review of Current Research on the Privacy Paradox Phenomenon, 64 Computers & Security 122, 128–30 (2017).
- 44See generally, for example, Christian Pieter Hoffmann, Christoph Lutz, and Giulia Ranzini, Privacy Cynicism: A New Approach to the Privacy Paradox, 10(4) Cyberpsychology: J Psychosocial Rsrch on Cyberspace 7 (2016).
- 45Measuring a chilling effect is complicated, as it requires proof that people would have behaved differently but for the surveillance. People’s conduct might also be affected by their (mis)perceptions of harm. See Oren Bar-Gill, Algorithmic Price Discrimination When Demand Is a Function of Both Preferences and (Mis)perceptions, 86 U Chi L Rev 217, 219–20 (2019).
- 46See Laura K. Donohue, High Technology, Consumer Privacy and U.S. National Security, 4 Am U Bus L Rev 11, 15–25 (2015); Sam Gustin, NSA Spying Scandal Could Cost U.S. Tech Giants Billions (Time, Dec 10, 2013), archived at http://perma.cc/LZ3D-P65S.
- 47Alex Marthews and Catherine Tucker, Government Surveillance and Internet Search Behavior *16–17 (unpublished manuscript, 2017), archived at http://perma.cc/2WEH-X4CJ.
- 48Jonathon W. Penney, Chilling Effects: Online Surveillance and Wikipedia Use, 31 Berkeley Tech L J 117, 145–61 (2016).
- 49Helen Nissenbaum, A Contextual Approach to Privacy Online, 140 Daedalus 32, 32 (Fall 2011). See also generally Helen Nissenbaum, Privacy in Context: Technology, Policy, and the Integrity of Social Life (Stanford 2009).
- 50The same reaction may result from a breach of trust that involves selling the data to other private firms. See, for example, Taylor Hatmaker, Users Dump AccuWeather iPhone App after Learning It Sends WB_wombat_location Data to a Third Party (TechCrunch, Aug 22, 2017), archived at http://perma.cc/BTK8-5D6V.
- 51See Margot E. Kaminski and Shane Witnov, The Conforming Effect: First Amendment Implications of Surveillance, Beyond Chilling Speech, 49 U Richmond L Rev 465, 500–01 (2015).
- 52See Larry Catá Backer, Measurement, Assessment and Reward: The Challenges of Building Institutionalized Social Credit and Rating Systems in China and in the West *5 (unpublished manuscript, Shanghai Jiaotong University, Sept 2017), archived at http://perma.cc/DEX3-NEY6.
- 53Increasing the price paid for the data will not solve this problem because the price would need to cover the expected costs resulting from the imposition of a higher legal standard, thereby eliminating deterrence.
- 54See Kaminiski and Witnov, 49 U Richmond L Rev at 472–73 (cited in note 51) (explaining how the Edward Snowden disclosure revealed such practices).
- 55See Marc Parry, Now E-textbooks Can Report Back on Students’ Reading Habits (Chronicle of Higher Education, Nov 8, 2012), archived at http://perma.cc/E8NE-V5B3.
- 56See Orla Lynskey, The Foundations of EU Data Protection Law 212–13 (Oxford 2015); Dustin Berger, Balancing Consumer Privacy with Behavioral Targeting, 27 Santa Clara Computer & High Tech L J 3, 14–15 (2011).
- 57Firstcarquote, for example, sets prices for car insurance for young drivers based on data analytics derived from Facebook posts. The app is based on findings of correlation between personality traits and levels of safe driving and on evidence that such traits are correlated with people’s habits on social media. For example, habits like writing in short, concrete sentences, using lists, or scheduling meetings at a specific time and place are correlated with traits like being conscientious and well-organized. Such traits are associated with safe driving and, therefore, merit a discounted insurance price. Conversely, the frequent use of exclamation marks and words like “always” or “never” are linked to overconfidence, which is associated with greater accident rates, leading to a higher price. Graham Ruddick, Admiral to Price Car Insurance Based on Facebook Posts (The Guardian, Nov 1, 2016), archived at http://perma.cc/5MCW-5QQG. Another example is Lodex, an Australian start-up that analyzes twelve thousand variables derived from a customer’s emails and contacts to predict their risk as borrowers. “This includes such details as how quickly you respond to an email and whether you write a title in the subject line. The idea is that when all 12,000 variables are put together and analysed, it produces a score which can help predict whether you will repay the loan.” See Clancy Yeates, How Your Social Media Account Could Help You Get a Loan (Sydney Morning Herald, Dec 30, 2017), archived at http://perma.cc/Z83N-RE8Q.
