AI Judgment Rule(s)
This Essay explores whether the use of AI to enhance decision-making brings about radical change in legal doctrine or, by contrast, is just another new tool. It focuses on decision-making by board members. This provides an especially relevant example because corporate law has laid out explicit expectations for how board members must go about decision-making.
Whatever else it produces, an organization is a factory that manufactures judgments and decisions. Daniel Kahneman, Thinking, Fast and Slow 418 (2011)
Introduction
Decision-making is the cornerstone of corporate life. Daily, employees at various hierarchical levels, officers, directors, and board members make decisions, most of them under uncertainty, some under risk. Decisions can be highly standardized, such as those that occur when a worker repeats one task at an assembly line. Others involve more discretion—for instance, credit underwriting decisions. Much of what employees use to prepare these credit decisions is standardized information, such as a credit score. However, in individual cases, employees can depart from what a score suggests if they feel they have a better understanding of a specific borrower’s profile. The higher you move up on the corporation’s hierarchical ladder, the more you tend to have room for discretionary decision-making. The head of compliance has considerable leeway when she organizes the work of employees who make credit-underwriting decisions. Moving yet further up, directors enjoy the highest latitude for decision-making. They structure the entire corporation’s workflow and make core business strategy decisions.
Decision-making under uncertainty is of keen interest to a variety of scholarly disciplines. Some are concerned with developing optimal, utility-maximizing strategies. They work with assumptions such as stable preferences, observer-independent measurability of probabilities, and known outcome values of choice options. Others are more interested in understanding how decisions are actually made under varying circumstances. One approach investigates which areas of the brain are activated in different decision-making situations. Others, in the vein of traditional methods of psychological research, use laboratory experiments. These scholars stress that perceptions of utility are subjective and context dependent. Their emphasis is on showing how heuristics and biases distort the path toward optimal decision outcomes. Yet others underscore that heuristics are anything but unhelpful. They view them as an efficient evolutionary tool to cope with situations where decisions must be made with limited information and time. Managerial decision-making is a paradigm example of what these authors have in mind. Similar thoughts have been advanced by scholars who point out that humans pursue satisficing, rather than optimizing strategies.
The law has its own theories on decision-making. Many are explicitly normative. They specify how a decision should be made, what the decision outcome must be, or—more often—what the outcome may not be. In addition to explicit normative theories, the law rests on implicit theories of decision-making. Take a legal rule that assumes decision-making follows optimal choice patterns. It will look very different than a rule that presumes heuristics and biases guide human decisions. The first type of legal rule will usually hold actors to a high standard of optimal decision-making procedures and outcomes. By contrast, the second type of legal rule might make room for known flaws in human decision-making.
So far, one unifying implicit assumption has underlain legal rules: they regulate human behavior. With the rise of artificial intelligence (AI) to support and augment human decision-making, this assumption does not necessarily hold. This is evident for propositions to conceptualize AI as an autonomous “algorithmic actant” that will, in the future, be the addressee of legal rules. Even without subscribing to this bold view, AI is about to change human decision-making in various, more or less subtle ways.
AI’s impact on decision-making will show across the economy. The worker at the assembly line will have a robot colleague, tirelessly performing tasks once done by a human. The employee’s decisions on credit underwriting will be preformatted by an AI that far surpasses human abilities to process relevant data on credit applicants. The head of compliance will use an AI to predict the probability of infringements, investigate past rule violations, and measure and surveil employees’ work-related behavior. Directors will benchmark their decisions against the prediction of the corporation’s AI. Many will use it for new business strategies, have it assess risks and rewards, and rely on its output when making a tough judgment call.
This essay explores whether the use of AI to enhance decision-making brings about radical change in legal doctrine or, by contrast, is just another new tool. It focuses on decision-making by board members. This provides an especially relevant example because corporate law has laid out explicit expectations for how board members must go about decision-making. By and large, the law trusts corporate boards to make disinterested, careful, and well-informed decisions. Directors are encouraged to delegate many decisions to officers and employees. They can, and sometimes even must, seek expert support when preparing core board decisions. At the same time, most legal orders rely on implicit theories of decision. Rather than requiring optimal decisions, the business judgment rule incorporates managerial intuition and gut instincts. Additionally, corporate law requires board members to own the decisions they make. Running the corporation is not a task board members can outsource via abdicating authority. If they delegate decisions, a duty to structure and supervise remains with the board. Their very own judgment, be it carefully deliberated or driven by intuition, is what the law expects.
What will owning a decision look like if an AI comes up with business strategies, evaluates competing options, and, based on countless comparable cases, suggests the best way forward? What if the AI is a black box? Will the law’s trust in board members allow them to delegate to an AI that they do not understand? Is the AI just another expert preparing board decision-making, or does an AI have better intuition than humans do?
This Essay engages with these questions. It submits that we must rethink the law’s implicit assumption of humans making the decisions that corporate law regulates. If there is movement in implicit assumptions about how people make decisions, legal rules need review. This does not automatically lead to a call for fundamental change. Long-standing implicit assumptions might still provide an adequate description of reality. But we need a careful investigation and debate to confidently say so.
I. Board Decision-Making in the Age of AI
Predictions are at the core of most management decisions. Take the investment in the development of a new product. It is economically viable only if the board anticipates a market for it. Alternatively, imagine a board that prepares the takeover of a target company. It envisions that integrating this company will be successful. Likewise, consider the decision to initiate an IPO. It depends on a prognosis of how the market will evaluate the company’s shares. For yet another example, picture a board that seeks a new board member. Again, this involves an evaluation of that person’s personality, competencies, and overall fit, compared with those of other potential candidates. Analyses of probabilities along those lines have in the past been based on traditional statistical methods.
By way of illustration, take senior management contemplating the risks and rewards of an IPO. There is the risk of substantial reputational damage if the process is initiated but later aborted because the market does not value the company in line with management's assessment. Treading carefully and using all available tools of analysis is core. Among these, the prediction of the future market price is one key factor. A standard method to proceed is to investigate, inter alia, the (assumed) interdependency between (i) return on equity and (ii) price-to-book ratios. For listed companies, both are known. For the potential IPO candidate, the board will assess variable (i) based on the business plan and input of senior management. Variable (ii) is unknown until market prices become visible. However, evaluating comparable listed companies allows one to make a prediction on the future price-to-book ratio of the IPO candidate to inform board members about the expected market price that a public offering might yield. To this end, (i) is used as the independent variable and (ii) as the dependent variable of a single linear regression.
Imagine asking an AI to make that prediction. In contrast to classical statistical methods, AI requires neither prior knowledge as to the dependence of the output on the input nor which input variables are of interest. (Necessary assumptions are that the data collected is vast enough to contain all the information required for the AI to be able to identify the variables of importance and for these to exist within the data set). Additionally, the AI does not assume any specific function, e.g., linear, nor a specific set of input variables. Some of what the AI identifies will involve variables that make immediate sense to human analysts—for instance, the company’s credit rating or its brand value. The same is true for softer variables such as board diversity and sustainability efforts.
However, the broader the database, the more variables drive the AI’s prediction. Possibly, the good looks of a CEO push IPO success. Maybe the ugliness of the CEO is an even better predictor. Maybe the month of the year, the hours of sunshine each day, or the number of coffee shops around the stock exchange have predictive force. If the board uses a black-box AI, it will be unknown which variables were important factors in influencing the decision.
At the same time, machine-learning AI establishes correlations only. Many will be spurious. Some will explain past events but, if circumstances change, will lose their predictive power. This puts board members who engage with the AI’s forecast in a difficult spot. In many instances, the AI will make far better predictions than traditional statistical models. Not using it would risk neglecting a powerful tool that, arguably, will become market standard. However, biased or poor-quality training data, a novel development that the AI has not (yet) integrated, or an unrecognized flaw of the model, such as over- or underfitting, can produce wrong predictions. Using an explainable AI provides help only if its explanation unearths the mistake, rather than leads to humans deepening it. Disturbingly, various studies suggest that human-AI collaboration can lead to worse decisions than relying on AI alone. Along a similar vein, transparency and explanations have been shown to backfire rather than help.
II. Decision-Making Under Uncertainty: Collecting Relevant Information
Decisions under uncertainty profit from collecting “relevant information[,] . . . reflection, and review.” Corporate law requires distinct, context-dependent efforts towards that goal.