- 58See Nadezhda Purtova, Property Rights in Personal Data: A European Perspective 47–50 (Kluwer Law International 2012); Lynskey, The Foundations of EU Data Protection Law at 199 (cited in note 56).
- 59See Lynskey, Foundations of EU Data Protection Law at 200 (cited in note 56).
- 60See id at 205.
- 61The constant monitoring involved in personalized law may violate people’s reasonable expectation of privacy. In Carpenter v United States, 138 S Ct 2206 (2018), the Supreme Court held that a person does not “voluntarily ‘assume[ ] the risk’ of turning over a comprehensive dossier of his physical movements.” Carpenter, 138 S Ct at 2220. Recently, the Seventh Circuit held that smart meters, which collect energy usage data at high frequencies, may carry serious privacy implications, reasoning that “a home occupant does not assume the risk of near constant monitoring by choosing to have electricity in her home.” Naperville Smart Meter Awareness v City of Naperville, 900 F3d 521, 527 (7th Cir 2018). Personalized law may also compromise other norms, such as the principle of equality under the law. At the same time, however, the expectation that all persons will be treated equally under the law does not mean that they should be treated in the same way. Personalization may promote equality by enabling the tailoring of norms to particular nuances. See Ben-Shahar and Porat, 91 NYU L Rev at 669–74 (cited in note 6).
- 62See Daniel J. Solove, “I’ve Got Nothing to Hide” and Other Misunderstandings of Privacy, 44 San Diego L Rev 745, 766 (2007); Ruth Gavison, Privacy and the Limits of Law, 89 Yale L J 421, 425–28 (1980).
- 63Daniel J. Solove, Introduction: Privacy Self-Management and the Consent Dilemma, 126 Harv L Rev 1880, 1887 (2013).
- 64Recently, in a case involving cell site location data, the Supreme Court has rejected the third-party doctrine, which presumes no reasonable expectation in privacy for information that was voluntarily exposed to third parties. The Court held that this doctrine does not apply to “the exhaustive chronicle of location information casually collected by wireless carriers today.” Carpenter, 138 S Ct at 2219.
- 65For a dystopian exposition of this risk, see generally Dave Eggers, The Circle (Vintage 2013).
- 66See Yoan Hermstrüwer and Stephan Dickert, Sharing is Daring: An Experiment on Consent, Chilling Effects and a Salient Privacy Nudge, 51 Intl Rev L & Econ 38, 45–46 (2017).
- 67Phillip Rogaway, The Moral Character of Cryptographic Work *25–30 (2015), archived at http://perma.cc/5DDH-HW8N.
- 68See Rozenshtein, 70 Stan L Rev at 115–17 (cited in note 3) (suggesting that industry giants like Google, Microsoft, and Yahoo have sufficient corporate power to check, rather than to facilitate, government surveillance).
- 69See Katie Benner and Paul Mozur, Apple Sees Value in Its Stand to Protect Security (NY Times, Feb 20, 2016), archived at http://perma.cc/SUH2-ZA4Y; Rozenshtein, 70 Stan L Rev at 130–32 (cited in note 3).
- 70See Rozenshtein, 70 Stan L Rev at 127–29 (cited in note 3).
- 71For instance, Trustbond, a joint venture between Suncorp and the Spanish start-up Traity, allows renters to substitute their rental bond for a lower nonrefundable fee, provided that they grant access to their social media accounts to help determine their level of risk. Yeates, How Your Social Media Account Could Help You Get a Loan (cited in note 57).
- 72See Planning Outline for the Construction of a Social Credit System (China
Copyright & Media, June 14, 2014) (Rogier Creemers, trans), archived at http://perma.cc/VBF6-JBKV. - 73See Mara Hvistendal, Inside China’s Vast New Experiment in Social Ranking (Wired, Dec 14, 2017), archived at http://perma.cc/9XS6-EKMB.