In some situations, the law asks decision-makers to collect all available information and then carefully reflect upon and painstakingly review the ultimate decision. Risk management and compliance fall into that category. A judge will not entertain a bank manager’s claim that the effort to measure precisely which cybersecurity risks the institution faces was too costly, and thus, the manager disregarded those risks. Neither will he accept the argument that a legal rule could be read in many ways and the corporation chose to rely on the one most beneficial to the pursued objective, even if no court had ever chosen that interpretation. The bank manager must implement a sound risk management department; the corporation must hire a lawyer. With professional help of that type, board members must assess and weigh all available options and try to reduce uncertainty as meticulously and exhaustively as feasible before reaching a decision.
For business judgments, the law has different expectations regarding how board members should deal with uncertainty. Faced with shareholders’ preference for risk-seeking boards, the business judgment rule, by and large, embraces uncertainty. Under U.S. law, directors profit from a presumption that they did not violate the duty of care. A court will uphold the director’s decision if it was made in good faith, in the absence of a conflict of interest, and with the care a reasonably prudent person would use. While this includes deciding on an informed basis, a full-scale collection of any available information and extensive reflection and review do not form part of the reasonably prudent person standard. Instead, the law is open to directors deciding based on their experience and intuition. It accepts that there is often little time, and that there will be a variety of known or unknown unknowns.
Similarly, under German law, a director does not violate his duty of care if he acted in the best interest of the company and could reasonably believe that this was done on an informed basis. Contrary to U.S. law, the German rule does not shift the burden of proof that is placed on directors. Furthermore, there is no direct equivalent to the U.S. standard of a reasonably prudent person. Instead, courts have a very high standard as to the available information board members must collect, despite scholarly criticism that this risks undermining the German business judgment rule.
The collection of relevant information as such (exhaustive or of reasonable scale) is an integral part of both business judgments and other board decisions. Technical support tools have been a part of this process, encompassing pocket calculators, Excel spreadsheets, or more sophisticated machines. The same goes for human support: boards regularly hear from employees, officers, or outside experts to inform their decisions.
Corporate law has been confident that board members can affirm ownership of a decision that technical support tools or humans have contributed to. As far as technical support tools are concerned, the law has unquestioningly assumed that the board owns its decision. There will be duty of care obligations to ensure the support tool is at market standard and appropriately used, but ownership for the ultimate decision resides with the board members. For humans that inform board decision-making, U.S. and German law both expect more engagement.
DGCL § 141(e) fully protects members of the board of directors that rely “upon . . . information, opinions, reports or statements presented to the corporation by any of the corporation’s officers or employees, or committees[,] . . . or by any other person.” This must be done in good faith. For outside experts (“any other person”), the rule’s test adds extra prongs. Their input must stem from “any other person as to matters the member reasonably believes are within such other person’s professional or expert competence.” Additionally, such a person must have “been selected with reasonable care by or on behalf of the corporation.” For both issues, the standard of review is strict, and the business judgment rule is not available.
German law goes one step further. It requires board members to explicitly affirm ownership of a decision that it takes with outside help. German law contains no statutory rule governing the integration of external input into board decision-making. However, when evaluating information by an outside expert, a landmark court decision has added an extra test prong to the board’s duty of care.
The watershed case involved an executive board deciding on a capital increase. One of the supervisory board members had suggested a specific strategy that the courts later declared illegal. This supervisory board member was the partner of a law firm that was mandated to work on structuring the capital increase. The members of the executive board claimed they had, in good faith, relied on the law firm partner on their supervisory board, along with the work done by his firm. The German court did not accept this defense. It stressed that the board’s own duty of care included investigating the legal ramifications of its decisions. The risk of misunderstanding the law, so the court held, was to be borne by the board, even if they were not legal experts. The court highlighted an obligation for individual board members to make sure the expert opinion was “plausible.” Board members were to double-check whether what the expert had proposed was in line with their own market knowledge, experience, and, possibly, intuition.
III. The Implicit Assumption: Human Decision-Makers
Corporate law rules governing board decisions and ownership of information furnished by non-board members all have human decision-makers in mind. DCGL’s § 141(e) speaks of directors relying on information that other human persons present to the board. The “plausibility check” under German law was developed to incentivize board members to engage in critical discussion with experts. An implicit assumption of both jurisdictions is that board members can cognitively follow when experts present their findings or, alternatively, ask for an explanation. Even if outside experts have been trained in another discipline than the board members, employ different methods, and are used to an unfamiliar style of reasoning, the law assumes that a meaningful dialogue between experts and board members is possible. German law explicitly asks board members to challenge those who furnish information and expects them to conduct their own plausibility check.
Does the implicit assumption that human cognition and interaction are what drive board decision-making translate seamlessly to integrating an AI?
One way of looking at it is to conceptualize AI as a purely technical support tool. Consider the IPO example from above: like the computer program that delivered the linear regression, the AI predicts how the market will react to the IPO. It is still the board, one might claim, that takes the decision to initiate the IPO process, even if it more or less blindly follows what the AI suggests.
Another option is to analogize an AI to an “officer, employee” or “any other person.” Similar to an expert that informs the board, the AI gives the board input to ponder. Again, one argument might run, the board, not the AI, makes the final decision, even if the board, as a rule, follows the AI’s recommendation.
Yet another approach is to stress dissimilarities between AI, traditional technical support tools, and human experts. The remaining part of this Essay goes down that route. It submits that reflection and review are core elements of how corporate law has conceptualized board decision-making. It moves on to suggest that with the increasing complexity of AI, especially of the black-box variety, processing its input by humans looks fundamentally different than dealing with traditional support tools or experts. This leaves us with the question of what ownership of AI-generated support could look like.
III. Decision-Making Under Uncertainty: “[R]eflection and Review”
We have seen above that corporate law trusts board members to collect information to prepare their decisions. Duties of care concern the choice of information; under U.S. law, they vary depending on whether an in-house or an outside expert has contributed. The implicit assumption that board members will cognitively engage with the input they receive is evident from the wording of DGCL § 141 (e). The rule addresses protection from liability for board members in the context of “the performance of such member’s duties.” And under DGCL § 141(a), board members’ prime duty is to manage the “business and affairs” of the corporation—a responsibility echoed in German Aktiengesetz § 76 (1). German courts spell this out even more clearly when they ask board members to perform a plausibility check on information they receive.
Engaging with input that was received from an AI looks different if compared to either a traditional technical support tool or a human expert. There is no conversation, even if large language models might make you think so. There is very little understanding of how the AI produces its results. With a black-box AI, board members do not get information on relevant variables or their weights. Depending on the data and model, it will be hard or impossible to estimate the probability that the AI’s prediction is biased, not suitable enough to the corporation’s situation, or altogether wrong.
Anyway, one counterargument might run, machines do not always function properly, and human experts can err. What is so different about an AI? The difference, I’d like to suggest, is the way in which an AI “malfunctions” and “errs.”
Corporate law’s received approach to cope with risks of malfunctioning technical support tools or erring human experts is based on the expectations of the duty of care: quality requirements (of technical tools) and critical dialogue (with human experts). The former will cure some flaws of AI support and is what regulation, such as the EU AI Act, has in mind. Arguably, critical dialogue has little equivalent because an AI “reasons” differently than a human expert. We have not yet been successful in encoding microtheories or frameworks to represent knowledge or understanding. Humans, when faced with a prediction task, tend to formulate a hypothesis against the background of their real-world understanding. Possibly, they collect data to test it. An AI approaches this task differently, namely as a challenge of inductive inferences from data. Even if researchers can employ an AI to generate a variety of causal hypotheses, it still performs “a theory-blind, data-driven search of predictors.”
For a board member, this makes evaluating the AI input a novel challenge, different from dealing with technical support tools or human experts. She might have a gut feeling that the data could be biased or inadequately reflect the situation of her corporation. However, she might not be able to establish whether her intuition would account for a change in the AI’s prediction. She would be able to ask a human expert that question and engage in a dialogue. By contrast, a black-box AI might not produce an answer; a flaw in the model or a bias in the training data might have been overlooked. An explainable AI will give her variables and their weights, but this does not help if the variable looks convincing while the mistake lurks elsewhere.