- 74Moreover, numerous foreign jurisdictions have accelerated data localization initiatives that restrict the storage, analysis, and transfer of digital information outside national borders. Donohue, 4 Am U Bus L Rev at 15–18, 35–36 (cited in note 46).
- 75See id at 16. See also Rozenshtein, 70 Stan L Rev at 118 (cited in note 3).
- 76Jaron Lanier, Who Owns the Future? 108–09, 245–46 (Simon & Schuster 2014); Eric A. Posner and E. Glen Weyl, Radical Markets: Uprooting Capitalism and Democracy for a Just Society 239–49 (Princeton 2018).
- 77For example, TrackMeNot issues randomized queries. Daniel C. Howe and Helen Nissenbaum, TrackMeNot (NYU Department of Computer Science), archived at http://perma.cc/HEN7-SP4Y. AdNauseum automatically clicks on all ads to obscure data subjects’ interests. AdNauseum, archived at http://perma.cc/2BQ6-HNC8. See also Helen Nissenbaum, From Preemption to Circumvention: If Technology Regulates, Why Do We Need Regulation (and Vice Versa)?, 26 Berkeley Tech L J 1367, 1371 (2011).
- 78See Amul Kalia, Here’s How to Protect Your Privacy from Your Internet Service Provider (Electronic Frontier Foundation, Apr 3, 2017), archived at http://perma.cc/R5RM-4LNT.
- 79In reaction to such tools, firms develop ways to follow one’s digital fingerprint. See, for example, Brendan van Alsenoy, et al, From Social Media Service to Advertising Network: A Critical Analysis of Facebook’s Revised Policies and Terms *89–90 (Belgian Privacy Commission Working Paper, Aug 25, 2015), archived at http://perma.cc/VZ9P-GKGV.
- 80Gaming might become more difficult as the system becomes more robust in terms of the volume, veracity, and velocity of the data on which it relies. See Porat and Strahilevitz, 112 Mich L Rev at 1454–56 (cited in note 6).
- 81See, for example, David Lazer, et al, The Parable of Google Flu: Traps in Big Data Analysis, 343 Science 1203, 1204 (2014) (discussing Google Flu Trends’s miscalculation of flu occurrences due to reliance on a stagnant search algorithm).
- 82See Lynskey, Foundations of EU Data Protection Law at 199 (cited in note 56).
- 83Testimony before the Subcommittees on Communications and Technology and Digital Commerce and Consumer Protection of the House Committee on Energy and Commerce, Algorithms: How Companies’ Decisions about Data and Content Impact Consumers, 115th Cong, 2d Sess 3 (Nov 29, 2017), archived at http://perma.cc/6V92-B85M.
- 84National Science and Technology Council Committee on Technology, Preparing for the Future of Artificial Intelligence *30 (Executive Office of the President, Oct 2016), archived at http://perma.cc/9C3A-FUQZ.
- 85Of course, data could also drive bad choices. See generally Cathy O’Neil, Weapons of Math Destruction: How Big Data Increases Inequality and Threatens Democracy (Crown 2016).
- 86For instance, Joy Buolamwini showed that facial recognition systems are more likely to err in identifying images of darker skinned women, whereas if the person in the photo is a white man, it is 99 percent accurate. Researchers argue that this is the result of the data that was used to train these systems consisting of more white men than black women. See Joy Adowaa Buolamwini, Gender Shades: Intersectional Phenotypic and Demographic Evaluation of Face Datasets and Gender Classifiers *3 (master’s thesis, MIT, Dec 2017), archived at http://perma.cc/XP49-WF3Q.
- 87See Carl-Stefan Neumann, Big Data versus Big Congestion: Using Information to Improve Transport (McKinsey & Co, July 2015), archived at http://perma.cc/RAC7-756Y.
- 88See Abhishek Bhattacharya, et al, Precision Diagnosis of Melanoma and Other Skin Lesions from Digital Images (AMIA Joint Summits on Translational Science, July 26, 2017), archived at http://perma.cc/PQQ2-9GTM.