A deeper reason for this conundrum is the difference between how a human and an AI “explain.” When confronted with a human expert, the board member would ask for a causal explanation. She would be interested to hear why certain variables—for instance, the looks of the CEO—drive the outcome and, against that background, make her decision. For an AI to provide a causal analysis of multivariate data, we would need to model and infer causality from data. In some cases, the attempts to develop causal AI can offer a useful work-around. Even if this is available, the AI mostly gives counterfactual clues, as it were, but does not provide the theoretical, conceptual explanation a human expert would expect to hear. Without a causal explanation, the human board member is left with the uneasy feeling that she might run tests to check counterfactuals but does not understand the broader picture.
IV. What’s Next: Owning Decisions in the Age of AI
Encoding knowledge, building hypotheses-based explanations, and causality are just three examples to show how an AI “reasons” and “explains” differently than a human person. They suggest that building a board decision on an AI’s prediction resembles neither the use of a traditional technical support tool nor a dialogue with a human expert.
This leaves us with the question of what owning a decision implies when it rests on the AI’s prediction. Once we have established an (even preliminary) understanding of what some form of cognitive cooperation might look like, the next steps concern zooming in on legal regulation. Elsewhere, I have suggested that duties of care and judicial scrutiny vary along two axes: the extent to which a board’s decision is a business judgment and the extent to which it delegates core parts to the AI.
Sometimes, a prediction will simply be an inspiration for the board to pursue a new business idea. The board will not be overly interested in understanding a theory that could explain a prediction, for instance on a new antibiotic. It would understand the probability that the AI is right or wrong as a known unknown, price it, and move on. There is no doubt that the board would own this decision. This is different if the AI’s prediction is an integral part of the board’s decision, for instance when it suggests a new member to fill a vacant board seat. Does the board own the decision if it just goes along with the person the AI suggests? What if the board members have an uneasy feeling about the candidate but are convinced that the AI will make the better judgment?
At the other end of the spectrum, we find the much-discussed issue of algorithmic discrimination. May a board claim that it did not discriminate because it outsourced the decision to an AI? What if this is a black-box AI? What if the AI only supports a compliance investigation?
Figure 1
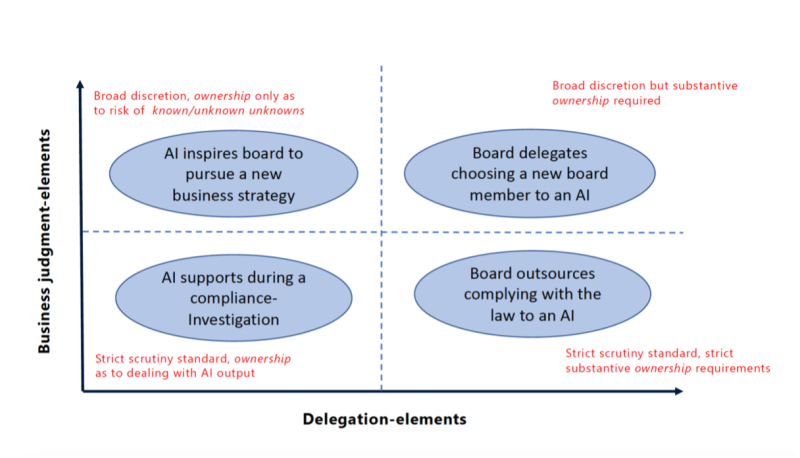
AI puts corporate law’s implicit assumptions about board decision-making to the test. This Essay has suggested that ownership of a board decision must be reviewed if cognitive reflection, critical dialogue, and review look different from what human decision-makers are used to. For corporate law, this implies the need to rethink implicit assumptions about how board members make decisions. Enhanced duties for boards that consult outside experts do not adequately capture what is different about an AI that augments board decisions, especially one of the black-box variety. At the same time, tightly regulating the use of AI would deprive boards of a powerful tool. Arguably, the law first needs another implicit theory on decision-making to then review and adapt its normative framework.
* * *
Katja is a law professor at Goethe-University, Frankfurt; member of Leibniz Institute SAFE; affiliated professor at SciencesPo, Paris; and visiting faculty at Fordham Law School.